This course will give an introduction into the topic of Machine Learning Operations, also known as MLOps. It will be based on the open source material from the Technical University of Denmark and cover the machine learning lifecycle from the design to the model development and until operations.
Einschreibeschlüssel: mlops23
- Викладач: Feurer Matthias
Key: dcqd2023 (Einschreibeschlüssel)
General Information
The class will take place as a block seminar from September 18 to 21 and September 25-28 (9am-12pm; 2pm-5pm) and will be taught online over Zoom in English.
To sign up for the class just sign up for this moodle class. The official registration will then be done with the exam registration. At the end of the seminar, students will be required to take an oral exam.
If you have any questions, please contact anna-carolina.haensch@stat.uni-muenchen.de
Rough course Outline: (more detailled Syllabus in week before seminar starts)
Week 1 - Data Collection (Prof. Sakshaug)
Short Course Description
The social survey is a research tool of fundamental importance across a range of disciplines and is widely used in applied research and as evidence to inform policy making. This course considers the process of conducting a survey, with an emphasis on practical aspects of survey data collection, as well as factors that influence the quality of survey data. The course will also cover key statistical concepts and procedures in sample design and estimation.Morning session (9-12) and Afternoon session (2-5):
Live lectures, discussion, and readings
Week 2 - Questionnaire Design (Prof. Kreuter)
Short Course Description
This course introduces students to the stages of questionnaire development. The course reviews the scientific literature on questionnaire construction, the experimental literature on question effects, and the psychological literature on information processing. It also discusses the relationship between mode of administration and questionnaire design.
Morning session (9-12):
Self-study, including lecture videos and multiple readings per day (will be made accessible via moodle)
Afternoon session (2-5):
Exercises,
quizzes, discussion sessions building on the material of the morning
session. Students are required to submit questions on the readings.
- Викладач: Beck Jacob
- Викладач: Fuchs Carla
- Викладач: Haensch Anna-Carolina
- Викладач: Kreuter Frauke
- Викладач: Sakshaug Joseph
Entropy is defined as a measurable physical property that is most commonly associated with a state of disorder, randomness, or uncertainty. It is strongly connected with probability distributions and the principle of maximum entropy can be very useful in statistical inference, in particular in Bayes statistics. In this course we will introduce the concept of entropy in the context of information theory as well as apply the concepts on real data sets.
Syllabus
- Introduction and Preview
- Entropy, Relative Entropy, and Mutual Information
- Asymptotic Equipartition Property
- Entropy Rates of a Stochastic Process
- Differential Entropy
- Information Theory and Statistics
Schedule:
day/time | location | instructor | start date | |
---|---|---|---|---|
Lecture/Tutorial | Mon, 12:00-14:00 | Geschw.-Scholl-Pl. 1 (B)/ B (106) | Farsani | 17.04.23 |
Lecture/Tutorial | Thurs, 16:00-20:00 | Geschw.-Scholl-Pl. 1 (M) / M 010 | Farsani | 20.04.23 |
Information Theory and Entropy for Master Statistics and Data Science, Master Data Science, Master Statistik, Master Biostatistik, Master Statistik WiSo.
Enrolment Key: Shannon23
- Викладач: Aminifarsani Zahra
- Викладач: Schmid Volker
This course will discuss essential research techniques in statistics and data science, also preparing students for successfully participating in seminars and writing a thesis. (5 sessions, Friday afternoon)
The course is part of the Bachelor’s programmes in Statistics and Data Science (150 major or 60 ECTS minors: `Methoden und Techniken des wissenschaftlichen Arbeitens’, P16.1, and WP 12.1 / WP 13.1, respectively ). It is also open to all other Bachelor’s students (4th semester or higher) and all Master’s students.
Einschreibeschlüssel: Zitieren
- Викладач: Augustin Thomas
- Викладач: Kreiß Dominik
Beginn der Ringvorlesung ist am 4. Mai; ein Termin wird sich jeweils auf knapp zwei Zeitstunden erstrecken (16.05 bis 18.00 Uhr).
Die Ringvorlesung gibt einen Überblick über verschiedene Themengebiete der Statistik, die in den spezifischen Modulen nicht entsprechend behandelt werden können.
Geplant sind
* verschiedene Gastvorträge aus der Berufspraxis
* einige Vorträge zur Geschichte der Statistik und der Künstlichen Intelligenz inklusive ihrer Grundlagen
* Überblicksvorträge über Teilgebiete der aktuellen statistischen Forschung und damit über die verschiedenen Spezialisierungen im Masterstudium
* ein Themenblock zu Kommunikation statistischer Ergebnisse, Datenjournalismus und Open Science
Einschreibeschlüssel: Ringvorlesung
- Викладач: Augustin Thomas
- Викладач: Kreiß Dominik
- Викладач: Schmid Volker
This is an overview page for all courses in official statistics offered this summer semester.
Enrollment Key: EMOS
- Викладач: Augustin Thomas
- Викладач: Herklotz Markus
- Викладач: Kreiß Dominik
- Викладач: Radermacher Walter
enrolment key: why?
- Викладач: Rodemann Julian
- Викладач: Schomaker Michael
Person: Dr. Cornelia Oberhauser
SAS-Kurs als 5-tägiger Blockkurs in den Semesterferien
Termine:
Tag | Uhrzeit | Raum | |
---|---|---|---|
Mo 18.09.2023 | Vorlesung | 9:15 - ca. 12:15 | online über Zoom |
Übung | 13:15 - 17:00 | online über Zoom | |
Di 19.09.2023 | Vorlesung | 9:15 - ca. 12:15 | online über Zoom |
Übung | 13:15 - 17:00 | online über Zoom | |
Do 21.09.2023 | Vorlesung | 9:15 - ca. 12:15 | online über Zoom |
Übung | 13:15 - 17:00 | online über Zoom | |
Mo 25.09.2023 | Vorlesung | 9:15 - ca. 12:15 | online über Zoom |
Übung | 13:15 - 17:00 | online über Zoom | |
Di 26.09.2023 | Vorlesung | 9:15 - ca. 12:15 | online über Zoom |
Übung | 13:15 - 17:00 | online über Zoom |
Gastschlüssel
- Der Gastschlüssel lautet: "saskurs2023"
- Викладач: Oberhauser Cornelia
Key: reg-cor-dat
Course kick-off 20.04. with an introductory in-person lecture.
Lecture Q&A / Exercise class (Scheipl/Sapargali) |
Thursday |
14:15-15:45 |
Schellingstr. 3 (S) - S 001 |
Format:
Inverted classroom: You are expected to come prepared for both the Q&As and exercise classes -- you've watched the lecture videos,
you've reviewed the slides/exercise sheets, you've asked ChatGPT to
explain parts you found confusing, you've done the self-assessment
quizzes, and you've posted questions you would like to see
addressed during the session in the Moodle Forum.
- Викладач: Sapargali Nurzhan
- Викладач: Scheipl Fabian
- Der Einschreibeschlüssel ist StoSta23
- Vorlesungen aus vorigen Semestern auch als aufgezeichnete Videos bei LMUcast (s.u.) verfügbar. Multiple Choice Quizzes zur Selbstkontrolle auf moodle.
- Für die Übungen gibt es Musterlösungen als PDF und Fragestunden zur Übung in vorraussichtlich 2 Gruppen Mittwochs und Donnerstags.
Termine
Vorlesung:
Di 12-14 @ S 002 (Schellingstr. 3) & Do 12-14 @ S 003 (Schellingstr. 3)
Übung/Fragestunde:
Mi 12-14 (E 004 HGB), Mi 14-16 (E 004 HGB), Do 14-16 (E 004 HGB)
- Викладач: Buttazzo Sergio
- Викладач: Sapargali Nurzhan
- Викладач: Scheipl Fabian
- Викладач: Schuhegger Aïsha
- Викладач: Schuhegger Aïsha
- Викладач: Windmann Michael
Schedule:
date/time | location | instructor | |
---|---|---|---|
Kick-off meeting | April 26, 18:00 - 19:00 | Old Library, Room 245, Ludwigstr. 33 | Wilhelm |
Presentations | at end of semester (date tbd) | tbd | Wilhelm |
- Викладач: Wilhelm Daniel
Schedule:
day/time | location | instructor | start date | |
---|---|---|---|---|
Lecture/Tutorial | Mon, 16:15-18:00 | Schellingstr. 3 (S) / S 006 | Wilhelm | 17.10.22 |
Lecture/Tutorial | Wed, 10:15-12:00 | Geschw.-Scholl-Pl. 1 (M) / M 010 | Wilhelm | 19.10.22 |
Enrolment key: ml23
- Викладач: Wilhelm Daniel
Syllabus:
- Overview
- Fundamentals and Properties of Stochastic Processes
- Univariate ARIMA-Processes
- Estimation and Forecasting of ARIMA-Models
- Univariate GARCH-Models + Extensions
- Selected aspects: Long Memory und Fractional Differencing, Threshold-Models
Intended audience: Advanced bachelor and master students of statistics, mathematics, computer science (Informatik), economics and business administration.
Prerequisites: Solid mathematical foundations (analysis and linear algebra), basic knowledge in econometrics (econometrics 1) or statistics (linear models).
Record of Achievement:
- Викладач: Mao Dennis
Einschreibeschlüssel: Infstat22023!
- Викладач: Anders Jan
- Викладач: Buttazzo Sergio
- Викладач: De Nicola Giacomo
- Викладач: Kauermann Göran
Dieser Kurs ist eine Wiederholung ausschließlich für die alte Prüfungsordnung 2010 für den Bachelor Statistik. Insofern der Kurs Wahrscheinlichkeitstheorie und Inferenz I noch nicht bestanden worden ist, empfehlen wir dringend auf die neue Veranstaltung zur Inferenz auszuweichen.
Hierfür wird direkt zu Beginn des Semesters Material in Form eines Skriptes, Vorlesungsfolien und Übungsblätter mit deren Lösungsansätzen online gestellt und in einer monatlichen Fragestunde besteht die Möglichkeit auf einzelne Dinge einzugehen und Fragen zu stellen.
Außerdem wird es in der Woche vor der Klausur ausführlicher Fragen zu stellen und Dinge zu wiederholen.
Diesen Kurs zu belegen, bedeutet also ein hohes Maß an Eigenverantwortung.
Ich empfehle dringendst zu der Einführungsstunde am Donnerstag, den 20.04. um 10 - 12 Uhr zu kommen, nachdem da das Format von dem Kurs erklärt wird.
Der Einschreibeschlüssel ist: Wiederholungskurs
- Викладач: Kobl Michael
- Викладач: Kreiß Dominik
Schedule
Time | Lecturer | Begin | |
---|---|---|---|
Exercise course (Group 1) |
Tuesday, 10:15 - 11:45 |
Sapargali, Garces Arias |
25.04.2023 |
Lecture |
Tuesday, 12:15 - 13:45 |
Prof. Dr. Heumann |
18.04.2023 |
Exercise course (Group 2) |
Wednesday, 08:15 - 09:45 |
Sapargali, Garces Arias |
26.04.2023 |
Tutorium |
Friday, 08:15 - 09:45 |
Eleftheria | 21.04.2023 |
Lecture |
Friday, 10:15 - 11:45 |
Prof. Dr. Heumann |
21.04.2023 |
Enrollment Key
- The enrollment key is "stat_inf_s23"
- Викладач: Garces Arias Esteban
- Викладач: Heumann Christian
- Викладач: Papavasiliou Eleftheria
- Викладач: Sapargali Nurzhan
- This lecture covers the basics of Bayesian statistics and its practical applications
- The lecture is held in English. It will be held online too.
- The course may be taken by students with a minor in "Statistik" or "Statistik und Data Science", as well as a major in "Statistik" (Prüfungsordnung 2010).
Schedule:
day/time | location | instructor | start date | |
---|---|---|---|---|
Lecture/Tutorial | Fri, 12:00-16:00 | Schellingstr. 3 (S) - S 007 | Farsani | 21.04.23 |
Introduction to Bayesian Statistics for Bachelor and Minor Statistics and Data Science
Registration key: Bayes23
- Викладач: Aminifarsani Zahra
- Викладач: Schmid Volker
The course Analysis of high-dimensional biological data (formerly known as Statistische Methoden in Genomik und Proteomik/Statistical methods for biological high-throughput data) will cover important statistical methods and concepts for the analysis of high-dimensional biological high-throughput data. We will focus on bulk RNA-Seq, single-cell RNA-Seq, proteomic, metabolic, and, in particular, microbiome such as 16S rRNA and other amplicon data.
- Викладач: Müller Christian
- Викладач: Peschel Stefanie
- Викладач: Boulesteix Anne-Laure
- Викладач: Hoffmann Sabine
- Викладач: Kümpel Hannah
Termine:
Termin | Ort | Person | Beginn | |
---|---|---|---|---|
Vorlesung | Mo, 9:15-11:45 | Geschw.-Scholl-Pl. 1 (E) / E 004 | Hoffmann/Boulesteix | 17.04.23 |
Übung | Di, 10:15-11:45 | Geschw.-Scholl-Pl. 1 (M) - M 109 | Rehms | 18.04.23 |
Einschreibeschlüssel
- Der Einschreibeschlüssel lautet: "DAS2023"
- Викладач: Boulesteix Anne-Laure
- Викладач: Hoffmann Sabine
- Викладач: Kümpel Hannah
- Викладач: Rehms Raphael
The enrolment key for the course is: FortStatSoftNF.
If you have questions please contact statprog@stat.uni-muenchen.de.
The course will be taught in English, more information can be found in the course itself.
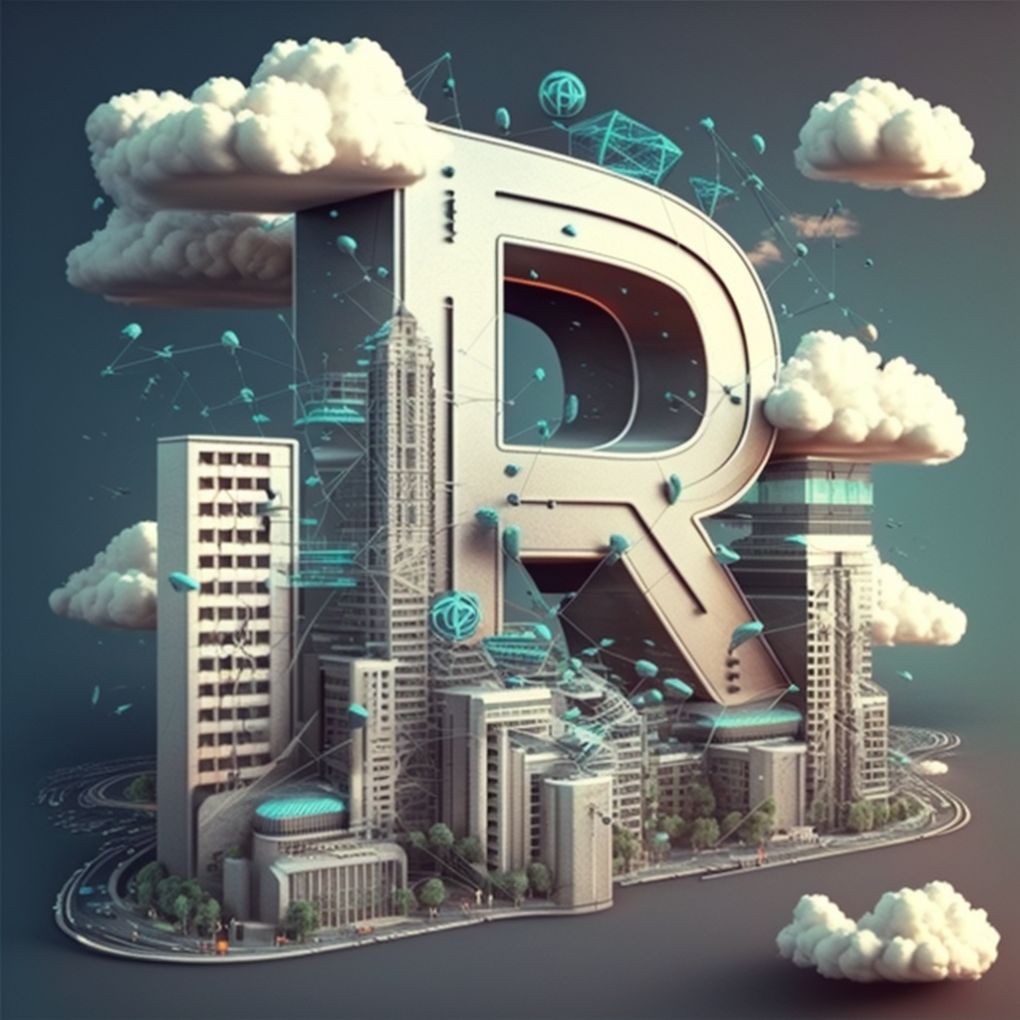
- Викладач: Beck Jacob
- Викладач: Haensch Anna-Carolina
- Викладач: Herklotz Markus
- Викладач: Simson Jan
The enrollment key is: Ganter
Termine (Beginn jeweils c.t.):
Mo |
12 - 14 |
Schellingstr. 3 (R) /
R 312
|
|
---|---|---|---|
Di |
08 - 10 |
Geschw.-Scholl-Pl. 1 (B) /
B 006 |
|
Do |
14 - 16 |
Geschw.-Scholl-Pl. 1 (M) /
M 118
|
- Викладач: Blocher Hannah
- Викладач: Schollmeyer Georg
Enrolment key: StatModSs23
Session | Instructor | Schedule | Rythm | Room |
---|---|---|---|---|
Lecture | Helmut Küchenhoff | Monday: 14:00-16:00 Thursday: 12:00-14:00 | weekly | Monday: Geschw.-Scholl-Pl. 1 (M) - M 218 Thursday: Geschw.-Scholl-Pl. 1 (B) - B 101 Live zoom: Meeting ID: 6846774868 Password: 654336 Videos : https://cast.itunes.uni-muenchen.de/vod/playlists/5wDnYPs55a.html |
Work Shop | Martje Rave | Thursday: 10:00-12:00 | weekly | Thursday: Schellingstr. 3 (S) - S 001 |
Tutorial | Thursday: 8:00-10:00 | weekly | Thursday: Geschw.-Scholl-Pl. 1 (A) - A 021 |
- Викладач: Klima André
- Викладач: Küchenhoff Helmut
- Викладач: Rave Martje
- Викладач: Schlichting Daniel
- Викладач: Schulte Karl
- Викладач: Strasser Ceballos Clara
- Викладач: Syliqi Dielle
- Викладач: Weigert Maximilian
Persons und Dates
Time | Place | Lecturers | |
---|---|---|---|
Lecture | Mo 4-6pm | Geschw.-Scholl-Pl. 1 (M) - M 014 / Zoom | Mina Rezeai, David Rügamer |
Lab Session | Tue 10-12am | Geschw.-Scholl-Pl. 1 (A) - A 120 / Zoom |
Anil Gündüz |
Enrollment key: learnDL
- Викладач: Gündüz Hüseyin
- Викладач: Rezaei Mina
- Викладач: Rügamer David
- Викладач: Sommer Emanuel
The course (timeline) will be project-based with individual meetings between project supervisor and students
Enrollment key: applyDL
- Викладач: Rezaei Mina
- Викладач: Rügamer David
Vorlesung | Mo 10–12 | Schellingstr. 3 (S) - S 004 | David Rügamer |
Di 10–12 | Schellingstr. 3 (S) - S 005 | ||
Übung Gruppe 1 | Mo 14–16 | Schellingstr. 3 (S) - S 005 | Viet Tran + Dominik Kreiß |
Übung Gruppe 2 | Mi 8–10 | Geschw.-Scholl-Pl. 1 (E) - E 004 | |
Tutorium | Di 16–18 | Geschw.-Scholl-Pl. 1 (M) - M 114 | Michael Kobl |
Hausübung | - | . | Max Lang |
Einschreibeschlüssel: WahrGrundStoff
- Викладач: Kobl Michael
- Викладач: Kreiß Dominik
- Викладач: Lang Max
- Викладач: Rügamer David
- Викладач: Tran Minh
Decision theory deals with rational decisions under uncertainty. It has high interdisciplinary importance, for example, in the analysis and support of decisions in business administration or finance (e.g. investment strategies), economics or sociology (rational choice theory), medicine (e.g. expert systems) or engineering (e.g. autonomous control). Moreover, statistical decision theory can be seen as a formal framework for choosing analysis methods (optimal tests or estimators, best classification algorithms, etc.). This general view, understanding statistics and machine learning as special cases of decision theory, plays a fundamental role in the critical analysis and problem-adequate generalization of any data-based learning procedure.
The course first discusses the general structure of decision problems, including fundamental decision principles. Then it analyzes and characterizes the Bayes and minimax criteria as extreme poles to deal with (state) uncertainty and develops modern alternatives in the context of complex uncertainty (ambiguity). In the second part, an overview of other decision-theoretic topics is given, also introducing to current research in decision theory.
The enrollment key is: A/DT23
- Викладач: Augustin Thomas
- Викладач: Jansen Christoph
- Викладач: Melev Ivan
Der Einschreibeschlüssel lautet: MatheIINF23
- Викладач: Jansen Christoph
- Викладач: Schollmeyer Georg
Enrolment Key
SDS-Methods-23
- Викладач: Jaime Sofia
- Викладач: Kern Christoph
Description
The lecture deals with theoretical and practical concepts from the fields of statistical learning and machine learning. The main focus is on predictive modeling / supervised learning. The tutorial applies these concepts and methods to real examples for illustration purposes.
Organization
- Class: Wednesday, 12:00-14:00 c.t.
- Location: Geschw.-Scholl-Pl. 1 / A 119
Enrolment Key
SL_s23
Target Audience
- Statistics MSc (Methods/Bio/WISO)
- Statistics and Data Science MSc
- Data Science MSc.
- Викладач: Bischl Bernd
- Викладач: Bothmann Ludwig
- Викладач: Kolb Chris
- Викладач: Pielok Tobias
Participants in this course will be automatically added based on the seminar assignments done by Dr. Schollmeyer.
If you have questions, write to ruegamer@stat.uni-muenchen.de
- Викладач: Gauß Jana
- Викладач: Gruber Cornelia
- Викладач: Kolb Chris
- Викладач: Nagler Thomas
- Викладач: Palm Nicolai
- Викладач: Pielok Tobias
- Викладач: Rehms Raphael
- Викладач: Rügamer David
- Викладач: Weber Tobias
- Викладач: Wimmer Lisa
Bachelor Seminar "Introduction to Causal Inference"
Enrolment key: ci_seminar_2023
In this seminar, we’ll learn about the core statistical and philosophical concepts related to causal inference and explore some of the techniques that have been developed to answer causal questions based on data.
- Викладач: Bothmann Ludwig
- Викладач: Scheipl Fabian
- Викладач: Wimmer Lisa
Seminar degree module
Enrolment Key
Content
Areal data is a data format in which point observations are aggregated over subregions of a predefined space. These subregions are non-overlapping and make up the entire space. This format is predominately common in medical research and one of the central formats in which, for example, data on Corona infections and hospitalizations were available. Due to the loss of information on the specific point of observation, the estimation of the spatial correlation can become a bit trickier than in spatial point processes. In recent years, especially during the pandemic, working with this type of data has become more relevant for data scientists and statisticians not only due to the relevance of their context but also due to somewhat recent advancements in research on network or graph theory, which allowed research on the statistical methods designed to work with this data more diverse.
Format
- Language In English. English by default.
- Attendance is mandatory for every session. If you miss more than one session without providing a reasonable excuse in time, you won’t pass.
- Where: Probably offline, possible to do go hybrid, if needed.
- Schedule:
- Kick off (within the first two weeks of the semester) we will talk about the basics of spatial statistics
- Phase
1: Foundations (2 weeks)
- In the first 2 weeks after the kick off, we’ll meet weekly (!) to discuss a specific section of Spatial Statistics and Modeling (Gaetan) We’ll work through chapter 5.2 and 5.3 as well as some excerpts of Applied Spatial Data
- Analysis with R (Bivand) at first to establish a foundation for us to work with.
- Phase 2: Book club (6 weeks)
- You will be asked to present a paper out of a selection of papers to the other students (45 mins to 1 hour) thereafter we will discuss the content of the presentation (30%= 20% presentation+ 10% discussion)
- Phase
3: Implementation (3 weeks)
- You will be asked to pick a dataset out of the ones provided or find your own in which you will apply one of the methods you found interesting in the book club phase.
- Phase
4: Presentations (1 week)
- You will hold a short presentation 10-15 mins about your project. (30%=20% presentation+ 10% discussion)
- Phase 5:
- You will write and hand in a final report on your implementation; this does not have to be handed in before the end of the semester, but should be within 2 or 3 weeks of the last day of lectures. (Not more than 10 pages) (40%)
Grading
- Presentation paper+ discussion: 30%
- Presentation work+ discussion: 30%
- Thesis: 40%
References
Phase II:
- Applied Spatial Data Analysis with R (Roger S. Bivand)
- Spatial Statistics and Modeling (Gaetan)
Phase III:
Tbd
Phase IV:
Tbd
- Викладач: Rave Martje
- Викладач: Sapargali Nurzhan
Termine und Personen:
Termin | Ort | Person | |
---|---|---|---|
Vorlesung |
Mo, 12.00 - 14.00 |
Geschw.-Scholl-Pl. 1 - E 216
|
Küchenhoff / Bender |
Vorlesung |
Do, 08.30 - 10.00 |
Schellingstr. 3 - S 004 |
Küchenhoff / Bender |
Übung |
Di, 10.00 - 12.00 |
Geschw.-Scholl-Pl. 1 - F 007 |
Rave / Weigert |
Übung |
Do, 14.00 - 16.00 |
Geschw.-Scholl-Pl. 1 - F 007 |
Rave / Weigert |
Tutorium |
Di, 12.00 - 14.00 |
Geschw.-Scholl-Pl. 1 - A 014 |
Alber |
Einschreibeschlüssel: LiMo23
- Викладач: Alber Helen
- Викладач: Bender Andreas
- Викладач: Küchenhoff Helmut
- Викладач: Rave Martje
- Викладач: Weigert Maximilian
Schedule
Type |
Date |
Location |
|
---|---|---|---|
Initial Meeting |
May 2nd 9:00-12:30 |
Seminar Room 144. Ludwigstr. 33 |
- Викладач: Olivares Gonzalez Mauricio
- Викладач: Olma Tomasz
Der Einschreibeschlüssel für den Kurs ist Stat2SozNF. Bei Fragen wenden Sie sich bitte an sarah.ball@stat.uni-muenchen.de. Eine Einschreibung übers LSF ist nicht notwendig.
- Викладач: Ball Sarah
- Викладач: Beck Jacob
- Викладач: Haensch Anna-Carolina
- Викладач: Kreuter Frauke
- Викладач: Schüttpelz Robin
- Викладач: Simson Jan
- Викладач: von der Heyde Leah
- Викладач: Wicht Leonie
Grundkurs | Mo, 05.06., 16:00 - 19:00 | Ludwigstraße 28 RG, Raum III (023) |
Grundkurs | Di, 06.06., 16:00 - 19:00 | Ludwigstraße 28 RG, Raum III (023) |
Grundkurs | Mo, 12.06., 16:00 - 19:00 | Ludwigstraße 28 RG, Raum III (023) |
Grundkurs | Di, 13.06., 16:00 - 19:00 | Ludwigstraße 28 RG, Raum III (023) |
Grundkurs | Mo, 19.06., 16:00 - 19:00 | Ludwigstraße 28 RG, Raum III (023) |
Grundkurs | Di, 20.06., 16:00 - 19:00 | Ludwigstraße 28 RG, Raum III (023) |
Aufbaukurs | Mo, 26.06., 16:00 - 19:00 | Ludwigstraße 28 RG, Raum III (023) |
Aufbaukurs | Di, 27.06., 16:00 - 19:00 | Ludwigstraße 28 RG, Raum III (023) |
Aufbaukurs | Mo, 03.07., 16.00 - 19.00 | Ludwigstraße 28 RG, Raum III (023) |
Aufbaukurs | Di, 04.07., 16.00 - 19.00 | Ludwigstraße 28 RG, Raum III (023) |
- Викладач: Funk Henri
- Викладач: Gorich Eugen
- Викладач: Khazaei Yeganeh
- Викладач: Weigert Maximilian
Class + Exercise
- Time: Wednesday, 12:15-13:45
- Location: Geschw.-Scholl-Pl. 1 (D) - D 209
Tutorial
- Time: Tuesday, 14:15-15:45
- Location: Schellingstr. 3 (S) - S 004
Enrollment key
- The enrollment key is I2ML
- Викладач: Bothmann Ludwig
- Викладач: Kopper Philipp
- Викладач: Wimmer Lisa
Dates:
Date | Place | Person | Start | |
---|---|---|---|---|
Lecture | Wed, 12:15-13:45 | Geschw.-Scholl-Pl. 1 (A) / A 021 | Nagler | 18.04.23 |
Lecture/Exercise | Thu, 10:15-11:45 | Geschw.-Scholl-Pl. 1 (B) / B 106 | Nagler/Palm | 19.04.23 |
Enrolment
- The enrolment key is: "rademacher"
- Викладач: Gauß Jana
- Викладач: Nagler Thomas
- Викладач: Palm Nicolai
Wednesday, 10:15 - 11:45
s23_advml
- Викладач: Bischl Bernd
- Викладач: Casalicchio Giuseppe
- Викладач: Ewald Fiona
- Викладач: Li Yawei
- Викладач: Olivares Gonzalez Mauricio
See this document for a description of the seminar.
All other information TBA.
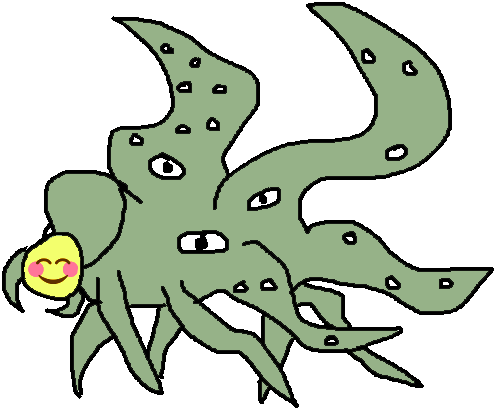
- Викладач: Binder Martin
- Викладач: Fischer Sebastian
- Викладач: Goschenhofer Jann
- Викладач: Rügamer David
- Викладач: Schneider Lennart
Termin | Ort | Person | Beginn | |
---|---|---|---|---|
Vorlesung | Di, 08:15-09:45 | tbd |
Nagler | 18.04.23 |
Vorlesung | Mi, 10:15-11:45 | tbd |
Nagler | 19.04.23 |
Übung | Mo, 16:15-17:45 | tbd |
Schiele |
24.04.23 |
Einschreibeschlüssel
- Der Einschreibeschlüssel lautet: "linalg"
- Викладач: Nagler Thomas
- Викладач: Schiele Philipp
Dates / Time |
Location | |
---|---|---|
Initial Meeting |
May (TBD) |
TBD |
Seminar / Presentations |
July (TBD) |
TBD |
- Викладач: Müller Christian
- Викладач: Peschel Stefanie
- Викладач: Tran Minh
Termine
- Vorlesung & Übung: Freitag, 09 - 12 c.t.
Einschreibeschlüssel
- Der Einschreibeschlüssel lautet "miniconda3".
- Викладач: Aßenmacher Matthias
- Викладач: Bothmann Ludwig
- Викладач: Feurer Matthias
- Викладач: Garces Arias Esteban
Termine
- Vorlesung: Dienstag, 16 - 18 c.t.
- Übungen (Statistik II):
Mittwoch, 12 - 14 c.t. (2x) & 14 - 16 c.t. (2x)
Donnerstag, 18 - 20 c.t.
Freitag, 10 - 12 c.t. - Übung (Statistik I):
Montag, 10 - 12 c.t.
Einschreibeschlüssel
- Der Einschreibeschlüssel lautet "20wiwistat23".
- Викладач: Amin Mostafa
- Викладач: Aßenmacher Matthias
- Викладач: Gordienko Polina
- Викладач: Heumann Christian
- Викладач: Küster Christopher
- Викладач: Mao Dennis
- Викладач: Prokosch David
- Викладач: Schiele Philipp
Termine:
Termin | Ort | Person | Beginn | |
---|---|---|---|---|
Vorlesung | Di, 12:15-13:45 | Geschw.-Scholl-Pl. 1 (A) - A 021 | Hoffmann/Kümpel/Garces Arias | 18.04.23 |
Vorlesung | Mi, 10:15-11:45 | Geschw.-Scholl-Pl. 1 (A) - A 125 | Hoffmann/Kümpel/Garces Arias | 19.04.23 |
Übung | Do, 10:15-11:45 | Geschw.-Scholl-Pl. 1 (A) - A 022 | Kümpel/Garces Arias | 27.04.23 |
Tutorium | Mo, 12:15-13:45 | Geschw.-Scholl-Pl. 1 (A) - A 016 | Kraft | 24.04.23 |
Einschreibeschlüssel: multi_verfahren_s23
- Викладач: Garces Arias Esteban
- Викладач: Hoffmann Sabine
- Викладач: Kraft Marie
- Викладач: Kümpel Hannah
- Викладач: Rehms Raphael