- معلم: Jacob Beck
- معلم: Jan Simson
Person: Dr. Cornelia Oberhauser
SAS-Kurs als 5-tägiger Blockkurs in den Semesterferien
Termine:
Tag |
Uhrzeit |
Raum |
|
---|---|---|---|
Mo 11.03.2024 |
Vorlesung | 9:15 - ca. 12:15 |
online über Zoom |
Übung |
13:15 - 17:00 |
online über Zoom |
|
Di 12.03.2024 |
Vorlesung |
9:15 - ca. 12:15 | online über Zoom |
Übung | 13:15 - 17:00 | online über Zoom | |
Do 14.03.2024 |
Vorlesung | 9:15 - ca. 12:15 | online über Zoom |
Übung | 13:15 - 17:00 | online über Zoom | |
Mo 18.03.2024 |
Vorlesung | 9:15 - ca. 12:15 | online über Zoom |
Übung | 13:15 - 17:00 | online über Zoom | |
Di 19.03.2024 |
Vorlesung | 9:15 - ca. 12:15 | online über Zoom |
Übung | 13:15 - 17:00 | online über Zoom |
Einschreibeschlüssel
- Der Einschreibeschlüssel lautet: "saskurs2024"
Gastschlüssel
- Der Gastschlüssel lautet: "saskurs2024"
- معلم: Cornelia Oberhauser
Course password: TBA
The course will begin on November 3rd.
More info coming soon!
- معلم: Verena Bauer
- معلم: Giacomo De Nicola
In the rapidly evolving field of biomedical research, the generation of
high-dimensional data has become a commonplace. This data deluge
presents both opportunities and challenges in extracting meaningful
insights. The seminar "Selected Topics in High-Dimensional Biomedical
Data Analysis" is designed for bachelor and master students. It aims to
teach students how to handle and analyze intricate biomedical data,
helping them gain a better grasp of the field.
- معلم: Christian Müller
- معلم: Stefanie Peschel
- معلم: Minh Tran
This course provides an introduction to the theory and application of methods for analyzing in- complete data sets. The main focus will be on Multiple Imputation (MI) which has become a very popular way for handling missing data, because it allows for correct statistical inference in the presence of missing data. With the advent of MI algorithms implemented in statistical stan- dard software (R, SAS, Stata, SPSS,. . . ), the method has become more accessible to data analysts. For didactic purposes, we start by introducing some naive ways of handling missing data, and we use the examination of their weaknesses to create an understanding of the framework of Multiple Imputation. Solid R skills are a prerequisite for this course.
Enrollment Key: Incomplete
- معلم: Thomas Augustin
- معلم: Dominik Kreiß
Financial econometrics is the subfield of
econometrics that investigates the theory of financial markets and the products that comes with it. A special
feature of financial data are the so-called "stylized facts", which do
not exist in a comparable way in any field of empirical economics. These
properties are significant in two respects: On the one hand, they have
led to the falsification or modification of popular, theoretical models
in the past. On the other hand, they have been the impetus for the
development of models that have been extremely successful in practice.
In this course we will study advanced technics of this field.
- معلم: Dennis Mao
Target Audience:
- Statistics bachelor students (preferably semester 5 and upwards) and
- Statistics master students (credits can be counted for “Methodology and Modelling” and “Biostatistics” specification )
In this seminar, we take a closer look at areal data, spatial point processes and related topics. The students are asked to present a publication in this area. Furthermore, they have to implement the in the publication presented algorithms and models based on a further spatial data set.
Spatial point processes are stochastic processes on a subspace of multidimensional real numbers. Their realisations are finite, random and countable observations. In other words, they are point events that occur in space/on earth/etc.. Examples of such data are locations of crimes, locations of disease outbreaks or tree locations.
Areal data is a data format in which point observations are aggregated over subregions of a predefined space. These subregions are non-overlapping and make up the entire space. This format is predominately common in medical research and one of the central formats in which, for example, data on Corona infections and hospitalizations were available. Due to the loss of information on the specific point of observation, the estimation of the spatial correlation can become a bit trickier than in spatial point processes.
- معلم: Hannah Blocher
- معلم: Martje Rave
- معلم: Anna-Carolina Haensch
- معلم: Frauke Kreuter
- معلم: Joseph Sakshaug
Date | Place | Person | Start | |
---|---|---|---|---|
Lecture | Friday, 12:15 - 13:45 | Geschw.-Scholl-Pl. 1 - A 016 | Schomaker | 20.10.23 |
Lecture/ Exercise Session | Thursday, 16:15-17:45 | Geschw.-Scholl-Pl. 1 - A 015 | Rehms/Kümpel | 19.10.23 |
Enrolment key
- The enrolment key is: "StatMetEpi2324"
- معلم: Hannah Kümpel
- معلم: Raphael Rehms
- معلم: Michael Schomaker
- معلم: Michael Schomaker
The "Advanced Programming (R)" course targets students in the Statistics and Data Science Master's programme (WP47).
The course can also be taken by advanced Bachelor's students
that have taken "Programmieren statistischer Software". For Bachelor students, Advanced
Programming can be credited as WP4/WP7 (Bachelor PO 2021), or WP2/WP8 (Bachelor PO 2010).
The first lecture will happen on Thursday, 2023-10-19, 18:00--20:00 c.t., location t.b.a.
Enrollment key: advaprogr2324
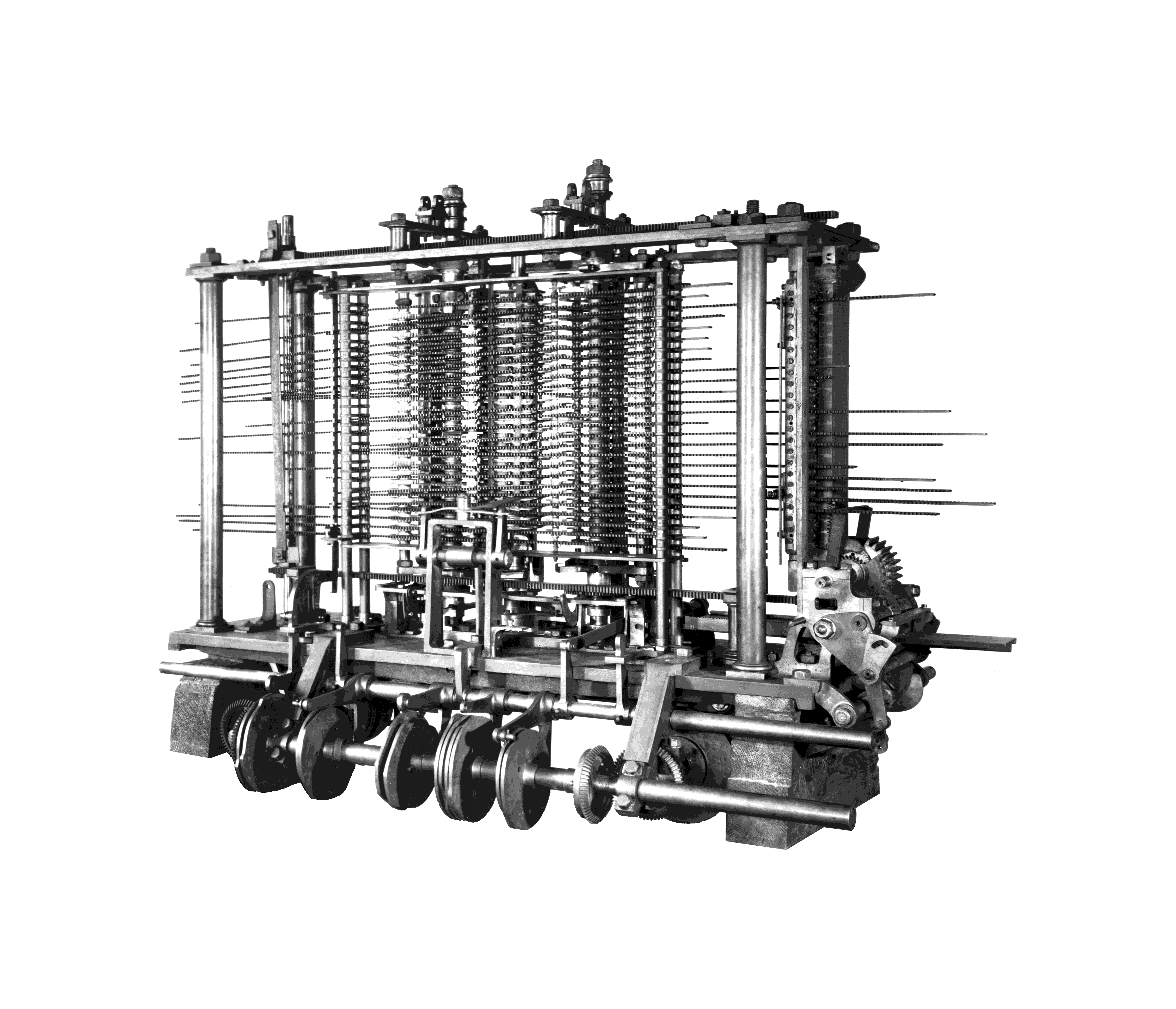
- معلم: Martin Binder
Die Veranstaltung "Programmieren
mit Statistischer Software (R)" wendet sich an Studenten im Bachelor
Statistik (3. Semester). Sie baut auf die Veranstaltung "Einführung in
die Statistische Software" (1. Semester) auf.
Termin | Ort | Person | |
---|---|---|---|
Vorlesung: | t.b.a. (!) |
t.b.a. |
Binder |
Uebung: |
t.b.a. | t.b.a. |
Binder |
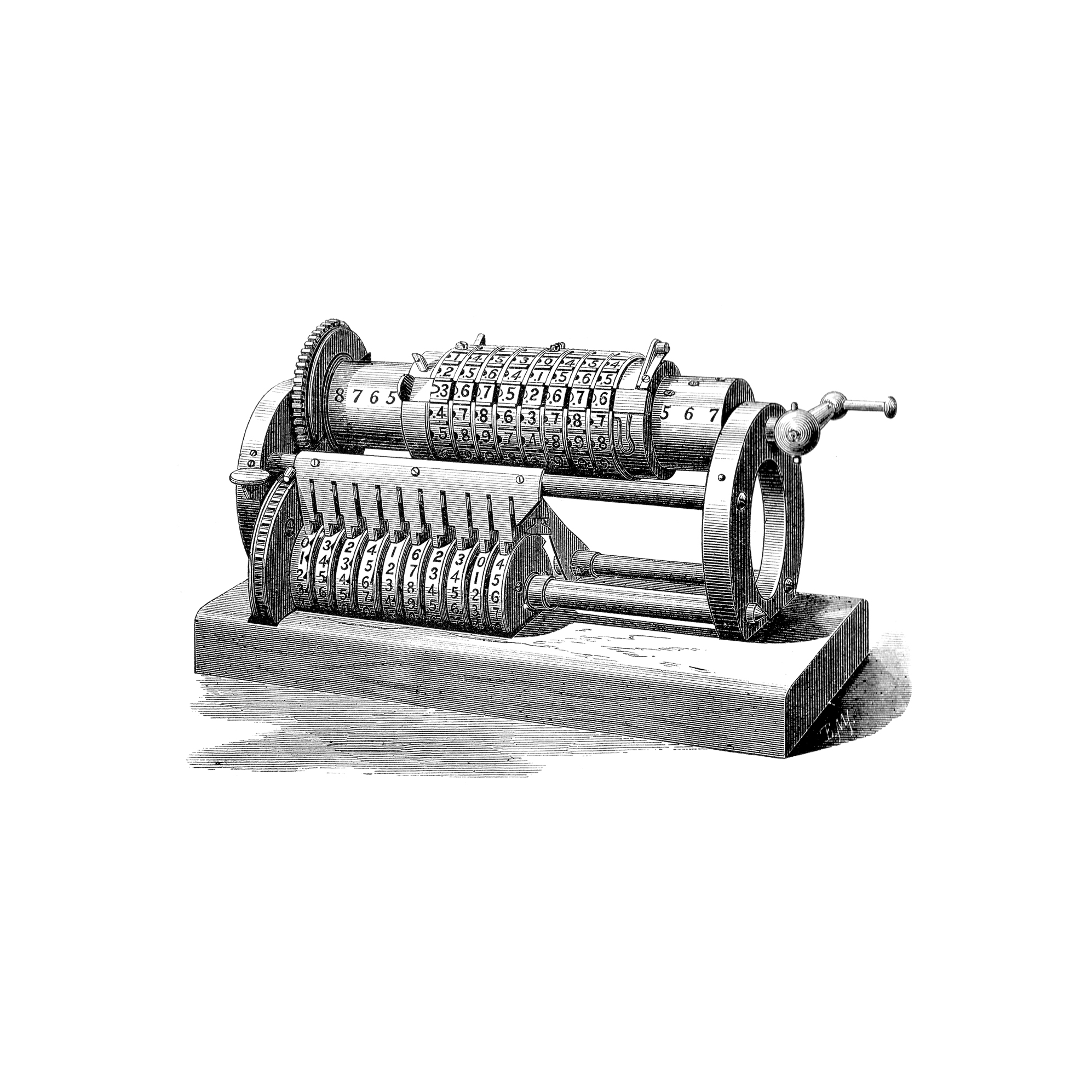
- معلم: Martin Binder
- معلم: Noah Hurmer
Welcome to the course "Statistics for Geosciences" in winter term 22/23!
Room: C 112
Date and time: Wednesday, 8.30h - 12h00 (weekly)
Enrolment key: rose-diagram
- معلم: Julian Rodemann
Official statistical agencies are the central information service providers in a democratically organized society, informing politics, business and society about current economic, social and increasingly also ecological developments. In this way, official statistics form an important basis for informed decisions. At the same time, official statistics act as a control authority, especially for politics, by empirically reflecting the consequences of decisions and actions. To meet this responsibility, official statistics production underlies a strict methodology and high-quality standards.
Against this background, there has also been an intensive discussion about the opportunities and challenges complex statistical modelling, machine learning techniques, and new data sources deliver. This development has also led to close cooperation of statistical authorities with a number of universities, resulting in an EU-wide certification of particular master's degree programmes (EMOS: European Master in Official Statistics). For students at LMU, it is possible to obtain an EMOS certificate by taking a specific route within the Machine Learning track and the Social Statistics and Data Science track.
The course "EMOS A" aims to prepare students for participation in the discourse between academic and official statistics and the numerous opportunities for cooperation with public data producers. It provides an insight into typical questions and modes of argumentation, methodological principles, and the most important products of official statistics. First, we take a look at current research concerning official statistics. Then, the basic principles and legal framework of official statistics, their fundamental structure in Germany/Europe and their most important supporting institutions are discussed as a basis. Further topics will then include the EU system of environmental indicators, the role of Big Data in official statistics, selected aspects of result dissemination and statistical literacy, and access to official data sources for (own) secondary analyses, including an introduction to statistical anonymization techniques.
The course format is a mix of classical lecture style, guest lectures and inverted classroom elements with in-person discussion and deepening of the topics. The course is compulsory for the EMOS variant; all other students can choose it as an elective module.
Enrollment Key: emosa
- معلم: Thomas Augustin
- معلم: Dominik Kreiß
Instructors:
Kick-off Meeting
- TBD (potentially on October 23, 4pm)
- Via Zoom
Credits and Contents
- Credits: 6 ECTS
- Applied Software Projects, working in a group of 1-3 students
- Final Submission Date: Open for Discussion
Enrollment key: TensorTorch
- معلم: David Rügamer
Einschreibeschlüssel: sample2023
- معلم: Verena Bauer
- معلم: Alexander Jäger
- معلم: Dominik Kreiß
- معلم: Ivan Melev
day/time | location | instructor | start date | |
---|---|---|---|---|
Lecture | Mon, 16:00-18:00 | Geschw.-Scholl-Pl. 1 (E) / E 006 | Bühler | 18.10.22 |
Tutorial | Wed, 8:30-10:00 | Geschw.-Scholl-Pl. 1 (E) / E 006 | Mao | 23.10.22 |
- معلم: Mathias Bühler
- معلم: Dennis Mao
This module discusses different
types of latent
structures and their statistical handling. Part A, taking place in the winter semester, will focus on
statistical aspects of measurement and modelling issues in
surveys and assessment studies. Part B, scheduled for the
summer semester, is planned to address statistical aspects of
combining data sets and different methods in the context of anonymization/privacy protection.
Concretely, Part A starts with classical testing theory (CTT) as a framework to describe the operationalization/measurement of continuous latent traits and utilizes CTT to derive and discuss critically well-known reliability measures such as Cronbach’s alpha. Then, different generalizations are studied, including structural equation models, Rasch-type models from probabilistic testing theory and situations with locally varying scales of measurement. In the second half of the semester, methods for handling incomplete data in regression models are investigated in more detail. Advanced frequentist and Bayesian correction methods for measurement error, misclassification, and missing data are developed. In this context, also an introduction to the framework of partial identification is given.
The module can be attended already in the first Master's semester. According to the Examination Regulations, the module is among the "narrow electives" in the Social Statistics and Social Data Science track, the "wide electives" in the Econometrics and Methodology and Modelling tracks and a potential "general elective" for all tracks. Alternatively, Part A or Part B can be recognized as 'Selected Topics of Social Statistics and Social Data Science'.
Time and Dates: Tuesday, 6.15 pm to 7.45 pm, E 216 (main building)
Enrolment Key: MeasMod
- معلم: Thomas Augustin
This course will discuss essential research techniques in statistics and data science, also supporting students in successfully participating in seminars and writing a thesis. It is part of the Bachelor’s programmes in Statistics and Data Science (150 major or 60 ECTS minors: `Methoden und Techniken des wissenschaftlichen Arbeitens’, P 16.1, and WP 12.1 / WP 13.1, respectively). In addition, all interested Master’s students and Bachelor's students from the "old PO" are most welcome as well.
We will meet mainly in the first half of the semester from 6.15 pm to 8 pm on the following Wednesdays: Oct 25th, Nov 8th, Nov 15th, Nov 22nd, Nov 29th, Dec 6th, and Jan 10th.
The following topics will be addressed:
Background: Science and Research
Professional Literature Search (with some Hands-on Tutorials)
Reading Research Papers
Presenting Research
Designing Simulation Studies
Some Further Tips and Tricks: Mutual Exchange of Experiences
Einschreibeschlüssel: wissArb
- معلم: Thomas Augustin
Description
A variety of digital data sources are providing new avenues for empirical social science research. With the emergence of Big Data, especially data from web sources play an increasingly important role in scientific research. In order to effectively utilize these data for answering substantive research questions, a modern methodological toolkit paired with a critical perspective on data quality is needed. This course introduces computational techniques that are suited for collecting and analyzing digital behavioral data, and for exploring, visualizing and finding patterns in (different types of) data from various sources. In addition, aspects of reproducibility, data quality and error frameworks for digital data are discussed.
Enrolment Key
CSSws2324
- معلم: Paul Bauer
- معلم: Unai Fischer Abaigar
- معلم: Christoph Kern
- معلم: Olga Kononykhina
Der Einschreibeschlüssel lautet: STDT2324
- معلم: Christoph Jansen
- معلم: Julian Rodemann
Enrolment key: w2324-optim
Kickoff
Thu, October 19, 2pm at room S 006 (Schellingstr. 3)
Credits
6 ECTS
Format
3 hours lecture, 1 hour exercise
Description
The course introduces the theoretical foundation of optimization as well as the most prominent methods in this field. It covers the taxonomy of optimization problems and other basic principles of optimization, considering univariate and multivariate problems and commonly used approaches to tackle these. This contains first- and second-order methods as well as stochastic approaches. The course further deals with constrained optimization problems, derivative-free methods as well as multi-criteria optimization.
Contents
- Mathematical concepts
- Optimization problems
- Univariate optimization
- First order methods
- Second order methods
- Constrained optimization
- Derivative-free optimization
- Evolutionary optimization
- Bayesian optimization
- معلم: Mario Teixeira Parente
Der Einschreibeschlüssel lautet: MatheINF
- معلم: Armin Egetenmeier
- معلم: Christoph Jansen
- معلم: Georg Schollmeyer
Einschreibeschlüssel: w2324-fmm
Start
Di., 17. Oktober, 16 Uhr in Raum E 004 (Hauptgebäude)
Credits
6 ECTS
Format
2 SWS Vorlesung, 2 SWS Übung
Inhalt
- Kondition, Stabilität und Komplexität
- Numerische Lineare Algebra
- Numerische Integration und Differentiation
- Differentialgleichungen
- Numerische Optimierung
- Interpolation und Funktionsapproximation
- معلم: Thomas Nagler
- معلم: Mario Teixeira Parente
Grundkurs | Mo, 26.02., 10:00 - 17:00 | Ludwigstraße 28 RG, Raum III (023) |
Grundkurs | Di, 27.02., 10:00 - 17:00 | Ludwigstraße 28 RG, Raum III (023) |
Grundkurs | Mi, 28.02., 10:00 - 17:00 | Ludwigstraße 28 RG, Raum III (023) |
Aufbaukurs | Do, 29.02., 10:00 - 17:00 | Ludwigstraße 28 RG, Raum III (023) |
Aufbaukurs | Fr, 01.03., 10:00 - 17:00 | Ludwigstraße 28 RG, Raum III (023) |
- معلم: Juliet Fleischer
- معلم: Henri Funk
- معلم: Eugen Gorich
- معلم: Lisa Kleinlein
- معلم: Mila Pfander
- معلم: Johannes Piller
- معلم: Helena Veit
- معلم: Simon Wiegrebe
Schedule
Time | Lecturer | Begin | |
---|---|---|---|
Exercise course (Group 1) | Tuesday, 10:15 - 11:45 | Sapargali, Garces Arias | 24.10.2023 |
Lecture | Tuesday, 16:15 - 17:45 | Garces Arias | 17.10.2023 |
Lecture | Friday, 14:15 - 15:45 | Garces Arias | 18.10.2023 |
Exercise course (Group 2) | Thursday, 08:15 - 09:45 | Sapargali, Garces Arias | 26.10.2023 |
Tutorium | Friday, 08:15 - 09:45 | Stephan | 21.04.2023 |
Enrollment Key
- The enrollment key is "stat_inf_w2324"
- معلم: Stephan Bark
- معلم: Esteban Garces Arias
- معلم: Nurzhan Sapargali
Selbsteinschreibung mit dem Passwort WiSo2024
- معلم: Thomas Augustin
- معلم: Anna-Carolina Haensch
- معلم: Frauke Kreuter
Enrolment key: InfStat12024!
- معلم: Jan Anders
- معلم: Giacomo De Nicola
- معلم: Göran Kauermann
- معلم: Michael Windmann
Instructor: Frauke Kreuter
Key: SoDa2023
- معلم: Anna-Carolina Haensch
Einschreibeschlüssel
- Der Einschreibeschlüssel lautet: "stat3nf2023"
- معلم: Neela Bhuiyan
- معلم: Malte Schierholz
Personen und Termine
Tag und Uhrzeit | Hörsaal | Dozent | |
Vorlesung | Mo 10-12 Uhr | Geschw.-Scholl-Pl. 1 (A) - A 120 | David Rügamer Fabian Scheipl |
Di 10-12 Uhr | Schellingstr. 3 (S) - S 006 | ||
Übung | Do 14-16 Uhr | Geschw.-Scholl-Pl. 1 (A) - Audi Max (A030) | Nurzhan Sapargali |
Tutorium | Fr 10-12 Uhr | Geschw.-Scholl-Pl. 1 (M) - M 018 | Emanuel Sommer |
Einschreibeschlüssel: 5tati5tik
- معلم: David Rügamer
- معلم: Nurzhan Sapargali
- معلم: Fabian Scheipl
- معلم: Karl Schulte
- معلم: Emanuel Sommer
Erster Termin: Voraussichtlich Donnerstag der 02. November 2023 sowohl von 10:15-11:45 Uhr: Alte Bibliothek (Raum 245, Ludwigstr. 33, 2. Stock)
, als auch von 16:15 -18:00 Uhr : Prof.-Huber-Pl. 2 (V) - LEHRTURM-V005
(Im späteren Verlauf kann dann vermutlich nur ein zeitslot angeboten werden.)
Einschreibeschlüssel: Fragestunde
- معلم: Armin Egetenmeier
- معلم: Georg Schollmeyer
Schedule:
Wednesday 10-12 Geschw.-Scholl-Platz 1 (A) - A 014
Thursday 12-14 Geschw.-Scholl-Platz 1 (A) - A 014
- معلم: Vincent Starck
Bitte einschreiben einschreibeschlüssel : statp24
- معلم: Paul Bauer
- معلم: Helmut Küchenhoff
- معلم: Johannes Piller
- معلم: Leah von der Heyde
Sie können sich in den Kurs mit dem Schlüssel DHS2324 selbst einschreiben.
- معلم: Franziska Danner
- معلم: Florian Fleischmann
- معلم: Helmut Küchenhoff
Date | Place | Person | Start | |
---|---|---|---|---|
Lecture | Tuesday, 9:15-11:45 | Geschw.-Scholl-Pl. 1 - A 014 | Boulesteix/Hoffmann | 17.10.23 |
Exercise Session | Monday, 14:15-15:45 | Geschw.-Scholl-Pl. 1 - A 120 | Sauer/Wünsch | 06.11.23 |
Enrolment key
- The enrolment key is: "PCS2324"
- معلم: Anne-Laure Boulesteix
- معلم: Sabine Hoffmann
- معلم: Christina Sauer
- معلم: Milena Wünsch
Schedule:
- Lecture: Monday, 16-18 c.t., Geschw.-Scholl-Pl. 1 - A 017 (starting Monday, 16 October 2023)
- Lecture/Exercise: Wednesday, 10-12 c.t., Geschw.-Scholl-Pl. 1 - A 016 (starting Wednesday, 25 October 2023)
Enrollment key:
life23Time
- معلم: Andreas Bender
- معلم: Simon Wiegrebe
Termine:
Termin | Ort | Person | Beginn | |
---|---|---|---|---|
Vorlesung | Di, 12:15-13:45 | Geschw.-Scholl-Pl. 1 (A) - A 120 | Hoffmann/Kümpel | 17.10.23 |
Vorlesung/Übung | Mi, 14:15-15:45 | Geschw.-Scholl-Pl. 1 (A) - A 120 | Mandl | 18.10.23 |
Einschreibeschlüssel
- Der Einschreibeschlüssel lautet: "EinfBiom2324"
- معلم: Sabine Hoffmann
- معلم: Hannah Kümpel
- معلم: Maximilian Mandl
Selbsteinschreibungsschlüssel: grlgprkt
Die
Veranstaltung wendet sich an Studierende im Bachelor Statistik (3.
Semester). Das "Grundlegende Praxisprojekt" (BA Statistik und Data
Science - PO 2021) ist
eine Pflichtveranstaltung (Modul P 11.1).
Die Veranstaltung wird sowohl während der Vorlesungszeit als auch
in den Semesterferien angeboten. Diese Moodle-Seite ist für beide Veranstaltungen.
Für beide Blöcke findet eine Einführungsveranstaltung mit Anwesenheitspflicht am 19.10. 10-12 statt.
Aus organisatorischen Gründen ist eine frühzeitige, separate Anmeldung für die Teilnahme während der Vorlesungszeit nötig -- schreiben Sie sich bitte ein und gehen Sie dann zur Anmeldung auf der Kursseite.
- معلم: Fabian Scheipl
- معلم: Karl Schulte
Einschreibeschlüssel: dskrpt
Termin | Ort | Person | |
---|---|---|---|
Vorlesung |
Do, 14.00 - 16.00 |
A140 (Hauptgebäude) |
Fabian Scheipl |
Vorlesung |
Fr, 10.00 - 12.00 | M118 (Hauptgebäude) |
Fabian Scheipl |
Übung 1 |
Do, 12.00 - 14.00 |
B106 (Hauptgebäude) |
Yichen Han |
Übung 2 |
Di, 16.00 - 18.00 |
C123 (Theresienstr. 41) |
Michael Kobl |
Tutorium |
Di, 18.00 - 20.00 |
S001 (Schellingstr. 3) |
Michael Kobl |
Diese Veranstaltung vermittelt elementare Wahrscheinlichkeitsrechnung sowie Grundlagen der deskriptiven und explorativen Statistik. Dies umfasst grundlegende Axiome und Rechenregeln für Wahrscheinlichkeiten (auch: bedingte und gemeinsame Wahrscheinlichkeiten) sowie die Begriffe der stochastischen und empirischen Unabhängigkeit für Ereignisse und Zufallsvariablen bzw. Merkmale. Die Lerninhalte umfassen auch eine erste einfache Begriffsbildung für und Eigenschaften von Zufallsvariablen, ihrer Wahrscheinlichkeitsdichten und Momente und wichtige parametrischer Verteilungsmodelle. Auf empirischer Seite werden entsprechend Skalenniveaus beobachteter Merkmale und einfache Erhebungsformen definiert und Techniken der uni- und multivariaten deskriptiven Statistik eingeübt: zum einen Datenvisualisierung anhand statistischer und wahrnehmungspsychologischer Leitlinien, zum anderen empirische Verteilungen und Kerndichten. Kennzahlen für Lage, Streuung, Schiefe, Wölbung, Konzentration und Assoziation werden eingeführt und ihre Eigenschaften intensiv diskutiert. Letzteres umfasst auch eine erste Einführung in die Probleme kausaler Interpretation von beobachteten Assoziationen.
Die Vorlesung (6 ECTS) entspricht Modul P3.1, die Übung (3 ECTS) dem Modul P4.1 des BA-Studiengangs Statistik und Data Science (PO 2021).
Vorlesungsmodul auch anrechenbar für Studierende mit HF Mathematik oder HF Informatik (mit integriertem Anwendungsfach Statistik).
- معلم: Michael Kobl
- معلم: Fabian Scheipl
Sie addressiert Studierende im ersten Semester und erfordert keine Vorkenntnisse.
Kurssprache ist Deutsch, ein Großteil der Unterlagen wird jedoch auf Englisch gestellt werden.
Einschreibeschlüssel: statsoft2324
- معلم: Andreas Bender
- معلم: Martin Binder
- معلم: Philipp Kopper
Statistik I für Studierende der Soziologie, des Nebenfachs Statistik, der Medieninformatik und der Cultural and Cognitive Linguistics
Sie müssen und können sich NICHT über das lsf für die Veranstaltung anmelden, schreiben Sie sich bitte einfach mit dem Einschreibeschlüssel in diesen Moodle Kurs ein. Alle erhalten einen Platz und es gibt keine Belegfristen.
Einschreibeschlüssel: Stat1NF
Vorlesung Anna-Carolina Haensch (anna-carolina.haensch@stat.uni-muenchen.de)
Übung Jacob Beck, Sarah Ball
- معلم: Sarah Ball
- معلم: Jacob Beck
- معلم: Anna-Carolina Haensch
- معلم: Robin Schüttpelz
- معلم: Jan Simson
- معلم: Leah von der Heyde
- Wednesdays, 10:15-12:00; Theresienstr. 39 - B 138
- Thursdays, 16:15-18:00; Schellingstr. 3 (S) - S 006
- معلم: Tomasz Olma
Dieser Kurs wird Studierenden mit NF Statistik eine Einführung in die statistische Software (R) geben.
Der
Kurs setzt sich aus einer Mischung aus Flipped-Classroom Lehrvideos,
Hands-On Lab-Sessions und Drop-In Hilfe-/Beratungssession zusammen.
Weitere Infos folgen in Kürze.
Sie müssen und können sich NICHT
über das lsf für die Veranstaltung anmelden, schreiben Sie sich bitte
einfach mit dem Einschreibeschlüssel StatSoftNF in diesen Moodle Kurs ein.
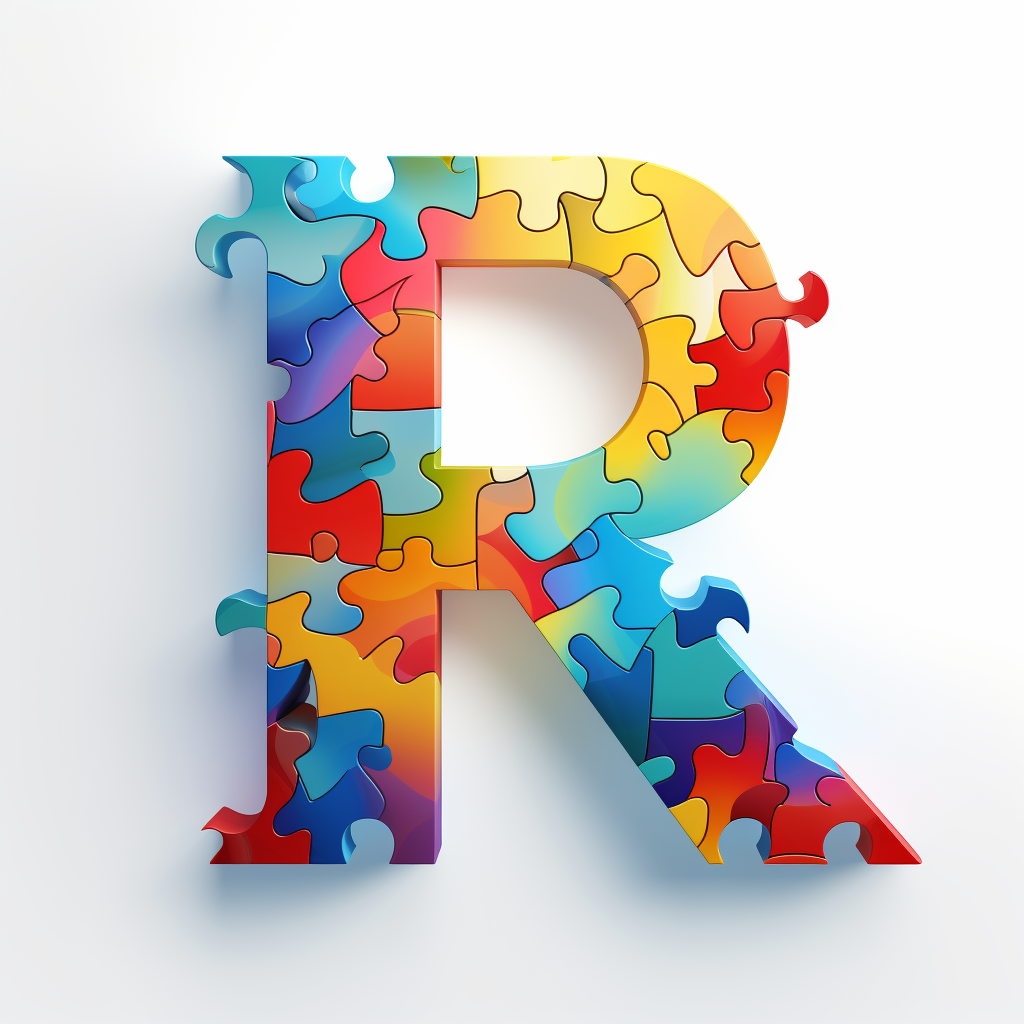
- معلم: Jacob Beck
- معلم: Anna-Carolina Haensch
- معلم: Jan Simson
Format
Class
- Time: Thursday 10:15-11:45 h
- Location: M 010
Exercise
- Time: Wednesday 12:15-13:45 h
- Location: E 004
Enrollment key
- The enrollment key is I2ML.
- معلم: Ludwig Bothmann
- معلم: Lisa Wimmer
The seminar bridges the realms of statistics and data science. While statistical inference offers tools to draw reliable conclusions from limited data, data science including machine learning and deep learning harnesses these insights to predict and recognize patterns. The course will delve into the questions that statistical inference can tackle in data science and the challenges that emerge in data-centric analyses.
- Kick-off date: October 27, 2023 (via Zoom)
- Presentations during the semester on 1 or 2 Fridays
Preliminary Topics:
- معلم: Jana Gauß
- معلم: Cornelia Gruber
- معلم: David Rügamer
- معلم: Karl Schulte
- معلم: Emanuel Sommer
Inverted classroom style. Weekly meeting (in person): TBD
Starting date: First week of term, exact date TBD
Enrollment Key: automl2324
- معلم: Matthias Feurer
- معلم: Lennart Schneider
MA seminar on multi-objective hyperparameter optimization and multi-objective machine learning algorithms.
The enrollment key is "hpo".
For questions please contact Matthias Feurer at matthias.feurer@stat.uni-muenchen.de
- معلم: Matthias Feurer
The lecture deals with theoretical and practical concepts from the fields of statistical learning and machine learning. The main focus is on predictive modeling / supervised learning. The tutorial applies these concepts and methods to real examples for illustration purposes.
key: SL_ws2324
- معلم: Bernd Bischl
- معلم: Chris Kolb
- معلم: Yawei Li
- معلم: Tobias Pielok
Session | Instructor | Schedule | Rythm | Room |
---|---|---|---|---|
Lecture | Helmut Küchenhoff | Tuesday: 12:15-13:45 Thursday: 12:15-13:45 | weekly (Both sessions discuss new topics) | Geschw.-Scholl-Pl. 1 (E) - E 004 Geschw.-Scholl-Pl. 1 (A) - A 140 |
Work Shop | Martje Rave | Monday: 12:15-13:45 Thursday: 10:15- 13:45 | weekly (Both sessions discuss the same topic that week) | Schellingstr. 3 (S) - S 001 Schellingstr. 3 (R) - R 051 |
- معلم: Henri Funk
- معلم: Helmut Küchenhoff
- معلم: Paul Messer
- معلم: Johannes Piller
- معلم: Martje Rave
- معلم: Daniel Schlichting
- معلم: Simon Wiegrebe
Teilnehmende dieses Seminars werden basierend auf der offiziellen Seminareinteilung von Dr. Schollmeyer dem Moodle-Kurs hinzugefügt.
Bei Fragen wenden Sie sich bitte an ludwig.bothmann@stat.uni-muenchen.de
- معلم: Ludwig Bothmann
- معلم: Christoph Kern
- Seminar für Bachelor Statistik und Data Science
- Anmeldung/Platzvergabe über LSF
Bayesianische Methoden werden in der Sportwissenschaft immer beliebter. Zu den ausgewiesenen Vorteilen des Bayesianischen Ansatzes gehört die Möglichkeit, komplexe Probleme zu modellieren, Schätzungen und Vorhersagen unter Berücksichtigung von Unsicherheiten zu erhalten, Informationsquellen zu kombinieren und gelerntes Wissen in Abhängigkeit von neu verfügbaren Daten zu aktualisieren. Das Ausmaß und die Vielfalt der Daten, die in den letzten Jahren im Sportbereich produziert wurden, und die Verfügbarkeit von Softwarepaketen für Bayesianische Analysen haben erheblich zu diesem Wachstum beigetragen.
In diesem Seminar werden wir verschiedene Anwendungen Bayesianischer Methoden in der Sportanalytik kennenlernen. Die Teilnehmer werden jeweils eine Methode vertieft theoretisch darstellen und praktisch anwenden.
Einschreibeschlüssel für Interessenten: Interesse
Zeitplan
- Vorbesprechung Mitte November 2023
- Präsentation der Theorie Januar 2024
- Abgabe Arbeiten Ende März 2024
- معلم: Volker Schmid
Master course
- Master Statistics & Data Science – WP44 Advanced Statistical Modelling
- ESG Data Science – Elective
- Master Statistik (mit WiSo), Biostatistik – Ausgewählte Gebiete... oder Schätzen und Testen II (mit Zusatzleistung)
Bayesian methods have seen increasing use within statistics in the last couple of years. In Bayesian frameworks, prior knowledge can be integrated as prior distribution. These also allows to regularise parameters in large p>>n models via priors, up to the point where the prior becomes part of the statistical model itself.
In this course, advanced Bayesian models will be discussed. These include several types of latent models, in particular latent Gaussian fields, applied for example to time series and spatial data, mixed effect models and other structured regression models. The course will also cover different type of algorithms to assess the posterior, based on Monte Carlo methods and on approximation. Further topics include Bayesian model and variable selection and Bayesian classification.
Requirements:
Students should be familiar with basics of Bayesian statistics.
Recommended:
- Statistical inference
- Statistical modelling
Learning outcomes:
After successfully completing the course, students will be able
- to understand Bayesian models
- to apply Bayesian modelling for appropriate data
- to interpret the results of Bayesian models
- to implement Bayesian models in standard software
- معلم: Volker Schmid
Master course
- Master Statistics & Data Science – WP45
- ESG Data Science – Elective
- Master Statistik (mit WiSo), Biostatistik – Räumliche Statistik
- معلم: Martje Rave
- معلم: Volker Schmid
Teacher: Walter J. Radermacher
Runtime: 1. October 2023 - 12. October 2023
Format: Self-learning through online videos in the first week and in-person workshops for practical appliance of use cases in the second week.
Examination: Oral Exam (3 ECTS credits)
Language: English
Who is this course for? MsC Statistics and Data Science (2021, WP 28+29+40+46), BsC Statistics and Data Science (2021, WP 8+11), Statistik und Data Science als Nebenfach für Bachelor 30 ECTS (2021, WP 4+5), Statistik und Data Science als Nebenfach für Bachelor 60 ECTS (2021, WP 11+12), Statistik und Data Science als Nebenfach 30 ECTS Mathematik (2021, WP 5+6), Statistik Nebenfach 60 ECTS Bachelor Soziologie (2021, WP 10+11), WISO Mater (2010, "Ausgewählte Gebiete..." (3ECTS))
- معلم: Markus Herklotz
- معلم: Walter Radermacher
Termine
- Vorlesung: Dienstag, 16 - 18 c.t.
- Übungen (Statistik I):
Mittwoch, 12 - 14 c.t. & 14 - 16 c.t.
Donnerstag, 10 - 12 c.t. (2x), 12 - 14 c.t. (2x), 18 - 20 c.t. - Übung (Statistik II):
Donnerstag, 08 - 10 c.t.
Einschreibeschlüssel
- Der Einschreibeschlüssel lautet "23wiwistat24".
- معلم: Matthias Aßenmacher
- معلم: Benjamin Dornow
- معلم: Sarah Grimm
- معلم: Antonio Melieni
- معلم: David Prokosch
- معلم: Franziska Reichmeier
- معلم: Teresa Rupprecht
- معلم: Michael Sawitzki
- معلم: Aïsha Schuhegger
Schedule
- Lecture: Wednesday, 9 - 11 c.t.
- Tutorial: Wednesday, 11 - 12 c.t.
- Location: Oettingenstr. 67, B U101 (Raumfinder)
Enrollment key
- Enrollment key: flash_attention
- معلم: Matthias Aßenmacher
- معلم: Marwin Härttrich
- معلم: Yihong Liu
- معلم: Hinrich Schütze
- معلم: Leonie Weißweiler
- معلم: Ingo Ziegler
Teilnehmende dieses Seminars werden basierend auf der offiziellen Seminareinteilung von Dr. Schollmeyer dem Moodle-Kurs hinzugefügt.
Bei Fragen wenden Sie sich bitte an matthias.feurer@stat.uni-muenchen.de
- معلم: Giuseppe Casalicchio
- معلم: Matthias Feurer