Key: BigDataSocialScience
Instructors
Frauke Kreuter frauke.kreuter@stat.uni-muenchen.de
Please contact Carolina Haensch (anna-carolina.haensch@stat.uni-muenchen.de) if you have any questions.
Learning outcome
Learn how to think about data analysis to solve social problems using and combining large quantities of heterogeneous data from a variety of different sources. Learn how to evaluate which data are appropriate to a given research question and statistical need. Learn the different data quality frameworks and learn how to apply them. Learn the basic computational skills required for data analytics (for text-mining, large-scale data integration and visualization), typically not taught in social science, economics, statistics or survey courses. Learn how to apply statistical and data quality frameworks to big data problems.
Organization
This is a block course (meetings on 6 days over Zoom), the course language is English.
The course will consist of recorded lectures, prepared materials like course notebooks with exercises and a course project as well as meetings on six days in September 2021 (probably 13.-17.9 and 20.9.).
Can count towards:
WP 16 Advanced Methods in Social Statistics and Social Data Science
WP 39 Computational Social Science
Enrollment Key: BigDataSocialScience
- Trainer/in: Anna-Carolina Haensch
- Trainer/in: Christoph Kern
- Trainer/in: Frauke Kreuter
- Trainer/in: Frauke Kreuter
Person: Dr. Cornelia Oberhauser
SAS-Kurs als 5-tägiger Blockkurs in den Semesterferien
Termine:
Tag |
Uhrzeit |
Raum |
|
---|---|---|---|
Mo 20.09.2021 |
Vorlesung | 9:15 - ca. 12:15 |
online über Zoom |
Übung |
13:15 - 17:00 |
online über Zoom |
|
Di 21.09.2021 |
Vorlesung |
9:15 - ca. 12:15 | online über Zoom |
Übung | 13:15 - 17:00 | online über Zoom | |
Do 23.09.2021 |
Vorlesung | 9:15 - ca. 12:15 | online über Zoom |
Übung | 13:15 - 17:00 | online über Zoom | |
Mo 27.09.2021 |
Vorlesung | 9:15 - ca. 12:15 | online über Zoom |
Übung | 13:15 - 17:00 | online über Zoom | |
Di 28.09.2021 |
Vorlesung | 9:15 - ca. 12:15 | online über Zoom |
Übung | 13:15 - 17:00 | online über Zoom |
Gastschlüssel
- Der Gastschlüssel lautet: "saskurs2021"
- Trainer/in: Cornelia Oberhauser
4-day block course in the semester break
Meetings:
Day | Time | Room | |
Mo 16.08.2021 | Lecture | 9:00 - 12:00 | online via Zoom |
Lecture | 14:00 - 17:00 | online via Zoom | |
Tu 17.08.2021 | Lecture | 9:00 - 12:00 | online via Zoom |
Lecture | 14:00 - 17:00 | online via Zoom | |
We 18.08.2021 | Lecture | 9:00 - 12:00 | online via Zoom |
Lecture | 14:00 - 17:00 | online via Zoom | |
Th 19.08.2021 | Lecture | 9:00 - 12:00 | online via Zoom |
Lecture | 14:00 - 17:00 | online via Zoom |
Course summary: The social survey is a research tool of fundamental importance across a range of disciplines and is widely used in applied research and as evidence to inform policy making. This course considers the process of conducting a survey, with an emphasis on practical aspects of survey design and implementation, as well as estimation. The course will introduce students to the basic principles of survey design that are used in large-scale surveys. The course will also provide an introduction to key elements of conducting a survey, including procedures in sample design, alternative modes of data collection, questionnaire design, sources of error, and key statistical concepts in estimation.
This is a block course (four days), the course language is English.
Registration key / Einschreibeschlüssel: SDE2021LMU
- Trainer/in: Joseph Sakshaug
Kurs | Zeit | Ort |
---|---|---|
Grundkurs | Di, 01.06., 16.00 - 19.30 | online |
Grundkurs | Di, 08.06., 16.00 - 19.30 | online |
Grundkurs | Di, 15.06., 16.00 - 19.30 | online |
Aufbaukurs | Di, 22.06., 16.00 - 19.30 | online |
Aufbaukurs | Di, 29.06., 16.00 - 19.30 | online |
- Trainer/in: Alexander Bauer
- Trainer/in: Felix Langer
- Trainer/in: Juliana Schäfer
- Trainer/in: Daniel Schlichting
- Trainer/in: Karl Schulte
- Trainer/in: Maximilian Weigert
Description: Machine Learning (ML) is increasingly used to guide high-stakes decisions in various contexts such as college admissions, granting loans or hiring employees. By eliminating human judgment, ML-based decision-making promises to be neutral and objective and to find the right decisions in shorter time. At the same time, however, concerns are raised that algorithmic decision-making may foster discrimination and amplify existing biases that are fed into the models. This course discusses recent advances in the field of Fair ML: How can we measure algorithmic fairness? How can we mitigate biases and make ML models fair(er) and interpretable? A key aspect of the course will be linking fairness definitions and correction techniques with real world examples of automated decision-making.
Key: FairADM21
- Trainer/in: Christoph Kern
- Trainer/in: Frauke Kreuter
Die Entscheidungstheorie befasst sich mit rationalen Entscheidungen unter Unsicherheit. Sie besitzt große interdisziplinäre Bedeutung etwa bei der Analyse und Unterstützung von Entscheidungen in der BWL (z.B. Investmentstrategien), VWL bzw. Soziologie (Rational Choice Theorie), Medizin (z.B. Expertensysteme) oder den Ingenieurwissenschaften (z.B. autonome Steuerung). Die statistische Entscheidungstheorie bietet zudem einen formalen Rahmen für die Wahl von Analysemethoden (optimale Tests oder Schätzer, beste Klassifikationsverfahren etc.); sie spielt eine fundamentale Rolle bei der kritischen Analyse und problemadäquaten Verallgemeinerung datenbasierter Lernverfahren.
Die Veranstaltung diskutiert zunächst die allgemeine Struktur von Entscheidungsproblemen inklusive fundamentaler Entscheidungsprinzipien. Dann analysiert und charakterisiert sie das Bayes- und das Minimaxkriterium als Extrempole, mit (Zustands-)Unsicherheit umzugehen, und entwickelt moderne Alternativen im Kontext komplexer Unsicherheit (Ambiguität). Im zweiten
Teil wird ein Überblick über weitere Themengebiete gegeben, der auch an die aktuelle Forschung im
Bereich der Entscheidungstheorie heranführt.
NEU:
In diesem Semester haben Sie erstmals zwei Möglichkeiten, ECTS-Punkte
zu erwerben. Sie können sich ausschließlich über den ersten Teil der
Veranstaltung prüfen lassen (Umfang etwa 2/3 der Vorlesungszeit) oder
eine Prüfung über beide Teile ablegen. In beiden Fällen erhalten Sie 6
ECTS-Punkte für das Modul "Entscheidungstheorie" aus den
Masterprüfungsordnungen, im zweiten darüberhinaus noch
3 weitere, separat benotete, ECTS-Punkte, die Sie flexibel einbringen können.
Wenn Sie sich begründet nachmelden wollen bitte Mail an dominik.kreiss@stat.uni-munchen.de
- Trainer/in: Thomas Augustin
- Trainer/in: Christoph Jansen
- Trainer/in: Dominik Kreiß
Termine und Personen:
Einschreibeschlüssel: LiMo_2021
- Trainer/in: Helmut Küchenhoff
- Trainer/in: Felix Langer
- Trainer/in: Martje Rave
Code für Selbsteinschreibung: SemGraf_21
- Trainer/in: André Klima
- Trainer/in: Georg Schollmeyer
Schlüssel für Selbsteinschreibung: Stat2_SoSe21
- Trainer/in: Hannah Blocher
- Trainer/in: André Klima
- Trainer/in: Helmut Küchenhoff
- Trainer/in: Giacomo De Nicola
- Trainer/in: Cornelius Fritz
Termine
Termin | Ort | |||||
---|---|---|---|---|---|---|
Vorlesung | Montag | 14 - 16 | Online |
als Q&A über Zoom |
||
Übung |
Dienstag |
10 - 12 | Online |
Live über Zoom |
- Der Einschreibeschlüssel lautet: ST2_21
- Trainer/in: Cornelius Fritz
- Trainer/in: Christian Heumann
- Trainer/in: Christopher Küster
- Trainer/in: Volker Schmid
- Trainer/in: Christoph Berninger
- Trainer/in: Robert Czudaj
- Trainer/in: Philipp Schiele
Description:
Basic concepts of the analysis of longitudinal data
are covered. Marginal
models as well as mixed effects (also known as random effects) models
for both Gaussian and discrete response variables are discussed.
In the lab, the students are encouraged to apply the lecture contents to
real data in order to deepen the understanding of the discussed
concepts and to become more familiar with the learned methods and
techniques.
Organization and Dates:
Flipped Classroom with Q&As via zoom every
Tuesday 8.30 - 10.00; Thursday 14.15 - 15.45
Enrolment Key: Longi2021!
Target Audience:
Statistics Master students, recommended prerequisites:
- Lecture "Lineare Modelle"
- Lecture "Generalisierte Regression"
- Basic knowledge in R
Examination:
15 Minutes oral exams
- Trainer/in: Giacomo De Nicola
- Trainer/in: Fabian Scheipl
Einschreibeschlüssel: MathErg21!
- Trainer/in: Annika Hoyer
- Trainer/in: Sevag Kevork
- Trainer/in: Antonio Melieni
Description
The lecture deals with theoretical and practical concepts from the fields of statistical learning and machine learning. The main focus is on predictive modeling / supervised learning. The tutorial applies these concepts and methods to real examples for illustration purposes.
Organization and Dates
Due to Corona, all lectures and exercises will be online!
Enrolment Key
FCIMPM21
Target Audience
- Statistics (Methods/Bio/WISO)
- Data Science MSc.
- Trainer/in: Bernd Bischl
- Trainer/in: Julia Herbinger
- Trainer/in: Julia Moosbauer
- Trainer/in: David Rügamer
Die Veranstaltungen EMOS A und EMOS B geben aus einer methodischen Perspektive einen Überblick über zentrale Konzepte der amtlichen Statistik. Besprochen werden in EMOS B u.a. folgende Themen: nationale und internationale Armutsmessung, dynamische Indikatoren der Wirtschaftsstatistik, grundlegende Konzepte und Methoden der Bevölkerungsstatistik/Demographie, spezielle Bereichsstatistiken (Haushalts-, Todesursachen- und Unternehmensstatistiken), Linkage und Matching von Datensätzen.
Diese Veranstaltung ist eine Pflichtveranstaltung für alle
Studierenden, die das EMOS-Zusatzzertifikat (European Master in Official
Statistics) erwerben wollen; alle anderen Masterstudierenden können
sich 6 ECTS-Punkte flexibel anerkennen lassen. Ein vorhergegangener Besuch von EMOS A (immer im Winteresemester
angeboten) ist nicht notwendig.
- Trainer/in: Thomas Augustin
- Trainer/in: Dominik Kreiß
Die Veranstaltung "Statistische Software (R)" wendet sich an Studierende im Bachelorstudiengang Statistik (2. Semester).
- Trainer/in: Andreas Bender
- Trainer/in: Philipp Kopper
Kurzübersicht:
- Vorlesung: Montag 12-14 Uhr und Dienstag 10-12 Uhr
- Übung: Donnerstag 10-12 Uhr und Donnerstag 12-14 Uhr
- Tutorium: Montag xx-xx Uhr und Dienstag 12-14 Uhr
Einschreibeschlüssel: "multi_ss_21"
- Trainer/in: Annika Hoyer
- Trainer/in: Marc Schneble
- Trainer/in: Dina Voeltz
Die Veranstaltung "Programmieren mit Statistischer Software (R)" wendet sich an Studierende im Bachelor Statistik (4. Semester). Sie baut auf den Veranstaltungen "Einführung in die Statistische Software" (1. Semester) und "Statistische Software" (2. Semester) auf.
Die Veranstaltung findet vom 12.04.2021 ausschließlich online statt und verläuft nach dem Inverted Classroom Prinzip.
Einschreibeschlüssel: progr2021
Wichtige Aspekte des Kurses:
- Dieser Kurs ist vollständig online, es gibt keine Präsenzvorträge oder -übungen.
- Es ist absolut unerlässlich, dass Sie R zu Hause auf Ihrem Gerät zum Laufen bringen, da wir nicht wissen, wann die CIP-Pools im Institut wieder geöffnet werden. Bitte benutzen Sie unsere Installationsanleitung, die Sie auf dieser Moodle-Seite finden.
- Um die 6 ECTS-Punkte für diesen Kurs zu erhalten, müssen Sie die Hausarbeit am Ende des Semesters bestehen.
- Die Buchkapitel, Links und weiteren Materialien sind alle optional. Wenn Sie jedoch jede Woche die Themen und Aufgaben abschließen und die Buchkapitel durcharbeiten, steigen Ihre Chancen den Kurs zu bestehen stark an.
- Wir werden jede Woche eine Zoom-Sitzungen abhalten, um Ihnen bei den Aufgaben und bei Fragen zu helfen.
- Sie sollen diesen Kurs im vierten Semester des Bachelor-Studiengangs Statistik abschließen. Die Master-Veranstaltung Fortgeschrittene Programmierung baut auf diesem Kurs auf.
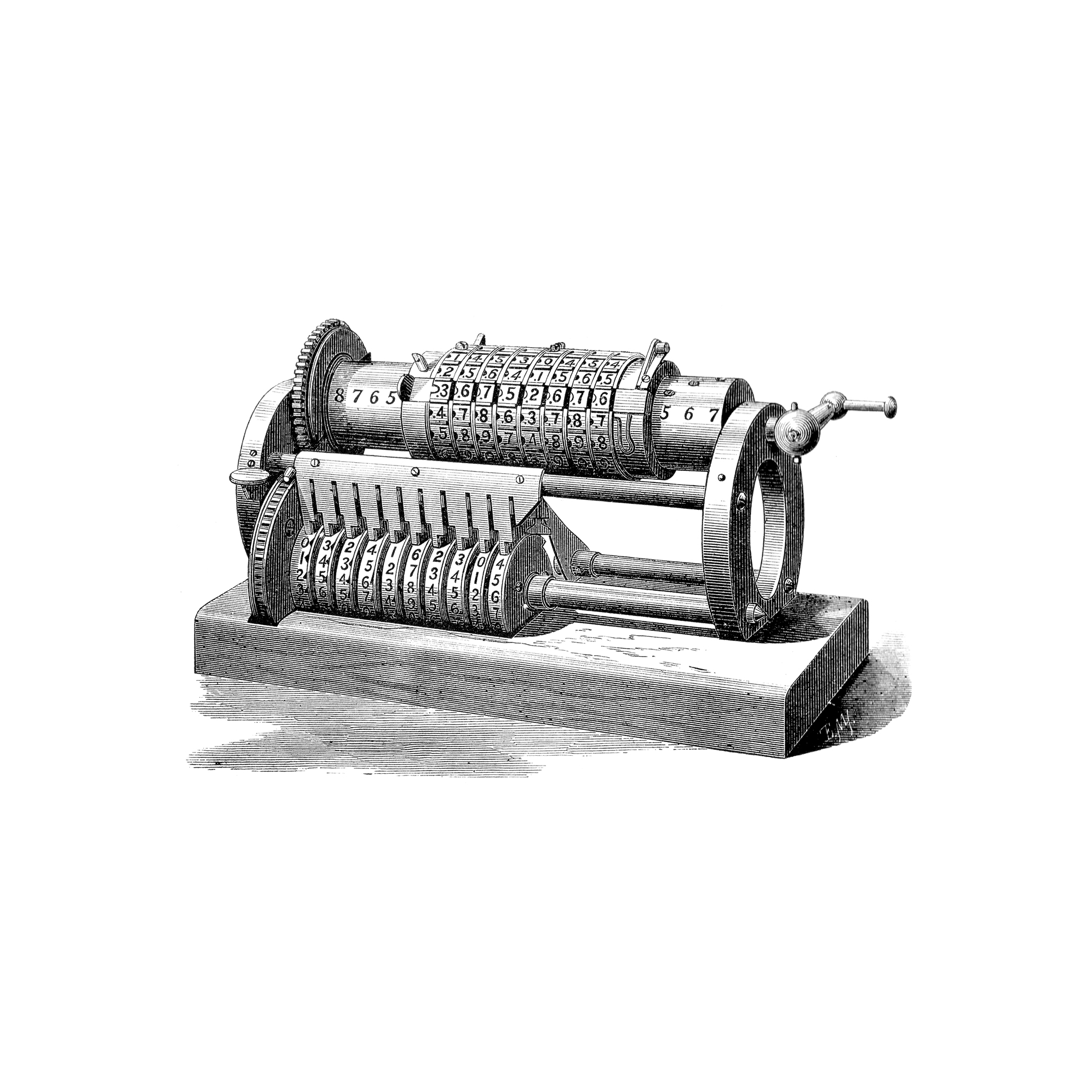
- Trainer/in: Andreas Bender
- Trainer/in: Martin Binder
- Trainer/in: Moritz Herrmann
- Trainer/in: Noah Hurmer
- Trainer/in: Philipp Kopper
- Trainer/in: Sven Lorenz
- Trainer/in: Julia Niebisch
Termine:
- Vorlesung: Montag 9:15 - 11:45 Uhr
- Übung: Mittwoch 8:15 - 9:45 Uhr
- Trainer/in: Anne-Laure Boulesteix
- Trainer/in: Annika Hoyer
- Trainer/in: Dina Voeltz
Einschreibeschlüssel: "stat4_nf"
- Trainer/in: Cornelia Fütterer
- Trainer/in: Malte Nalenz
- Trainer/in: Christian Müller
- Trainer/in: Roberto Olayo Alarcon
Formale Techniken und Argumentationen besitzen auch in den Sozial-,
Geistes- und Wirtschaftswissenschaften eine große, und immer weiter
wachsende,
Bedeutung, stellen aber für viele, darauf nicht so gut vorbereitete
Studierende eine sehr große Hürde dar. Im Rahmen eines
fachübergreifenden Propädeutikums
sollen nichtmathematikaffine Studierende der LMU sanft in die
Formalisierung eingeführt und mit wesentlichen Techniken (wieder)
vertraut gemacht werden.
Gerade unter den Herausforderungen der Corona-Situation ist es uns ein
besonderes Anliegen, nicht-mathematikaffine Studienanfän-ger*innen in
einem semesterbegleitenden Vorkurs zu begleiten und zu unterstützen.
Die Veranstaltung richtet sich sowohl an Studienanfänger als auch an Studierende die im Rahmen Ihres Studiums bereits auf Schwierigkeiten im Umgang mit formalen Methoden gestoßen sind.
Zielgruppe: Studierende der Geistes-, Sozial- und Wirtschaftswissenschaften
Einschreibeschlüssel (sowohl für Teilnehmer*innen, als auch für Gastzugang): CoVorMa
- Trainer/in: Thomas Augustin
- Trainer/in: Hannah Blocher
- Trainer/in: Cornelia Fütterer
- Trainer/in: Christoph Heindl
- Trainer/in: Dominik Kreiß
- Trainer/in: Malte Nalenz
- Trainer/in: Yusuf Sale
- Trainer/in: Georg Schollmeyer
VL: Marcel Neunhoeffer
Übung: Anna-Carolina Haensch
Tutorium: Christoph Heindl
Termine Vorlesung:
Mo. | 12:00 bis 14:00 c.t. |
Termine Übung (nur eine Übung muss besucht werden!):
Die Übung von Gruppe 1 startet am 20.4, die Übung von Gruppe 2 startet am 21.4
Di. 16:00 bis 18:00 c.t.
Mi. 14:00 bis 16:00 c.t.
Termine Tutorium:
Das Tutorium startet in der dritten Vorlesungswoche (29.04.).
Do. 10:00 bis 12:00 c.t.
Einschreibeschlüssel: Stat2Soz!2021
- Trainer/in: Anna-Carolina Haensch
- Trainer/in: Christoph Heindl
- Trainer/in: Marcel Neunhoeffer
Termine
- Vorlesung: Mittwoch, 12:00 - 14:00 Uhr,
- Übungen: Freitag, 8:00 - 10:00 Uhr
Einschreibeschlüssel
- Der Einschreibeschlüssel lautet "oek_s21".
- Trainer/in: Robert Czudaj
- Trainer/in: Philipp Schiele
- Der Einschreibeschlüssel ist StoSta22
- Vorlesungen sind als aufgezeichnete Videos bei LMUcast (s.u.) verfügbar, jede Woche gibt es eine Online-Fragestunde am Dienstag. Multiple Choice Quizzes zur Selbstkontrolle auf moodle.
- Für die Übungen gibt es Musterlösungen als PDF, aufgezeichnete Erklärvideos dazu und Online-Fragestunden zur Übung in 3 Gruppen jeden Mittwoch und Donnerstag.
Termin & Ort
Vorlesung:
Di 12-14 , Do 12-14 @ M010 (Hauptgebäude)
Übung/Fragestunde:
Do 14-16, Mi 12-14, Mi 14-16
- Trainer/in: Catharina Hollauer
- Trainer/in: Fabian Scheipl
Einschreibeschlüssel: ---
- Trainer/in: Christoph Berninger
- Trainer/in: Michael Kobl
- Trainer/in: Dominik Kreiß
- Trainer/in: Henry Port
- Trainer/in: Benjamin Sischka
Dates & Overview
Kickoff: April, 16th of April, 11:00 am
Block: Friday, 25th of June, 1:00 pm-4:00 pm
Key: Seminar_DUL
Target groups: Master Statistics, Master Data Science
Course Description
Deep learning algorithms have made outstanding results in many domains such as computer vision (CV), natural language processing (NLP), recommendation systems, and medical image analysis. However, the outcome of current methods depends on a huge amount of training labeled data, and in many real-world problems such as medical image analysis and autonomous driving, it is not possible to create such an amount of training data. Learning from unlabeled data can reduce the deployment cost/time of deep learning algorithms where it requires annotations from experts such as medical professionals and doctors.
In this course, we will learn about the theory of deep unsupervised learning and will review some state-of-the-art methods. We will offer different topics with different applications (i.e. NLP, CV) for a variety of tasks (i.e. clustering, representation learning, density modeling, etc). As part of the seminar, you will also apply one of the frameworks to a given real-world problem. This means every participant will be asked to prepare an oral presentation about a current technique and to write up a reproducible case study of actual data analysis in an unsupervised DL framework, in addition to peer-reviewing the (theoretical and practical) work of a colleague.
Recommended prerequisites: Deep learning; Python, PyTorch, TensorFlow, We would also hold the seminar in English and also allow students from other courses (especially DS students)
Preliminary meeting:
April 16th, 11:00 - 12:30
Virtual Room:
Seminar: Blocked towards the end of the semester (1-2 units)
- Trainer/in: Mina Rezaei
- Datum: 30.08.2021 - 03.09.2021
- Zeit: tbd
- Ort: tbd
- Dozent: Dr. Ludwig Bothmann (E-Mail: ludwig.bothmann@stat.uni-muenchen.de)
- Vorkenntnisse: Statistik I - IV, Multivariate Verfahren, Generalisierte Regressionsmodelle, I2ML, Grundlegende Programmierkenntnisse, idealerweise in R. Es werden keine Python-Kenntnisse oder Kenntnisse in Deep Learning vorausgesetzt.
Wichtig: Sie müssen das Anmeldeformular auf der Moodle-Seite ausfüllen um einen Platz für den Kurs zu bekommen, die Selbsteinschreibung mit dem Einschreibeschlüssel reicht nicht.
- Trainer/in: Ludwig Bothmann
- Trainer/in: Omid Charrakh
- Trainer/in: Mina Rezaei
- Trainer/in: Tobias Weber
- Nebenfach Statistik
- Bachelor Statistik
Einschreibeschlüssel: Invers
- Trainer/in: Volker Schmid
Entropy is defined as a measurable physical property that is most commonly associated with a state of disorder, randomness, or uncertainty. It is strongly connected with probability distributions and the principle of maximum entropy can be very useful in statistical inference, in particular in Bayes statistics. In this course we will introduce the concept of entropy in the context of information theory as well as apply the concepts on real data sets.
Syllabus
- Introduction and Preview
- Entropy, Relative Entropy, and Mutual Information
- Asymptotic Equipartition Property
- Entropy Rates of a Stochastic Process
- Differential Entropy
- Information Theory and Statistics
Target audience:
- Advanced Bachelor Statistics (e.g. "Ausgewählte Gebiete der angewandten Statistik B", 3 ECTS)
- Master Statistics, Biostatistics, Statistics (WiSO), (e.g. "Ausgewählte Gebiete der ... Statistik B", 3 ECTS)
- ESG Data Science (3 ECTS Elective Course)
Date and Time: April 16th to May 28th (!), Friday 2pm to 6pm
Exam: Midterm & Final Exams (Written Exams)
The course will be held online in English.
Code for course enrollment: Information
- Trainer/in: Zahra Aminifarsani
- Trainer/in: Volker Schmid
Syllabus
- Introduction to Stochastic Processes
- Autoregressive Moving Average Processes
- Estimation of Vector ARMA Models
- Prediction
- Testing for Causality
- Innovations Accounting
- Structural VAR
Intended audience: Advanced students and PhD students in econometrics, statistics, VWL, BWL, mathematics or computer science.
Prerequisites: Profound knowledge in matrix-algebra and econometrics (econometrics I) or statistics (linear models). Basic knowledge in univariate time series analysis is not demanded but of advantage.
Teaching Style: Online via Zoom and Moodle
Examination: (written) Exam
Record of Achievement: 3 ECTS + 3 ECTS
Time Schedule
- Trainer/in: Robert Czudaj
- Trainer/in: Dennis Mao
- Trainer/in: Nurzhan Sapargali
Syllabus:
- Overview
- Fundamentals and Properties of Stochastic Processes
- Univariate ARIMA-Processes
- Estimation and Forecasting of ARIMA-Models
- Univariate GARCH-Models + Extensions
- Selected aspects: Long Memory und Fractional Differencing, Threshold-Models
Intended audience: Advanced bachelor and master students of statistics, mathematics, computer science (Informatik), economics and business administration.
Prerequisites: Solid mathematical foundations (analysis and linear algebra), basic knowledge in econometrics (econometrics 1) or statistics (linear models).
Teaching Style: Online via Zoom and Moodle in english
Examination: (written) Exam
Record of Achievement: 6 ECTS
Time Schedule
The lectures and tutorials take place between 12.04.2021 (first lecture) and 18.05.2021.
- Trainer/in: Dennis Mao
Dates & Overview
Kickoff: Wednesday, 14th of April, 5pm
Block 1: Friday, 28th of May, 1pm-4pm
Block 2: Friday, 25th of June, 1pm-4pm
Key: will be announced at kickoff event
Target groups: Master Statistics, Master Data Science
Course Description
Classical
statistical learning excels at learning associations and making
accurate predictions. However, many questions like treatment effect
estimation are not associative but causal in nature.
Graphical models
provide a general framework for describing statistical relations
between random variables and performing inference. More recently, they
have become a popular framework for reasoning about causal relations.
In
this seminar, we will introduce the basics of inference in graphical
models, discuss how they can be employed to perform causal inference and
peak into recent research on connections to classical machine learning and interpretable machine learning.
- Trainer/in: Gunnar König
Description
The course is a practical introduction into TensorFlow and PyTorch as a software with their practical application in statistics and deep learning. The course covers
- basic operations
- (probabilistic) statistical models
- multilayer feedforward neural networks
- convolutional neural networks
- recurrent neural networks
- autoencoder
in TensorFlow and PyTorch.
Target audience
Statistics and Data Science Master students
Enrollment Key
appliedDL2021!
- Trainer/in: Hüseyin Gündüz
- Trainer/in: Mina Rezaei
- Trainer/in: David Rügamer
- Master Statistik (Pflicht)
- Master Biostatistik, WiSo (Wahl)
- Master ESG Data Science (elective)
- Sie bekommen wöchentlich Videos, Übungsaufgaben und weitere Materialien zu den Inhalten, die Sie zu Hause bearbeiten.
- Wir treffen uns regelmäßig (in Zoom) zur Besprechung der Inhalte und zur Klärung von Fragen.
- Wichtige Übungsaufgaben werden gemeinsam online besprochen.
The course is held in the form of an "inverted classroom":
- You will receive weekly videos, exercises and other materials on the contents, which you will work on at home.
- We meet regularly (in Zoom) to discuss the contents and answer questions.
- Important exercises will be discussed together online.
- Einführungsveranstaltung / Introductory meeting: 12. April 12–14 Uhr
- Fragestunde / Question time jeden: Donnerstag / every Thursday 10–12
- Übungen / Exercises: jeden Montag / every Monday 12–14
Attention: This course is partially in German and partially in English!
Achtung: Diese Vorlesung wird teilweise auf Englisch und teilweise auf Deutsch abgehalten!
- Vorlesungsvideos und Fragestunde auf Deutsch
- Question time in English on request
- Exercises/Tutorials in English
Einschreibeschlüssel / Enrolment code: Markov
- Trainer/in: Nurzhan Sapargali
- Trainer/in: Volker Schmid
Important Dates (all mandatory):
Preliminary meeting | 06.04.21, 16-18 c.t. | Zoom |
Additional Presentations | 26.04., 17.05., 14.06. (16 c.t.) | Zoom |
Presentations | 08./09.07.21, 10-15 s.t. | Zoom |
Submission deadline | 31.08.21, 23.59 CET |
Enrollment key
Will be announced at the preliminary meeting.
Note
This seminar is subject to the regular application process for seminars at the Department of Statistics.- Trainer/in: Matthias Aßenmacher
- Trainer/in: Christian Heumann
- Trainer/in: Daniel Schalk
Termine
- Vorlesung: Dienstag, 16 - 18 c.t., Prof. Dr. Christian Heumann
- Übungen (Statistik II):
Mittwoch, 12 - 14 c.t. (2x) & 14 - 16 c.t. (2x)
Donnerstag, 18 - 20 c.t.
Freitag, 10 - 12 c.t. - Übung (Statistik I):
Montag, 10 - 12 c.t.
Einschreibeschlüssel
- Der Einschreibeschlüssel lautet "wiwistat21".
- Trainer/in: Matthias Aßenmacher
- Trainer/in: Lukas Beise
- Trainer/in: Christian Heumann
- Trainer/in: Katrin Racic-Rachinsky
- Trainer/in: Nora Valiente Bauer
- Trainer/in: Simon Wiegrebe