Blockkurs Feb 27 - March 3, 2023
Enrolment key: fairML23
- Trainer/in: Christoph Kern
Kurs | Zeit | Ort |
---|---|---|
Grundkurs | Mo, 27.02., 10.00 - 17.00 Uhr | Ludwigstraße 28, IuK-Pool (207) |
Grundkurs | Di, 28.02., 10.00 - 17.00 Uhr | Ludwigstraße 28, IuK-Pool (207) |
Grundkurs | Mi, 01.03., 10.00 - 17.00 Uhr | Ludwigstraße 28, IuK-Pool (207) |
Aufbaukurs | Do, 02.03., 10.00 - 17.00 Uhr | Ludwigstraße 28, IuK-Pool (207) |
Aufbaukurs | Fr, 03.03., 10.00 - 17.00 Uhr | Ludwigstraße 28, IuK-Pool (207) |
- Trainer/in: Helen Alber
- Trainer/in: Theresa Meier
- Trainer/in: Daniel Schlichting
- Trainer/in: Maximilian Weigert
- Trainer/in: Simon Wiegrebe
- Wednesdays, 10:15-12:00; Schellingstr. 3(R), R 051
- Thursdays, 16:15-18:00; Schellingstr. 3(S), S 006.
- Trainer/in: Tomasz Olma
Enrolment token: csds22/23
More information soon.
- Trainer/in: Jacob Beck
- Trainer/in: Frauke Kreuter
- Trainer/in: Frauke Kreuter
- Trainer/in: Joseph Sakshaug
Schedule:
date/time | location | instructor | |
---|---|---|---|
Initial meeting | in Nov (date tbd) | tbd | Wilhelm |
Presentations | beginning of Feb (date tbd) | tbd | Wilhelm |
- Trainer/in: Daniel Wilhelm
Schedule:
day/time | location | instructor | start date | |
---|---|---|---|---|
Lecture | Mon, 16:15-18:00 | Schellingstr. 3 (S) / S 004 | Wilhelm | 17.10.22 |
Lecture/Tutorial | Wed, 8:15-10:00 | Geschw.-Scholl-Pl. 1 (E) / E 006 | Wilhelm | 19.10.22 |
Enrolment key: ci2223
- Trainer/in: Philipp Schiele
- Trainer/in: Daniel Wilhelm
- Trainer/in: Anna-Carolina Haensch
- Trainer/in: Frauke Kreuter
- Trainer/in: Frauke Kreuter
- Trainer/in: Dennis Mao
- Trainer/in: Philipp Schiele
Introductory meeting of the seminar
- Trainer/in: Cornelius Fritz
- Trainer/in: Thomas Nagler
- Trainer/in: Julian Rodemann
Sie können sich in den Kurs mit dem Schlüssel DHS2223 selbst einschreiben.
- Trainer/in: Florian Fleischmann
- Trainer/in: Helmut Küchenhoff
- Trainer/in: Valentin Weber
Description
We offer a dozen implementation challenges in TensorFlow and Pytorch that you will work on during the course of the semester with extensive supervision by an expert. The goal is to learn one of the two major deep learning languages with hands-on experience and implement an advanced deep learning project.
Organization
- Kick-off Session (Zoom): 24.10.2022, 5pm
- Final Submission Deadline: End of the semester
Enrolment Key
appliedDL2223
Target Audience
- Statistics
- Data Science
- 6 ECTS (e.g., for the module Advanced Deep Learning)
- Trainer/in: Emilio Dorigatti
- Trainer/in: Chris Kolb
- Trainer/in: Mina Rezaei
- Trainer/in: David Rügamer
- Trainer/in: Tobias Weber
Der Einschreibeschlüssel lautet: DT2223
The enrollment key is: DT2223
- Trainer/in: Christoph Jansen
Official statistical agencies are the central information service providers in a democratically organized society, informing politics, business and society about current economic, social and increasingly also ecological developments. In this way, official statistics form an important basis for informed decisions but at the same time act as a control authority, especially for politics, by empirically reflecting the consequences of decisions and actions. To meet this responsibility, official statistics production underlies a strict methodology and high-quality standards. Against this background, there has also been an intensive discussion about the opportunities and challenges complex statistical modelling, machine learning techniques and new data sources deliver. This development has also led to close cooperation of statistical authorities with a number of universities, resulting in an EU-wide certification of particular master's degree programmes (EMOS: European Master in Official Statistics). For students at LMU, it is possible to obtain an EMOS certificate by taking a specific route within the master's program specialisations in machine learning and social statistics and data science.
The course "EMOS A" aims to prepare students for participation in the discourse between academic and official statistics and the numerous opportunities for cooperation with public data producers. It provides an insight into typical questions and modes of argumentation, methodological principles, and the most important products of official statistics. First, we take a look at current research concerning official statistics. Then, the basic principles and legal framework of official statistics, their fundamental structure in Germany/Europe and their most important supporting institutions are discussed as a basis. Further topics will then include the EU system of environmental indicators, a discussion of the role of Big Data in official statistics, selected aspects of result dissemination and statistical literacy, and access to official data sources for (own) secondary analyses, including an introduction to statistical anonymization techniques.
The
format of the course is a mix of inverted classroom elements with
online or in-person discussion and deepening of the topics and guest
lectures. In
addition, there is an independent examination of the material in the
form of short mini-projects as exercises, in which specific content is
worked through and then discussed. Grade bonuses are possible.
The course is compulsory for the EMOS variant; all other students can acquire 6 ECTS credits, which can be used flexibly.
Enrollment Key: emosa
- Trainer/in: Thomas Augustin
- Trainer/in: Dominik Kreiß
Syllabus
ONLINE COURSE
Teachers: Hanna Brenzel, Hariolf Merkle, Marco Puts, Piet Daas
Runtime: 14. November 2022 - 16. December 2022
Format: Flipped Classroom: Self-learning through online videos and literature, weekly 1-hour online meetings
Examination: Examination sheets (3 ECTS credits)
Language: English
Prerequisites: Basic R knowledge is required.
Who is this course for? MsC Statistics and Data Science (2021, WP 28+29+40+46), BsC Statistics and Data Science (2021, WP 8+11),Bsc Statistik (2010, WP 6.0.3+6.0.4), Statistik und Data Science als Nebenfach für Bachelor 30 ECTS (2021, WP 4+5), Statistik und Data Science als Nebenfach für Bachelor 60 ECTS (2021, WP 11+12), Statistik und Data Science als Nebenfach 30 ECTS Mathematik (2021, WP 5+6), Statistik Nebenfach 60 ECTS Bachelor Soziologie (2021, WP 10+11), Grundlegende Statistik als Nebenfach für MA 30 ECTS (2011, WP 6), Vertiefte Statistik Master (2011, WP 5)
- Trainer/in: Hanna Brenzel
- Trainer/in: Piet Daas
- Trainer/in: Anna-Carolina Haensch
- Trainer/in: Markus Herklotz
- Trainer/in: Marco Puts
Termin | Ort | Person | Beginn | |
---|---|---|---|---|
Vorlesung | Di, 12:15-13:45 | TBA | Nagler | 18.10.22 |
Übung | Mi, 12:15-13:45 | TBA | Nagler | 26.10.22 |
Einschreibeschlüssel
- Der Einschreibeschlüssel lautet: "fmm"
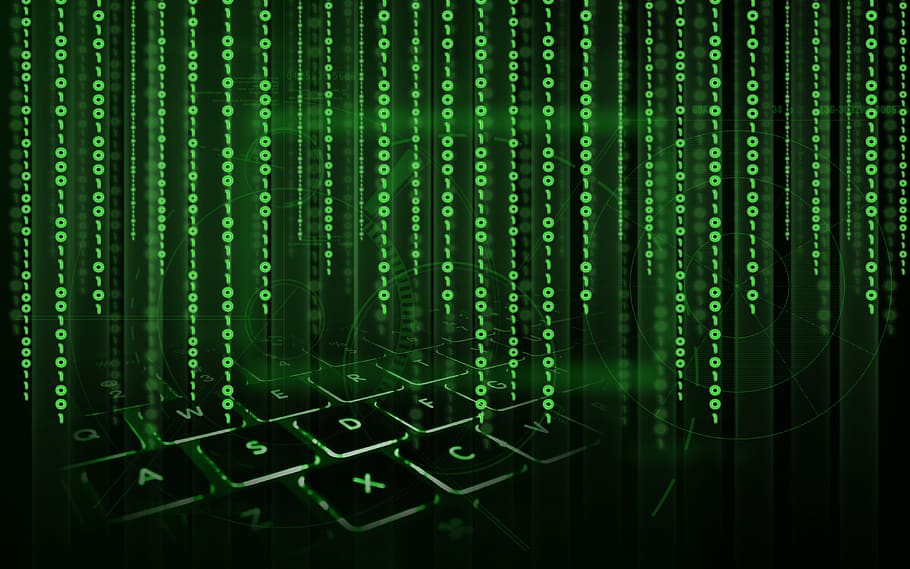
- Trainer/in: Thomas Nagler
- Trainer/in: Mario Teixeira Parente
Dieser Kurs wird Studierenden mit NF Statistik eine Einführung in die statistische Software (R) geben.
Der Kurs setzt sich aus einer Mischung aus Flipped-Classroom Lehrvideos, Hands-On Lab-Sessions und Drop-In Hilfe-/Beratungssession zusammen.
Weitere Infos folgen in Kürze.
Sie müssen und können sich NICHT
über das lsf für die Veranstaltung anmelden, schreiben Sie sich bitte
einfach mit dem Einschreibeschlüssel FortStat1WS22 in diesen Moodle Kurs ein.
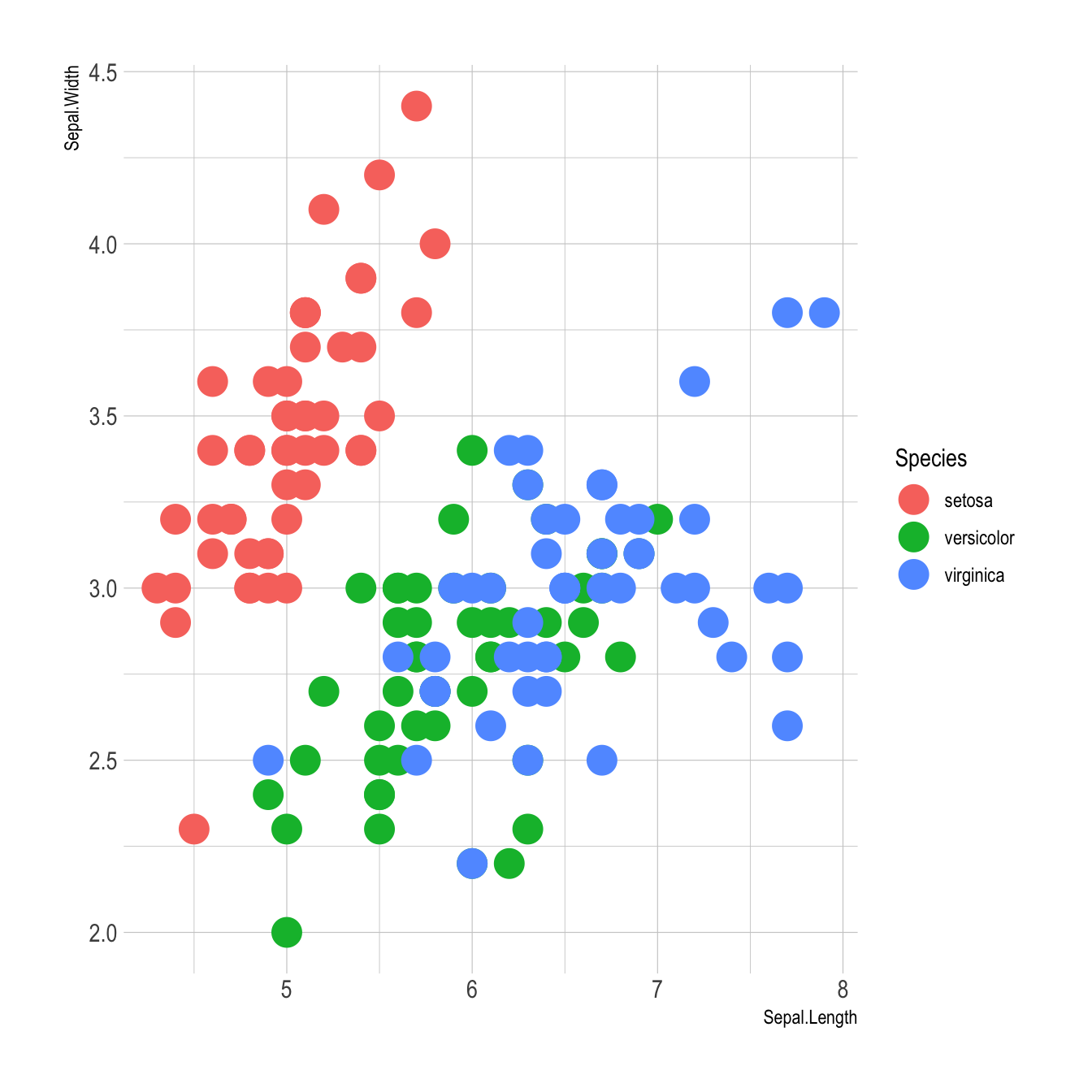
- Trainer/in: Jacob Beck
- Trainer/in: Anna-Carolina Haensch
- Trainer/in: Felix Henninger
- Trainer/in: Markus Herklotz
- Trainer/in: Jan Simson
- Der Einschreibeschlüssel lautet: "stat3nf2022"
- Trainer/in: Malte Schierholz
- Trainer/in: Ruben Camilo Wißkott
Inverted classroom style. Weekly meeting (in person): Wednesday, 14:00-16:00 c.t. Seminar Room
Starting date: 19.10.2022
Enrollment Key: automl23
- Trainer/in: Lennart Schneider
- Trainer/in: Janek Thomas
Termine und Personen:
Termin | Ort | Person | |
---|---|---|---|
Vorlesung |
Mo, 12.00 - 14.00 |
M018 |
Scheipl |
Vorlesung |
Mi, 10.00 - 12.00 |
M018 |
Scheipl |
Übung |
Mo, 14.00 - 16.00 |
S002 (Schelling 3) |
Rave |
Übung |
Mo, 16.00 - 18.00 |
S002 (Schelling 3) |
Gruber |
Tutorium |
Di, 10.00 - 12.00 |
A214 |
NN |
Tutorium |
Do, 14.00 - 16.00 |
B006 |
Sapargali |
Themen
- Das multiple lineare Regressionsmodell
- Statistische Regularisierung
- Das verallgemeinerte lineare Modell
- Gemischte Modelle
- Das generalisierte additive Modell
- GAMLSS, Quantilregression, Verteilungsregression
- Kategoriale Regression
- Lebensdauermodelle
- Messfehler, Fehlklassifikation und fehlende Daten
- Trainer/in: Cornelia Gruber
- Trainer/in: Martje Rave
- Trainer/in: Nurzhan Sapargali
- Trainer/in: Fabian Scheipl
Schedule:
- Lecture: Monday, 16-18 c.t., Geschw.-Scholl-Pl. 1 - A 017 (starting Monday, 17 October 2022)
- Lecture/Exercise: Wednesday, 10-12 c.t., Geschw.-Scholl-Pl. 1 - A 016 (starting Wednesday, 26 October 2022)
Enrollment key:
life22Time
- Trainer/in: Andreas Bender
- Trainer/in: Simon Wiegrebe
Einschreibeschlüssel: dskrpt
Termine:
Termin | Ort | Person | |
---|---|---|---|
Vorlesung |
Do, 14.00 - 16.00 |
M118 (Hauptgebäude) |
Fabian Scheipl |
Vorlesung |
Fr, 10.00 - 12.00 | M118 (Hauptgebäude) |
Fabian Scheipl |
Übung 1 |
Mo, 10.00 - 12.00 |
S004 (Schellingstr. 3) |
Eugen Gorich |
Übung 2 |
Do, 12.00 - 14.00 | B106 (Hauptgebäude) |
Michael Kobl |
Tutorium |
Di, 16.00 - 18.00 |
S001 (Schellingstr. 3) | Michael Kobl |
Übung & Tutorium beginnen erst in der zweiten Semesterwoche.
Diese Veranstaltung vermittelt elementare Wahrscheinlichkeitsrechnung sowie Grundlagen der deskriptiven und explorativen Statistik. Dies umfasst grundlegende Axiome und Rechenregeln für Wahrscheinlichkeiten (auch: bedingte und gemeinsame Wahrscheinlichkeiten) sowie die Begriffe der stochastischen und empirischen Unabhängigkeit für Ereignisse und Zufallsvariablen bzw. Merkmale. Die Lerninhalte umfassen auch eine erste einfache Begriffsbildung für und Eigenschaften von Zufallsvariablen, ihrer Wahrscheinlichkeitsdichten und Momente und wichtige parametrischer Verteilungsmodelle. Auf empirischer Seite werden entsprechend Skalenniveaus beobachteter Merkmale und einfache Erhebungsformen definiert und Techniken der uni- und multivariaten deskriptiven Statistik eingeübt: zum einen Datenvisualisierung anhand statistischer und wahrnehmungspsychologischer Leitlinien, zum anderen empirische Verteilungen und Kerndichten. Kennzahlen für Lage, Streuung, Schiefe, Wölbung, Konzentration und Assoziation werden eingeführt und ihre Eigenschaften intensiv diskutiert. Letzteres umfasst auch eine erste Einführung in die Probleme kausaler Interpretation von beobachteten Assoziationen.
Die Vorlesung entspricht Modul P3.1, die Übung dem Modul P4.1 des BA-Studiengangs Statistik und Data Science (PO 2021)
- Trainer/in: Michael Kobl
- Trainer/in: Fabian Scheipl
Der Einschreibeschlüssel lautet: MatheINF
- Trainer/in: Christoph Jansen
- Trainer/in: Georg Schollmeyer
Termine:
Termin | Ort | Person | Beginn | |
---|---|---|---|---|
Vorlesung | Di, 12:15-13:45 | Geschw.-Scholl-Pl. 1 (A) - A 120 | Hoffmann | 18.10.22 |
Vorlesung/Übung | Mi, 14:15-15:45 | Geschw.-Scholl-Pl. 1 (A) - A 120 | Rehms | 19.10.22 |
Einschreibeschlüssel
- Der Einschreibeschlüssel lautet: "EinfBiom2223"
- Trainer/in: Nicole Ellenbach
- Trainer/in: Sabine Hoffmann
- Trainer/in: Maximilian Mandl
- Trainer/in: Raphael Rehms
- Trainer/in: Theresa Ullmann
- Trainer/in: Theresa Ullmann
Date | Place | Person | Start | |
---|---|---|---|---|
Lecture | Thursday, 10:15-11:45 | Geschw.-Scholl-Pl. 1 - B 006 | Boulesteix/Hoffmann | 20.10.22 |
Enrolment key
- The enrolment key is: "ORS2223"
- Trainer/in: Anne-Laure Boulesteix
- Trainer/in: Sabine Hoffmann
- Trainer/in: Raphael Rehms
Date | Place | Person | Start | |
---|---|---|---|---|
Lecture | Tuesday, 16:00-17:45 | Geschw.-Scholl-Pl. 1 - A 015 | Schomaker/Hoffmann | 18.10.22 |
Lecture/ Exercise Session |
Thursday, 16:15-17:45 | Geschw.-Scholl-Pl. 1 - A 015 | Rehms | 27.10.22 |
Enrolment key
- The enrolment key is: "StatMetEpi2223"
- Trainer/in: Nicole Ellenbach
- Trainer/in: Sabine Hoffmann
- Trainer/in: Hannah Kümpel
- Trainer/in: Raphael Rehms
- Trainer/in: Michael Schomaker
- Trainer/in: Michael Schomaker
Date | Place | Person | Start | |
---|---|---|---|---|
Lecture | Tuesday, 9:15-11:45 | Geschw.-Scholl-Pl. 1 - A 014 | Boulesteix/Hoffmann | 18.10.22 |
Exercise Session | Monday, 14:15-15:45 | Geschw.-Scholl-Pl. 1 - A 120 | Rehms | 25.10.22 |
Enrolment key
- The enrolment key is: "PCS2223"
- Trainer/in: Anne-Laure Boulesteix
- Trainer/in: Sabine Hoffmann
- Trainer/in: Raphael Rehms
- Trainer/in: Christina Sauer
- Trainer/in: Milena Wünsch
Schedule
- Q&A + exercise session: Friday, 10 - 12
- The enrollment key is OPTIM2022
- Trainer/in: Bernd Bischl
- Trainer/in: Julia Moosbauer
- Trainer/in: Christian Müller
- Trainer/in: Tobias Pielok
- Trainer/in: Mario Teixeira Parente
Description
A variety of digital data sources are providing new avenues for empirical social science research. With the emergence of Big Data, especially data from web sources play an increasingly important role in scientific research. In order to effectively utilize these data for answering substantive research questions, a modern methodological toolkit paired with a critical perspective on data quality is needed. This course introduces computational techniques that are suited for collecting and analyzing digital behavioral data, and for exploring, visualizing and finding patterns in (different types of) data from various sources. In addition, aspects of reproducibility, data quality and error frameworks for digital data are discussed.
Enrolment Key
CSSws2223
- Trainer/in: Unai Fischer Abaigar
- Trainer/in: Christoph Kern
- Trainer/in: Olga Kononykhina
Der Einschreibeschlüssel lautet CoVorMa22
- Trainer/in: Georg Schollmeyer
Sie müssen und können sich NICHT über das lsf für die Veranstaltung anmelden, schreiben Sie sich bitte einfach mit dem Einschreibeschlüssel in diesen Moodle Kurs ein. Alle erhalten einen Platz und es gibt keine Belegfristen.
Einschreibeschlüssel: Stat1Soz2022
Vorlesung Anna-Carolina Haensch (anna-carolina.haensch@stat.uni-muenchen.de)
Übung Jacob Beck, Leah von der Heyde, Sarah Ball
Tutor*innen Jonathan Koop
- Trainer/in: Sarah Ball
- Trainer/in: Jacob Beck
- Trainer/in: Anna-Carolina Haensch
- Trainer/in: Felix Henninger
- Trainer/in: Jonathan Koop
- Trainer/in: Leah von der Heyde
Description
The lecture deals with theoretical and practical concepts from the fields of statistical learning and machine learning. The main focus is on predictive modeling / supervised learning. The tutorial applies these concepts and methods to real examples for illustration purposes.
Organization
- Class: Friday, 8.30-10 am
- Location: Geschw.-Scholl-Pl. 1 / A 119
Enrolment Key
SL_w2223
Target Audience
- Statistics (Methods/Bio/WISO)
- Data Science MSc.
- Trainer/in: Ludwig Bothmann
- Trainer/in: Chris Kolb
- Trainer/in: Philipp Kopper
- Trainer/in: Yawei Li
- Trainer/in: Lisa Wimmer
Deep Learning algorithms have made outstanding results in many domains such as computer vision (CV), natural language processing (NLP), recommendation systems, and medical image analysis. However, the outcome of current methods depends on a huge amount of training labeled data, and in many real-world problems such as medical image analysis and autonomous driving, it is not possible to create such an amount of training data. Learning from unlabeled data can reduce the deployment cost/time of deep learning algorithms which requires annotations from experts such as medical professionals and doctors.
In this seminar, we will learn about the theory of deep unsupervised learning, the Autoregressive models, Generative models, deep learning methods for density modeling, and Self-supervised learning, and will review some state-of-the-art methods and applications of unsupervised/self-supervised algorithms. We will offer different topics with different applications (i.e. NLP, CV) for a variety of tasks (i.e. clustering, representation learning, density modeling, etc). As part of the seminar, you will also apply one of the frameworks to a given real-world problem. This means every participant will be asked to prepare an oral presentation about a current technique and to write up a reproducible case study of actual data analysis in an unsupervised DL framework, in addition to peer-reviewing the (theoretical and practical) work of a colleague.
Recommended prerequisites: Deep learning; Python, PyTorch, TensorFlow, We would also hold the seminar in English and also allow students from other courses (especially DS students)
Seminar: Blocked towards the end of the semester,
Weekly Meeting: Fridays 8:30- 12:30
First meeting: Oct. 21st
Zoom link
Key: "seminar_udl"
The "Advanced Programming (R)" course targets students in the Statistics and Data Science Master's programme. The course can also be taken by advanced Bachelor's students that have taken "Programmieren statistischer Software". Advanced Programming can be credited as WP4/WP7 (PO 2021), or WP2/WP8 (PO 2010).
The first lecture will happen on Thursday, 2022-10-20, 18:00--20:00 c.t., in Ludwigstr. 28 (Y) RG, Room 023.
The second lecture will be on Thursday 2022-10-27, 18:00--20:00 c.t. at the same place.
Times and dates for the lectures that follow will be discussed on 2022-10-20, so please attend the first lecture (or notify the lecturer if you can't come) if you care about coming to the following ones.
Enrollment key: advaprogr2223
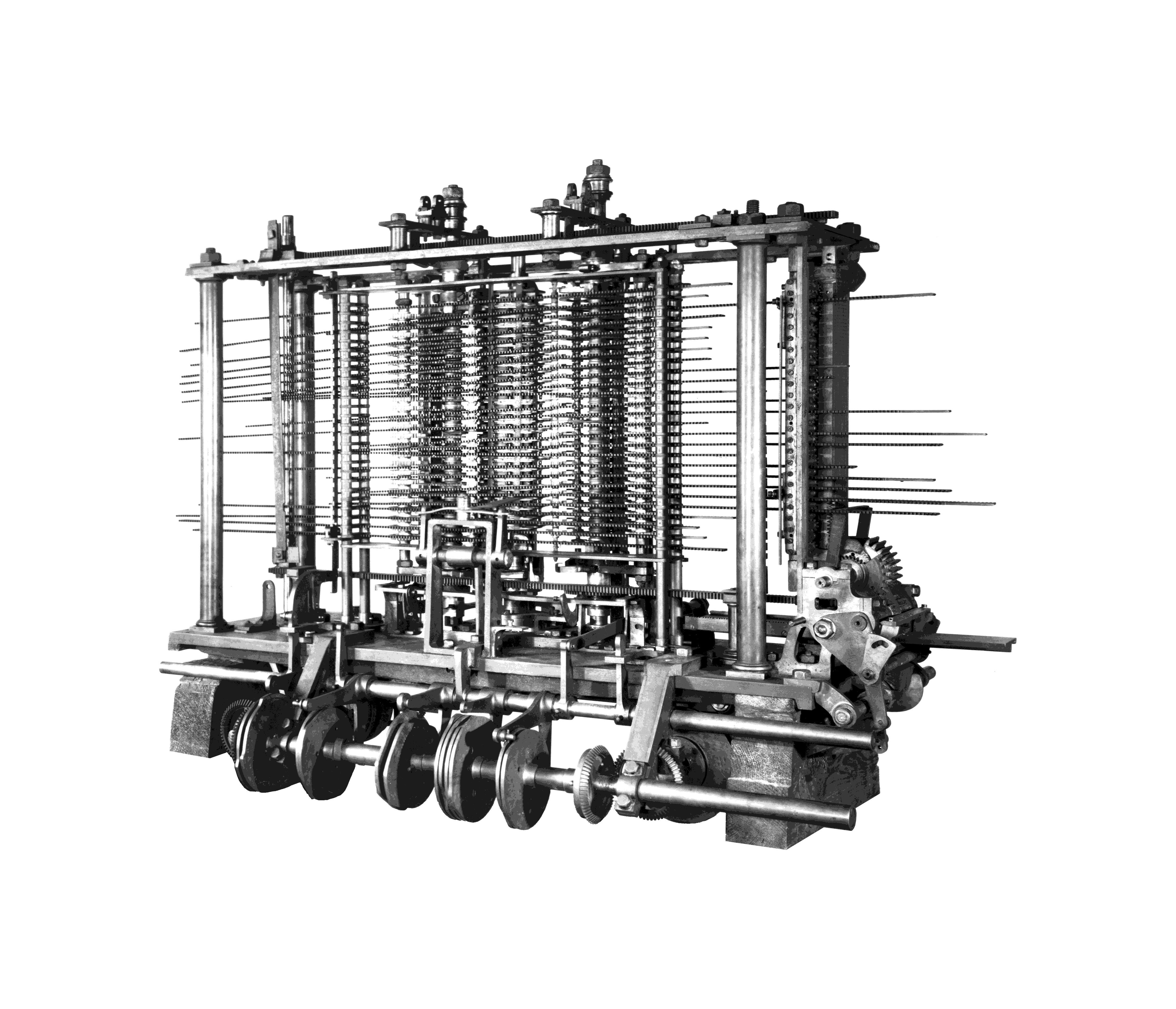
- Trainer/in: Martin Binder
- Trainer/in: Daniel Schalk
Die Veranstaltung "Programmieren
mit Statistischer Software (R)" wendet sich an Studenten im Bachelor
Statistik (3. Semester). Sie baut auf die Veranstaltung "Einführung in
die Statistische Software" (1. Semester) auf.
Termin | Ort | Person | |
---|---|---|---|
Vorlesung: | Fr., 12:00 -- 14:00 c.t. |
Theresienstr. 37/39, B U136 |
Binder, Bender |
Sprechstunde: |
t.b.a. | Vsl. Online |
t.b.a. |
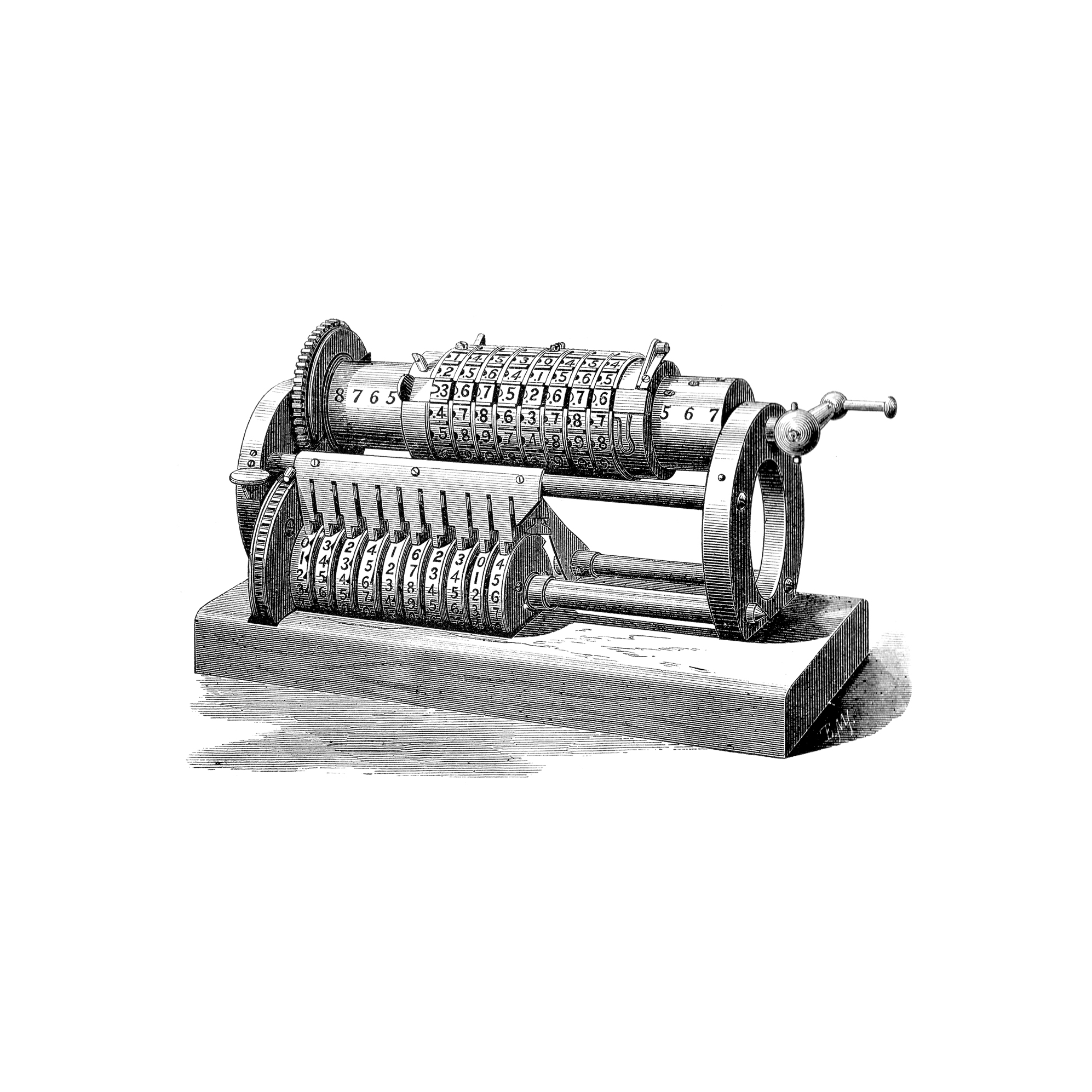
- Trainer/in: Andreas Bender
- Trainer/in: Martin Binder
- Trainer/in: Lukas Burk
- Trainer/in: Noah Hurmer
Einschreibeschlüssel: InfStat12022!
- Trainer/in: Jan Anders
- Trainer/in: Giacomo De Nicola
- Trainer/in: Göran Kauermann
- Trainer/in: Michael Windmann
Termin | Ort | Person | |
---|---|---|---|
Mo., 10 - 12 c.t. |
Geschw.-Scholl-Pl. 1 (A) - A 113 |
Fritz |
|
Fr., 8 - 10 c.t. | Geschw.-Scholl-Pl. 1 (A) - A 125 |
Fritz |
- Trainer/in: Cornelius Fritz
Schedule
- Class: Thursday, 12pm - 14 pm
- Location: Geschw.-Scholl-Pl. 1 (A) - 214
Enrollment key
- The enrollment key is I2ML
- Trainer/in: Ludwig Bothmann
- Trainer/in: Philipp Kopper
- Trainer/in: Lisa Wimmer
Selbsteinschreibung mit dem Passwort WiSo202223
- Trainer/in: Thomas Augustin
- Trainer/in: Sarah Ball
- Trainer/in: Jacob Beck
- Trainer/in: Unai Fischer Abaigar
- Trainer/in: Carla Fuchs
- Trainer/in: Anna-Carolina Haensch
- Trainer/in: Frauke Kreuter
- Trainer/in: Frauke Kreuter
- Trainer/in: Julian Rodemann
- Trainer/in: Jan Simson
- Trainer/in: Leah von der Heyde
Instructor: Frauke Kreuter
The seminar will be held in English on Tuesdays 10:00-11:30 over zoom. Please contact anna-carolina.haensch@stat.uni-muenchen.de with any questions.
Key: SoDa2022
- Trainer/in: Anna-Carolina Haensch
- Trainer/in: Frauke Kreuter
- Trainer/in: Frauke Kreuter
Eine Einschreibung in die Moodle-Seite ersetz nicht die Anmeldung zum Seminar. Zur Anmeldung bitte nur die zentrale Seminaranmeldung nutzen (siehe https://www.statistik.uni-muenchen.de/studium/lehrveranstaltungen/info_anmeldung_seminare.html)
Selbsteinschreibung: Seminar_Klima
- Trainer/in: André Klima
- Trainer/in: Helmut Küchenhoff
Die Veranstaltung wendet sich an Studierende im Bachelor Statistik (3. Semester). Das "Grundlegende Praxisprojekt" (BA Statistik und Data Science - PO 2021) bzw. "Anfängerpraktikum" (BA - Statistik PO 2010) ist eine Pflichtveranstaltung. Der Bachelor-Studiengang Statistik (PO 2010) kennt weiterhin noch die Veranstaltung "Praxisprojekt", welches eine Wahlpflichtveranstaltung (Wahlplichtmodul 6) ist.
Die Veranstaltung wird sowohl während der Vorlesungszeit als auch in den Semesterferien angeboten. Diese Moodle-Seite ist für beide Veranstaltungen.
Selbsteinschreibungsschlüssel: APR2022
- Trainer/in: Helen Alber
- Trainer/in: André Klima
- Trainer/in: Theresa Meier
- Trainer/in: Fabian Scheipl
- Trainer/in: Daniel Schlichting
Die Veranstaltung wendet sich an Studierende mit Hauptfach Statistik und Data Science bzw. Statistik. Das Fortgeschrittende Praxisprojekt (PO 2021) bzw. Statistische Praktikum (PO 2010) ist für Studierende im Bachelor Studiengang Statistik ein Pflichtbestandteil des Studiums. In Gruppen von 3-4 Personen werden Projekte aus der angewandten Statistik bearbeitet. In der Regel besteht ein Projekt aus statistischen Fragestellungen, die sich aus der Zusammenarbeit mit externen Kooperationspartnern ergeben.
Die Veranstaltung wird sowohl während der Vorlesungszeit als auch
in den Semesterferien angeboten. Diese Moodle-Seite ist für beide Veranstaltungen.
Selbsteinschreibungsschlüssel: StatPrak2022
- Trainer/in: Andreas Bender
- Trainer/in: Cornelius Fritz
- Trainer/in: André Klima
- Trainer/in: Helmut Küchenhoff
- Trainer/in: Daniel Racek
- Trainer/in: Maximilian Weigert
Use the registration key (Einschreibeschlüssel) "bigDS22" to read more about the course.
This
course aims to foster the practice of software engineering and project
management techniques in the context of data science and machine
learning projects.
The target audience for this course is master's students from Computer Science, Statistics & Data Science.
Organization
- Lecturers: Bernd Bischl, Giuseppe Casalicchio, Susanne Dandl, Florian Pfisterer
- Time: During workshops: Thursday, 8-12 s.t (Only first weeks)
- Place: CIP-Pool Institute for Statistics (1st Floor)
- ECTS: 12 ECTS (e.g. as an alternative to the Statistical Consulting or Data Science Practical)
Eligibility Requirements
- Good programming skills in Data science-related languages (R, Python, Julia, C++, etc.)
- Predictive Modelling, KDD, Deep Learning, or comparable Machine Learning courses
Projects
- Industry Projects with several Munich-based industry leaders
- Research / Data Science for Social Good projects
- Trainer/in: Giuseppe Casalicchio
- Trainer/in: Susanne Dandl
- Trainer/in: Florian Pfisterer
Die Veranstaltung "Statistische Software (R)" wendet sich an Studierende im Bachelorstudiengang Statistik (1. Semester).
- Trainer/in: Andreas Bender
- Trainer/in: Martin Binder
- Trainer/in: Sophie Hopp
- Trainer/in: Philipp Kopper
- Trainer/in: Julia Niebisch
This course is closely related
to the Generalized Regression Models (Generalisierte Regressions/GRM)
course, also taught by Prof. Dr. Kuechenhoff. This course is, however,
taught in English and designed for students currently attaining their
master’s degree in statistics.
We will take a closer look at
generalized models, mixed models, Bayesian approaches, generalized
additive models, survival analysis and error models. This is not an
exhaustive list, but should provide you with a general idea of the
contents of the course.
If you have questions regarding the course, contact:
Kuechenhoff, Helmut <kuechenhoff@stat.uni-muenchen.de>
Rave, Martje <martje.rave@stat.uni-muenchen.de>
Session |
Instructor |
Schedule | Rythm | Room |
---|---|---|---|---|
Lecture | Helmut Küchenhoff | Tuesday: 12:00 -14:00 Thursday: 12:00-14:00 | weekly | Geschw.-Scholl-Pl. 1 (M) - M 018/ Geschw.-Scholl-Pl. 1 (A) - A 140 Videos : https://cast.itunes.uni-muenchen.de/vod/playlists/5wDnYPs55a.html |
Work Shop |
Martje Rave/ Henri Funk |
Monday: 12:00-14:00 Thursday: 10:00-12:00 | weekly | Schellingstr. 3 (S) - S 001/ Schellingstr. 3 (S) - S 001 MeetingID: 976 3599 7286 Password: GreatStats |
Tutorial | Dielle Syliqi/ Daniel Schlichting |
Thursday: 08:00-10:00 | weekly | Geschw.-Scholl-Pl. 1 (A) - A 213 |
- Trainer/in: Henri Funk
- Trainer/in: Helmut Küchenhoff
- Trainer/in: Martje Rave
- Trainer/in: Daniel Schlichting
- Trainer/in: Dielle Syliqi
Teacher: Walter J. Radermacher
Runtime: 1. October 2022 - 13. October 2022
Format: Self-learning through online videos in the first week and in-person workshops for practical appliance of use cases in the second week.
Examination: Oral Exam (3 ECTS credits)
Language: English
Who is this course for? MsC Statistics and Data Science (2021, WP 28+29+40+46), BsC Statistics and Data Science (2021, WP 8+11), Statistik und Data Science als Nebenfach für Bachelor 30 ECTS (2021, WP 4+5), Statistik und Data Science als Nebenfach für Bachelor 60 ECTS (2021, WP 11+12), Statistik und Data Science als Nebenfach 30 ECTS Mathematik (2021, WP 5+6), Statistik Nebenfach 60 ECTS Bachelor Soziologie (2021, WP 10+11), Grundlegende Statistik als Nebenfach für MA 30 ECTS (2011, WP 6), Vertiefte Statistik Master (2011, WP 5)
Self-Enrolment Key: StatsPublicGood#2223
- Trainer/in: Anna-Carolina Haensch
- Trainer/in: Markus Herklotz
- Trainer/in: Walter Radermacher
Schedule
Time | Lecturer | Begin | |
---|---|---|---|
Lecture |
Monday, 8:30 - 10:00 |
Prof. Dr. Heumann |
24.10.2022 |
Exercise course (Group 1) |
Tuesday, 10:15 - 11:45 |
Sapargali | 25.10.2022 |
Tutorium |
Tuesday, 16:15 - 17:45 |
Eleftheria |
25.10.2022 |
Lecture |
Wednesday, 12:15 - 13:45 |
Prof. Dr. Heumann |
19.10.2022 |
Exercise course (Group 2) |
Thursday, 08:15 - 09:45 |
Garces Arias | 20.10.2022 |
Enrollment Key
- The enrollment key is "stat_inf_ws2223"
- Trainer/in: Esteban Garces Arias
- Trainer/in: Christian Heumann
- Trainer/in: Eleftheria Papavasiliou
- Trainer/in: Nurzhan Sapargali
Schedule
- Live sessions: tbd
- Location: tbd
Enrollment key
- The enrollment key is "openscience22".
- Trainer/in: Andreas Bender
- Trainer/in: Giacomo De Nicola
- Trainer/in: Felix Henninger
- Trainer/in: Martje Rave
- Trainer/in: Lisa Wimmer
Schedule
- Lecture: Wednesday, 9 - 11 c.t.
- Tutorial: Wednesday, 11 - 12 c.t.
- Location: Oettingenstr. 67 B U101
Enrollment key
- Enrollment key: sesame_street
- Trainer/in: Matthias Aßenmacher
- Trainer/in: Ivan Habernal
- Trainer/in: Christian Heumann
- Trainer/in: Marius Roger
- Trainer/in: Hinrich Schütze
- Trainer/in: Leonie Weißweiler
Audience:
- Master Statistik: Räumliche Statistik (P 6.0.38/39)
- Master Biostatistik: Räumliche Statistik (P 8.0.24/25)
- Master Statistik WiSo: Räumliche Statistik (P 7.0.18/19)
- Master Statistics and Data Science: Spatial Statistics (WP45)
- Master Data Science ESG: Elective (WP1 to WP5)
Date/Time:
- Monday 10–12
- Wednesday 14–16
Language:
- The lecture will be in English, unless all students agree to German
Enrollment key:
- Enrollment key is "Matern".
- Trainer/in: Christopher Küster
- Trainer/in: Volker Schmid
Termine
- Vorlesung: Dienstag, 16 - 18 c.t.
- Übungen (Statistik I):
Mittwoch, 12 - 14 c.t. & 14 - 16 c.t.
Donnerstag, 10 - 12 c.t. (2x), 12 - 14 c.t. (2x), 18 - 20 c.t. - Übung (Statistik II):
Donnerstag, 08 - 10 c.t.
Einschreibeschlüssel
- Der Einschreibeschlüssel lautet "22wiwistat23".
- Trainer/in: Mostafa Amin
- Trainer/in: Matthias Aßenmacher
- Trainer/in: Polina Gordienko
- Trainer/in: Anne Gritto
- Trainer/in: Christopher Küster
- Trainer/in: Antonio Melieni
- Trainer/in: Lea Schulz-Vanheyden
- Dienstag 10–12 (Vorlesung)
- Donnerstag 12–14 (Vorlesung)
- Donnerstag 14–16 (Übung)
- Dienstag 18–20 (Tutorium)
Einschreibung
Einschreibeschlüssel: Bayes
- Trainer/in: Michael Kobl
- Trainer/in: Marie Scherzer
- Trainer/in: Volker Schmid