6 meetings in September and October (irregular schedule, usually late afternoon on Thursday or Friday, depending on availability of guest speakers). Most speakers have a background in social data science but the topics are hopefully of interest to all interested in a PhD (How to find a PhD position/PhD Aborad/PhD as a career step in industry/academia...).
Offered as WP40 for Master students.
Key: htdaphd
- Trainer/in: Anna-Carolina Haensch
- Trainer/in: Frauke Kreuter
- Trainer/in: Tomasz Olma
- Trainer/in: Vincent Starck
- Trainer/in: Daniel Wilhelm
Termine
- Dieser Kurs ist als Kurs zum Selbststudium konzipiert.
Einschreibeschlüssel
- Der Einschreibeschlüssel lautet "miniconda3".
- Trainer/in: Ludwig Bothmann
Termin: Do 16.15-18.00 in D209
Die Ringvorlesung gibt einen Überblick über verschiedene Themengebiete der Statistik, die in den spezifischen Modulen nicht entsprechend behandelt werden können.
Geplant sind
* verschiedene Gastvorträge aus der Berufspraxis
* einige Vorträge zur Geschichte der Statistik und der Künstlichen Intelligenz inklusive ihrer Grundlagen
* Überblicksvorträge über Teilgebiete der aktuellen statistischen Forschung und damit über die verschiedenen Spezialisierungen im Masterstudium
* ein Themenblock zu Kommunikation statistischer Ergebnisse, Datenjournalismus und Open Science
Einschreibeschlüssel: Ringvorlesung
Die Veranstaltung bildet zusammen mit dem Grundlegenden Praxisprojekt das Pflichtmoduls P11: Einführung in die praktische Statistik (6 ECTS-Punkte). Es findet keine eigenständige Prüfung zu diesem Modulteil statt. Der Erwerb der entsprechenden Kompentenzen (3 ECTS-Pukte) kann zum Beispiel durch regelmäßige aktive Anwesenheit nachgewiesen werden. (Aternativen nach Rücksprache mit dem Veranstatltungsleiter.)- Trainer/in: Thomas Augustin
o Content:
Areal data is a data format in which point observations are aggregated over subregions of a predefined space. These subregions are non-overlapping and make up the entire space. This format is predominately common in medical research and one of the central formats in which, for example, data on Corona infections and hospitalizations were available. Due to the loss of information on the specific point of observation, the estimation of the spatial correlation can become a bit trickier than in spatial point processes. In recent years, especially during the pandemic, working with this type of data has become more relevant for data scientists and statisticians not only due to the relevance of their context but also due to somewhat recent advancements in research on network or graph theory, which allowed research on the statistical methods designed to work with this data more diverse.
We will start of by going over the basic concepts in areal data analysis from Moran’s I over to a brief gloss over Markov Random Fields. With this we can then explore more classical methods on areal data analysis, such as Bayesian MCMC methods in the BYM model framework, up to models which have been developed in recent years and include more theoretical concepts of graph theory. It is also possible to explore some machine learning models designed to work with areal data, as well as taking a closer look at integrated nested Laplace approximation (INLA).
o Schedule:
o Kick off (within the first two weeks of the semester) we will talk about the basics of spatial statistics
o
Phase
1: Foundations (2 weeks)
In the first 2 weeks after the
kick off, we’ll meet weekly (!) to discuss a specific section of Spatial
Statistics and Modeling (Gaetan) We’ll
work through chapter 5.2 and 5.3 as well as some excerpts of Applied
Spatial Data
Analysis with R (Bivand) at first to establish a foundation for us to work
with.
o Phase 2: Book club (2 weeks)
You will be asked to present a paper out of a selection of papers to the other students (45 mins to 1 hour) thereafter we will discuss the content of the presentation (30%= 20% presentation+ 10% discussion)
o Phase 3: Implementation (5 weeks)
You will be asked to pick a dataset out of the ones provided or find your own in which you will apply one of the methods you found interesting in the book club phase.
o Phase 4: Presentations (1 week)
You will hold a short presentation 10-15 mins about your project. (30%=20% presentation+ 10% discussion)
Phase 5:
You will write and hand in a final report on your implementation; this does not have to be handed in before the end of the semester, but should be within 3 or 4 weeks of the last day of lectures. (Not more than 10 pages) (40%)
- Trainer/in: Martje Rave
Curricular Embedding: 6 ECTS, Master's programme in Statistics and Data Science: "Narrow electice (2 out of 3)" in the Methodology and Modelling track, "wider elective" in the Social Statistics and Data Science and the Econometrics tracks, "general elective" for all tracks; elective in the Master's programme Versicherungs- und Finanzmathematik; options for other programmes upon request.
Contents: Decision theory deals with rational decisions under uncertainty. It has high interdisciplinary importance, for example, in the analysis and support of decisions in business administration or finance (e.g. investment strategies), economics or sociology (rational choice theory), medicine (e.g. expert systems) or engineering (e.g. autonomous control). Moreover, statistical decision theory can be seen as a formal framework for choosing analysis methods (optimal tests or estimators, best classification algorithms, etc.). This general view, understanding statistics and machine learning as special cases of decision theory, plays a fundamental role in the critical analysis and problem-adequate generalization of any data-based learning procedure.
The course first discusses the general structure of decision problems, including fundamental decision principles. Then it analyzes and characterizes the Bayes and minimax criteria as extreme poles to deal with (state) uncertainty and develops modern alternatives in the context of complex uncertainty (ambiguity).
Enrolment Key: DT24
Additional Note:
If you are interested in active participation in the course but cannot
always come in person for good reasons, you are welcome to contact me
(Thomas Augustin). We are currently investigating whether we can offer a
fallback Zoom solution.
- Trainer/in: Thomas Augustin
- Trainer/in: Ivan Melev
The course offers a unique, first-hand introduction to official
statistics. It is based on video material produced by colleagues from
Eurostat, the German Federal Statistical Office, and the statistics
departments of the European Central Bank and the Deutsche Bundesbank.
The material is successively made available for self-study and then
discussed in classroom meetings, during which some of the lecturers from
official statistics and students from Trier University will join us.
Enrolment key: EMOS-B24
Time: Tuesday from 4 pm (s.t.) to 5.30 pm, approximately every second week
Begin: Tuesday, April 19 in hybrid format (F007 (Main Building) and Zoom)
- Trainer/in: Thomas Augustin
Enrolment key: RegCorDat24
Date: Thursday 14:00-16:00
This is an inverted classroom style - course. We only meet once a week despite this being 4 hour / week because you are expected to come prepared to every session
-- that means: you've watched the lecture videos on LMUcast, you've
reviewed the slides, you've worked through the self-assessment quizzes,
and you've posted the questions you would like to see
addressed during the session in the Moodle forum.
Exercise sheets will be available at least one week
in advance, with full written solutions available before and discussed
during Thursday in-person sessions.
You are expected to hand in solutions for exercise sheets 2 and 4 via Moodle, due on May 09 and May 30, respectively.
Grading: Oral exam at the end of the semester.
- Trainer/in: Fabian Scheipl
Dates:
Date | Place | Person | Start | |
---|---|---|---|---|
Lecture | Wed, 12:15-13:45 | Geschw.-Scholl-Pl. 1 (M) / M 209 | Nagler | 17.04.24 |
Lecture/Exercise | Thu, 10:15-11:45 | Prof.-Huber-Pl. (W) / Lehrturm-W401 | Nagler/Gauss | 18.04.24 |
Enrolment
- The enrolment key is: "rademacher"
- Trainer/in: Jana Gauß
- Trainer/in: Thomas Nagler
Syllabus:
- Overview
- Fundamentals and Properties of Stochastic Processes
- Univariate ARIMA-Processes
- Estimation and Forecasting of ARIMA-Models
- Univariate GARCH-Models + Extensions
- Selected aspects: Long Memory und Fractional Differencing, Threshold-Models
Intended audience: Advanced bachelor and master students of statistics, mathematics, computer science (Informatik), economics and business administration.
Prerequisites: Solid mathematical foundations (analysis and linear algebra), basic knowledge in econometrics (econometrics 1) or statistics (linear models).
Record of Achievement:
- Trainer/in: Dennis Mao
The course Analysis of high-dimensional biological data (formerly Statistische Methoden in Genomik und Proteomik / Statistical methods for biological high-throughput data) will cover important statistical methods and concepts for the analysis of high-dimensional biological high-throughput data. We will focus on bulk RNA-Seq, single-cell RNA-Seq, proteomic, metabolic, and especially microbiome data such as 16S rRNA and other amplicon data.
All lectures and exercises will be taught in English. Most lectures are already recorded and are available online (see Announcements for the link). The lecture slot will be used as flipped classroom in person. The flipped lectures will not be recorded.
The first in-person meeting will be on April 17 where we discuss admin and general course structure topics.
Enrollment key: HighDimBio24
- Trainer/in: Christian Müller
- Trainer/in: Stefanie Peschel
Enrolment Key
SDS-Methods-24
- Trainer/in: Unai Fischer Abaigar
- Trainer/in: Christoph Kern
- Trainer/in: Jan Simson
Der Einschreibeschlüssel lautet: MatheIINF2024
- Trainer/in: Christoph Jansen
- Trainer/in: Georg Schollmeyer
Dates [Summer Term 2024]
Lecture: Monday, 12h15 - 13h45
Lecture/Exercise (alternating): Tuesday, 08h30 - 10h00
If self-enrollment in this moodle course does not work automatically, you need to register via the backdoor ;)
--> Enrollment key: backdoor
- Trainer/in: Julian Rodemann
- Trainer/in: Michael Schomaker
- Trainer/in: Michael Schomaker
- Trainer/in: Christoph Wiederkehr
Instructors:
- David Rügamer
- Emanuel Sommer
- TBD
Kick-off Meeting
- Wednesday, April 17, 12-2pm
- Via Zoom
Credits and Contents
- Credits: 6 ECTS
- Applied Software Projects, working in a group of 1-3 students
- Final Submission Date: Open for Discussion
Enrollment key: TensorTorchJax
- Trainer/in: David Rügamer
- Trainer/in: Emanuel Sommer
This course is about the theoretical foundations of probabilistic and Bayesian DL
-- Only for participants that have been officially assigned to the seminar --
- Trainer/in: Cornelia Gruber
- Trainer/in: Thomas Nagler
- Trainer/in: Tobias Pielok
- Trainer/in: Raphael Rehms
- Trainer/in: David Rügamer
- Trainer/in: Rickmer Schulte
- Trainer/in: Emanuel Sommer
The lecture aims at providing a basic theoretical and practical understanding of modern neural network approaches.
Note: Both the lecture and the lab sessions start in the first week of the summer semester (15.04 - 19.04)
Time | Place | Lecturers | |
---|---|---|---|
Lecture | Mo 4-6pm (c.t.) | Geschw.-Scholl-Pl. 1 (M) - M 014 | David Rügamer |
Lab Session | Tue 14-16pm (c.t.) | Geschw.-Scholl-Pl. 1 (E) - E 216 | Emanuel Sommer |
Enrollment key: DLearn
- Trainer/in: David Rügamer
- Trainer/in: Emanuel Sommer
People and Dates
Date and Time | Place | Lecturer | |
Lecture | Mo. 10.00-12.00 | Geschw.-Scholl-Pl. 1 (M) -M 218 | David Rügamer |
Tue. 10.00-12.00 | Geschw.-Scholl-Pl. 1 (E) - E 004 | ||
Exercise | Thu. 12.00-14.00 | Geschw.-Scholl-Pl. 1 (B) - B 006 | Rickmer Schulte |
Tutorial | Thu. 08.00-10.00 | Geschw.-Scholl-Pl. 1 (A) - A 021 | Elisa Noltenius |
Enrolment key: StatMod_s24
- Trainer/in: Andreas Bender
- Trainer/in: David Rügamer
- Trainer/in: Rickmer Schulte
Format
Class
- Time: Wednesday 10:15-11:45 h
- Location: M 105
Exercise
- Time: Tuesday 08:15-09:45 h
- Location: A 021
Enrollment key
- The enrollment key is I2ML.
- Trainer/in: Ludwig Bothmann
- Trainer/in: Fiona Ewald
- Trainer/in: Lisa Wimmer
Einschreibeschlüssel: Infstat22024!
- Trainer/in: Jan Anders
- Trainer/in: Sergio Buttazzo
- Trainer/in: Giacomo De Nicola
- Trainer/in: Jan Deller
- Trainer/in: Göran Kauermann
Der Einschreibeschlüssel ist StoSta24
Der Einschreibeschlüssel ist StoSta24
Termine
- Trainer/in: Sergio Buttazzo
- Trainer/in: Ivan Melev
- Trainer/in: Ivan Melev
- Trainer/in: Nurzhan Sapargali
- Trainer/in: Fabian Scheipl
The lecture deals with theoretical and practical concepts from the fields of statistical learning and machine learning. The main focus is on predictive modeling / supervised learning. The exercise session applies these concepts and methods to real examples for illustration purposes.
Schedule:
Person | Start | ||||
---|---|---|---|---|---|
Lecture | Wednesday, 12:15-13:45 |
| Bischl | 16.04.24 | |
Exercise session | Thursday, 16:15-17:45 | Schellingstr. 3 (S) - S 003 | Pielok | 18.04.24 |
Enrolment key
- The enrolment key is: SL_ss24
- Trainer/in: Bernd Bischl
- Trainer/in: Chris Kolb
- Trainer/in: Yawei Li
- Trainer/in: Tobias Pielok
Schedule:
Person | Beginning | |||
---|---|---|---|---|
Lecture/Exercise | Tuesday, 10:15-11:45 | B 006 | Hoffmann/Boulesteix | 16.04.24 |
Enrolment key
- The enrolment key is: "Pitfalls24"
- Trainer/in: Anne-Laure Boulesteix
- Trainer/in: Sabine Hoffmann
- Trainer/in: Hannah Kümpel
- Trainer/in: Raphael Rehms
Termin | Ort | Person | Beginn | |
---|---|---|---|---|
Vorlesung | Di, 08:30-10:00 Mi, 10:15-11:45 |
Geschw.-Scholl-Pl. 1 (D) / D 209 Schellingstr. 3 (S) / S 005 |
Thomas Nagler | 16.04.2024 |
Übungsgruppe 1 | Mo, 16:15-17:45 | Geschw.-Scholl-Pl. 1 (M) - M 010 | Nicolai Palm | 22.04.2024 |
Übungsgruppe 2 | Do, 12:15-13:45 | Geschw.-Scholl-Pl. 1 (D) - D 209 | Jana Gauß | 18.04.2024 |
Tutorium | Fr, 12:15-13:45 | Schellingstr. 3 (S) / S 004 | Eugen Gorich | 19.04.2024 |
Einschreibeschlüssel
- Der Einschreibeschlüssel lautet: "linalg2024"
------------------------------------------------------------------------
- Trainer/in: Jana Gauß
- Trainer/in: Thomas Nagler
- Trainer/in: Nicolai Palm
Schedule
Time | Lecturer | Begin | |
---|---|---|---|
Lecture | Tuesday, 12:15 - 13:45 | Prof. Dr. Heumann | 16.04.2024 |
Exercise course (Group 1) | Wednesday, 08:15- 09:45 | Sapargali, Garces Arias | 24.04.2024 |
Exercise course (Group 2) | Wednesday, 14:15 - 15:45 | Sapargali, Garces Arias | 24.04.2024 |
Lecture | Friday, 10:15 - 11:45 | Prof. Dr. Heumann | 19.04.2024 |
Tutorium | Friday, 08:15 - 09:45 | Stephan | 26.04.2024 |
Enrollment Key
- The enrollment key is "stat_inf_s24"
- Trainer/in: Stephan Bark
- Trainer/in: Esteban Garces Arias
- Trainer/in: Christian Heumann
- Trainer/in: Nurzhan Sapargali
Short Description: You will learn how to tackle practical challenges in ML using R. Topics include an introduction to an ML toolbox in R, (advanced) tuning techniques, custom ML pipelines, classifier calibration, handling class imbalances, ML benchmark experiments with hypothesis testing, ML model ensembling/stacking, and ML interpretability. Sessions consist of brief methodological overviews followed by hands-on exercises, requiring laptops for full engagement.
Prerequisite: To participate in this lecture, you must have completed and passed the courses:
- Introduction to Machine Learning (I2ML)
- Programming with Statistical Software (R), ideally with a good grade as good knowledge of R is required
Kick-off: Wednesday, April, 17th from 09:00 - 12:00 (s.t.) at Theresienstr. 39 - B 139 (check Moodle for up-to-date information)
Enrolment key: appml24
- Trainer/in: Bernd Bischl
- Trainer/in: Giuseppe Casalicchio
- Trainer/in: Fiona Ewald
- Trainer/in: Matthias Feurer
- Trainer/in: Holger Löwe
This course will discuss essential research techniques in statistics and data science, also preparing students for successfully participating in seminars and writing a thesis. The material presented focuses mainly on classical research techniques and a first understanding of research as a social system. Beyond this, classroom discussions will hopefully help combine the classical techniques with "modern personal knowledge management methods", which are currently also intensively disseminated by different YouTube authors.
The course is part of the Bachelor’s programmes in Statistics and Data Science (150 major or 60 ECTS minors: `Methoden und Techniken des wissenschaftlichen Arbeitens’, P16.1, and WP 12.1 / WP 13.1, respectively ). All interested Master’s students and Bachelor's students from the "old PO" are most welcome as well. A certificate can be issued for active personal attendance.
NEW: Minor students and Bachelor's degree Erasmus students who will not attend a seminar can obtain 3 ECTS via an examation at the end of the course.
Time:
Monday 12.15 to 14.00, M 110 (main building), on April 22, May 6, May
13, May 20, June 5, and on a further date at the end of June (to be
arranged).
Enrolment key: ResTech24
If you are interested in active participation in the course but cannot always come in person for good reasons, you are welcome to contact me (Thomas AugustiIn). We are currently investigating whether we can offer a fallback Zoom solution.
- Trainer/in: Thomas Augustin
Schedule:
Person | Beginning | |||
---|---|---|---|---|
Lecture | Tuesday, 14:15-16:45 | Richard-Wagner-Str. 10 / D 105 | Hoffmann/Boulesteix | 16.04.24 |
Exercise session | Monday, 10:15-11:45 | E 004 | Hornung | 29.04.24 |
Enrolment key
- The enrolment key is: "DAS24"
- Trainer/in: Anne-Laure Boulesteix
- Trainer/in: Sabine Hoffmann
- Trainer/in: Roman Hornung
- Trainer/in: Christina Sauer
Dates: Wednesday 24.04.; 08.05.; 29.05.; 26.06.; 03.07.; 10.07.; 17.07.
Time: 09:00 - 12:00 s.t.
Location: A213 main building
Enrollment Key: MissingData24Course Description
Missing data are a common problem in almost any dataset which can lead to biased results if the missingness is not taken into account at the analysis stage. Imputation is often suggested as a strategy to deal with missing values allowing the analyst to use standard complete data methods after imputation. However, several misconceptions about the aims and goals (isn't imputation making up data?) of imputation make some users skeptical about the approach. In the first part of the course we will illustrate why thinking about missing data is important and clarify which goals a useful imputation method should try to achieve (and which not).The second part of the course will provide a detailed introduction to multiple imputation, a convenient strategy for dealing with missing data. We will motivate the concept and illustrate why multiple imputation should generally be preferred over single imputation methods. The main focus of the course will be on strategies to generate (multiple) imputations and how to deal with common problems when applying the methods for large scale surveys. We will also discuss various options for assessing the quality of the imputations. All concepts will be demonstrated using software illustrations in R.
Curricular Embedding
The course can be recognized as 'Selected Topics of Social Statistics and Social Data Science' (3 ECTS, WP 40). Alternatively, the course can be combined with the course "Measurement and Modelling, Part A" (offered each winter semester) to the 6 ECTS Module "Measurement and Modelling" (WP 38).
- Trainer/in: Thomas Augustin
- Trainer/in: Jörg Drechsler
- Trainer/in: Jörg Drechsler
- Trainer/in: Anna-Carolina Haensch
- Trainer/in: Lea Höhler
Einschreibeschluessel: s24dcqd
Block course 5. August-8. August + 12. August - 15. August
- Trainer/in: Anna-Carolina Haensch
- Trainer/in: Frauke Kreuter
- Trainer/in: Joseph Sakshaug
Die Veranstaltung wendet sich an Studierende im Bachelor Statistik (3. / 4. Semester). Das "Grundlegende Praxisprojekt" (BA Statistik und Data Science - PO 2021) ist eine Pflichtveranstaltung (Modul P 11.1).
Die Veranstaltung wird während der Vorlesungszeit angeboten. Diese Die Einführungsveranstaltung mit Anwesenheitspflicht findet am 23.04. 9-11 statt.
Aus organisatorischen Gründen ist eine frühzeitige, separate Anmeldung für die Teilnahme während der Vorlesungszeit nötig -- schreiben Sie sich bitte ein und gehen Sie dann zur Anmeldung auf der Kursseite.
Einschreibeschlüssel: grndlgnprks24
- Trainer/in: Helmut Küchenhoff
- Trainer/in: Johannes Piller
Enrolment key: ml24
- Trainer/in: Zhan Lin
- Trainer/in: Daniel Wilhelm
Enrolment key: semml24
- Trainer/in: Daniel Wilhelm
Advanced Statistical Programming using R
The enrolment key / password for the course is: FortStatSoftNF.
If you have questions please contact statprog@stat.uni-muenchen.de.
The course will be taught in English, more information can be found in the course itself.
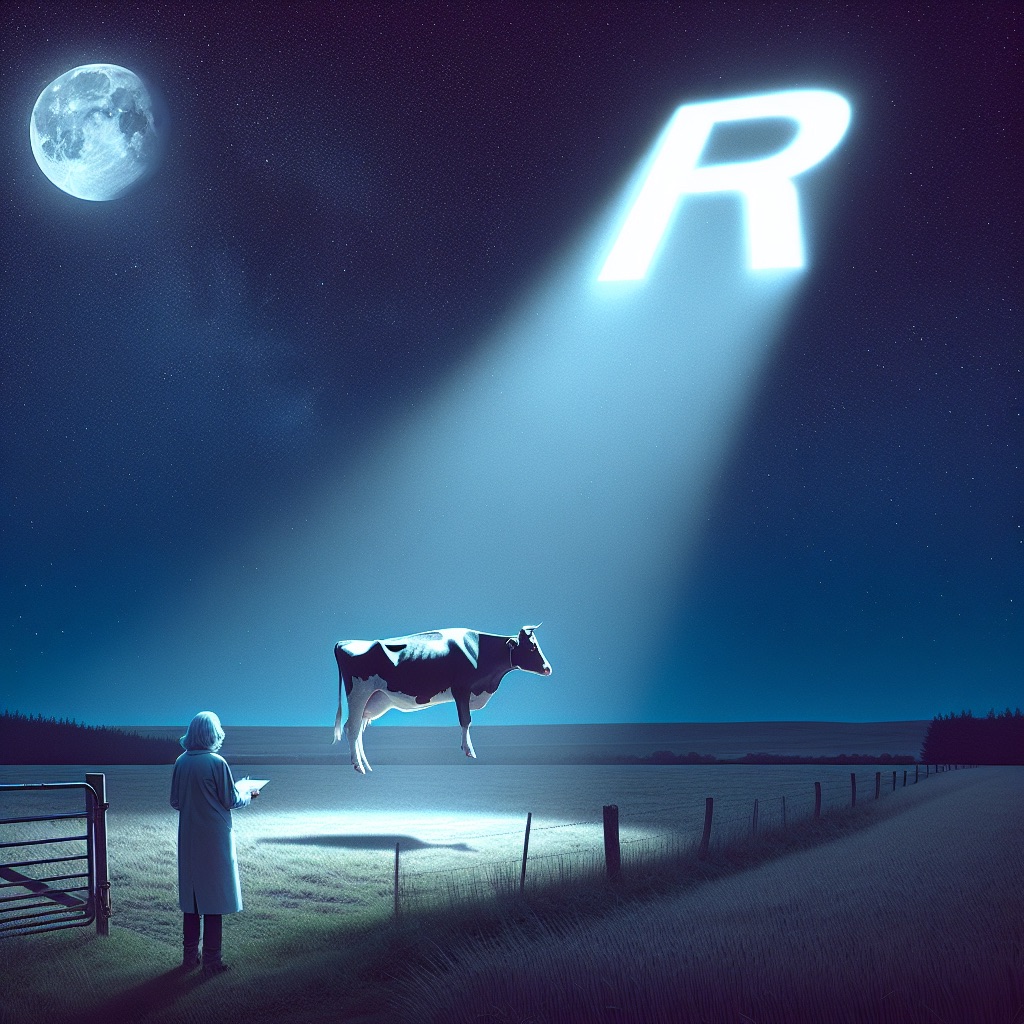
- Trainer/in: Jacob Beck
- Trainer/in: Lisa Bondo Andersen
- Trainer/in: Markus Herklotz
- Trainer/in: Jan Simson
- Trainer/in: Anna Steinberg
- Trainer/in: Jonas Stiehler
Organisation
Veranstaltung | Termin | Ort | Person |
---|---|---|---|
Vorlesung |
Mo, 12.00 - 14.00 |
Geschw.-Scholl-Pl. 1 - E 216 |
Küchenhoff |
Vorlesung |
Do, 08.00 - 10.00 |
Schellingstr. 3 - S 004 |
Küchenhoff |
Übung |
Di, 10.00 - 12.00 |
Geschw.-Scholl-Pl. 1 - F 007 |
Rave / Piller |
Übung |
Do, 14.00 - 16.00 |
Geschw.-Scholl-Pl. 1 - F 007 |
Rave / Piller |
Tutorium |
Di, 12.00 - 14.00 |
Geschw.-Scholl-Pl. 1 - F 007 |
Einschreibeschlüssel
LiMo_s24
- Trainer/in: Helmut Küchenhoff
- Trainer/in: Johannes Piller
- Trainer/in: Martje Rave
- Trainer/in: Christina Sauer
- Trainer/in: Robin Schüttpelz
Der Schlüssel zum Einschreiben ist Stat2NFSoz24.
- Trainer/in: Anna-Carolina Haensch
- Trainer/in: Niklas Ippisch
- Trainer/in: Bolei Ma
- Trainer/in: Clara Strasser Ceballos
- Trainer/in: Leah von der Heyde
- Bachelor Statistik und Data Science: WP7 Ausgewählte Gebiete der statistischen Modellierung
- Nebenfach Statistik und Data Science (60 ECTS): WP6 Einführung in die Bayes-Statistik
- Nebenfach Statistik und Data Science (30 ECTS): WP8 Einführung in die Bayes-Statistik
- Bachelor Informatik mit integriertem Anwendungsfach Statistik: WP40 Einführung in die Bayes-Statistik
- Bachelor Wirtschaftsmathematik: P17 Ausgewählte Gebiete der angewandten Statistik
- Andere Studiengänge auf Anfrage
Einschreibeschlüssel: HaroldJeffreys
- Trainer/in: Martje Rave
- Trainer/in: Volker Schmid
Enrollment Key: repro_nlp
- Trainer/in: Esteban Garces Arias
- Trainer/in: Christian Heumann
A first meeting will take place at the beginning of the semester (will be scheduled in agreement with the participants), where the seminar topics are briefly introduced and assigned to the participants.
The main part of the seminar with the presentations and discussions will be held as a block during the semester
Seminar type: Block-type and in-person
Language: The seminar will be held in English
Target group: Bachelor and Master in Statistics
Recognition possibilities: Biostatistics, Methodology and Modeling
- Trainer/in: Stefanie Peschel
Enrollment Key: automl
- Trainer/in: Matthias Feurer
The seminar will present and discuss current statistical analyses on the topic of the climate crisis by addressing central topics from a joint research project (CLIMEX II) with the LMU Chair of Physical Geography and Environmental Modelling. There will be two focal points.
Statistical modelling of climate change, extreme climatic events and natural hazards. This involves uncertainty quantification of climate models, drought forecasts for Bavaria, modelling of extreme events
Effects of climate change on human health. In particular, models that attempt to analyse the current and future adverse effects (e.g., the number of additional deaths) caused by climate change will be discussed. In particular, analyses by the Intergovernmental Panel on Climate Change (IPCC) will be discussed.
Students are expected to engage intensively with the scientific literature and present the statistical methodology for the chosen topic. It is possible to present your topics and, if suitable, to work on them in the seminar.
Key: Climate24
- Trainer/in: Henri Funk
- Trainer/in: Helmut Küchenhoff
Schedule
Type | Date | Location | Start |
---|---|---|---|
Lecture | Wednesdays 08:15-10:45 | Geschw.-Scholl-Pl. 1 (A) / A 017 | 17.04.2024 |
Lecture/Tutorial | Fridays 08:15-10:00 | 19.04.2024 |
Enrolment Key: ectheory24
- Trainer/in: Mauricio Olivares Gonzalez
Date |
Time | Location |
|
---|---|---|---|
Initial meeting | 16.04.2024 |
9:00-12:00 | #144, Ludwigstr. 33 |
Binding registration (Econ M.Sc.) |
19.04.2024 |
- | - |
Final presentations |
11.07.2024 |
8:30-18:00 | #144, Ludwigstr. 33 |
Term paper submission |
31.08.2024 | - |
The enrollment key will be communicated to the registered students by email.
- Trainer/in: Mauricio Olivares Gonzalez
- Trainer/in: Tomasz Olma
Termine:
Termin | Ort | Person | Beginn | |
---|---|---|---|---|
Vorlesung | Di, 12:15-13:45 | Geschw.-Scholl-Pl. 1 (A) - A 021 | Schulz-Kümpel | 16.04.24 |
Übung/Vorlesung | Do, 12:15-13:45 | Geschw.-Scholl-Pl. 1 (A) - A 022 | Schulz-Kümpel | 18.04.24 |
Einschreibeschlüssel: multi_verfahren_s24
- Trainer/in: Hannah Kümpel
Statistik II für Statistiker: Wahrscheinlichkeitstheoretische Grundlagen der Statistik
Termin | Hörsaal | Dozent | |
---|---|---|---|
Vorlesung | Mo 10-12 | Volker Schmid | |
Do 10–12 | |||
Übung Gruppe 1 | Mo 14–16 | Martje Rave, N.N. | |
Übung Gruppe 2 | Mi 8–10 | ||
Tutorium | Di 18-20 | Michael Kobl |
Einschreibeschlüssel: Poisson
- Trainer/in: Yichen Han
- Trainer/in: Michael Kobl
- Trainer/in: Martje Rave
- Trainer/in: Patrick Schenk
- Trainer/in: Patrick Schenk
- Trainer/in: Marie Scherzer
- Trainer/in: Volker Schmid
- Trainer/in: Maxim Winter
Termine
- Vorlesung: Dienstag, 16 - 18 c.t.
- Übungen (Statistik II):
Mittwoch, 12 - 14 c.t. (2x) & 14 - 16 c.t. (2x)
Donnerstag, 18 - 20 c.t.
Freitag, 10 - 12 c.t. - Übung (Statistik I):
Montag, 10 - 12 c.t.
Einschreibeschlüssel
- Der Einschreibeschlüssel lautet "2024wiwistat".
- Trainer/in: Matthias Aßenmacher
- Trainer/in: Benjamin Dornow
- Trainer/in: Polina Gordienko
- Trainer/in: Franziska Reichmeier
- Trainer/in: Teresa Rupprecht
- Trainer/in: Michael Sawitzki
- Trainer/in: Charlotte Schöllkopf