Dates:
Tuesdays, 16-18 Uhr for more detail see the Syllabus
Content:
This course first reflects on official statistics about social matters like income, living conditions, poverty measures, and unemployment. Hereby, a focus is put on the measurement of social and abstract constructs. Later, the most important voluntary and compulsory surveys conducted by official statistics are discussed, as well as recent developments in the area of processed produced external data and so-called smart statistics. Then, the module turns to business statistics with respect to national accounts, terms of trade, and indicators of economic development.
Learning outcome:
After this course students will know the benefits as well as the difficulties trying to quantify social constructs. They are familiar with the major surveys in official statistics, their specific characteristics and their special quality standards. They understand the specific requirements as well as methodological opportunities and challenges of new data sources.
Enrollment key: EMOS
- Enseignant: Jan Pablo Burgard
Vorlesungszeiten:
- Mittwoch: 8:30-10:00 Uhr Hauptgebäude B 106
- Donnerstag: 10:15-11:45 Uhr Hauptgebäude B 106
Vorlesungsinhalt:
Das Modul gibt einen Überblick über die Grundlagen und die Anwendung der wichtigsten Stichprobenverfahren.
Zunächst wird in die grundlegenden Ideen von Stichprobenziehungen eingeführt und die einfache Zufallsstichprobe und das Ziehen ohne Zurücklegen vorgestellt. Danach werden sowohl modellbasierte Verfahren als auch designbasierte Verfahren der Stichprobenziehung behandelt. Das Horwitz-Thompson Prinzip wird ausführlich besprochen, und es werden Clusterstichproben als auch geschichtete Stichproben vorgestellt. Das Modul schließt ab mit kombinierten und mehrstufigen Verfahren
Qualifikationsziele:
Die Studierenden kennen unterschiedliche Stichprobenverfahren und können je nach Anwendungsfall das passendste Stichprobenverfahren auswählen und praktisch umsetzen.
Enrollment key: sampling
- Enseignant: Jan Pablo Burgard
Termin: Donnerstag, 16.15-18.00 in A214 (Hauptgebäude); Beginn: 24.4.2025
Die Ringvorlesung ist der zweite Bestandteil des Moduls P11: Einführung in die praktische Statistik. Sie gibt einen Überblick über verschiedene Themengebiete der Statistik, die in den spezifischen Modulen nicht entsprechend behandelt werden können.
Geplant sind
- verschiedene Gastvorträge aus der Berufspraxis
- einige Vorträge zur Geschichte der Statistik und der Künstlichen Intelligenz inklusive ihrer Grundlagen
- Überblicksvorträge über die verschiedenen Spezialisierungen im Masterstudium und damit über aktuelle Teilgebiete der gegenwärtigen statistischen Forschung
- ein Themenblock zur Ethik des statistischen Arbeitens
Einschreibeschlüssel: Ringvorlesung
- Enseignant: Thomas Augustin
Time and Dates: Thursdays,
12:15 pm to 1.45 pm, M114 (main building). We will have 5 meetings in
the first half of the semester: April 24, May 8 and 22 (note the correction!), June 5 and 12;
one meeting for further mutual exchange will take place at the end of
the semester (tbd.).
Overview: This course will discuss essential research techniques in statistics and data science, also preparing students for successfully participating in seminars and writing a thesis. The material presented focuses mainly on classical research techniques and a first understanding of research as a social system. Beyond this, classroom discussions will hopefully help combine the classical techniques with "modern personal knowledge management methods", which are currently also intensively disseminated by different YouTube authors, and own experience in using generative AI tools.
The course is part of the Bachelor’s programmes in Statistics and Data Science (150 major or 60 ECTS minors: `Methoden und Techniken des wissenschaftlichen Arbeitens’, P16.1, and WP 12.1 / WP 13.1, respectively). Bachelor's students from the "old POs" and Master’s students are most welcome as well. A certificate can be issued for active personal attendance.
Minor students and Bachelor's degree Erasmus students who will not attend a seminar can obtain 3 ECTS via an examination/small project at the end of the course.
Enrollment key: ResTech25
- Enseignant: Thomas Augustin
Enrolment key: ml25
- Enseignant: Daniel Wilhelm
der Einschreibeschlüssel lautet: Alexis-Claude Clairaut
- Enseignant: Georg Schollmeyer
Key: dcqd2025
Dates: 28.7-8.8.2025
- Enseignant: Anna-Carolina Haensch
- Enseignant: Frauke Kreuter
- Enseignant: Joseph Sakshaug
Organisation
Veranstaltung | Termin | Ort | Person |
---|---|---|---|
Vorlesung |
Mo, 12.00 - 14.00 |
Schellingstr. 3 (S) Raum S 001 |
Hoffmann |
Vorlesung |
Di, 12.00 - 14.00 |
Geschw.-Scholl-Pl. 1 (A) - A 120 |
Hoffmann |
Übung |
Di, 10.00 - 12.00 |
Geschw.-Scholl-Pl. 1 (A) - A 120 | Gruber/Sauer |
Übung |
Do, 14.00 - 16.00 |
Geschw.-Scholl-Pl. 1 (A) - A 120 |
Gruber/Sauer |
Tutorium |
Mo, 12.00 - 14.00 |
Schellingstr. 3 (S) Raum S 001 | Gorich |
Einschreibeschlüssel
LiMo_s25
- Enseignant: Eugen Gorich
- Enseignant: Cornelia Gruber
- Enseignant: Sabine Hoffmann
- Enseignant: Christina Sauer
Termine:
Termin | Ort | Person | Beginn | |
---|---|---|---|---|
Vorlesung | Di, 08:30-10:00 Mi, 10:15-11:45 |
Schellingstr. 3 (S) - S 001 Schellingstr. 3 (S) - S 006 |
Thomas Nagler | 06.05.2025 |
Übungsgruppe 1 | Mo, 16:15-17:45 | Geschw.-Scholl-Pl. 1 (B) - B 006 | Jana Gauß | 12.05.2025 |
Übungsgruppe 2 | Do, 12:15-13:45 | Geschw.-Scholl-Pl. 1 (B) - B 006 | Jana Gauß | 08.05.2025 |
Tutorium | Fr, 12:15-13:45 | Geschw.-Scholl-Pl. 1 (D) - D 209 | Eugen Gorich | TBA |
Einschreibeschlüssel
- Der Einschreibeschlüssel lautet: "linalg2025"
- Enseignant: Jana Gauß
- Enseignant: Eugen Gorich
- Enseignant: Thomas Nagler
Schedule:
Person | Beginning | |||
---|---|---|---|---|
Lecture/Exercise | Tuesday, 10:15-11:45 | A 213 | Hoffmann/Boulesteix | 29.04.25 |
Enrolment key
- The enrolment key is: "Pitfalls25"
- Enseignant: Anne-Laure Boulesteix
- Enseignant: Sabine Hoffmann
- Enseignant: Christina Sauer
Schedule:
Person | Beginning | |||
---|---|---|---|---|
Lecture | Tuesday, 14:15-16:45 | F 007 | Hoffmann/Sauer/Boulesteix | 29.04.25 |
Exercise session | Friday, 14:15-15:45 | F 007 | Sauer | 16.05.25 |
Enrolment key
- The enrolment key is: "DAS25"
- Enseignant: Anne-Laure Boulesteix
- Enseignant: Sabine Hoffmann
- Enseignant: Christina Sauer
Dates:
Date | Place | Person | Start | |
---|---|---|---|---|
Lecture | Tue, 12:15-13:45 | Geschw.-Scholl-Pl. 1 (M) / M 101 | Nagler | 06.05.23 |
Lecture/Exercise | Wed, 12:15-13:45 | Oettingenstr. 67 / 057 | Nagler/Palm | 07.05.23 |
Enrolment
- The enrolment key is: "rademacher"
- Enseignant: Thomas Nagler
- Enseignant: Nicolai Palm
- Bachelor Statistik und Data Science: WP7 Ausgewählte Gebiete der statistischen Modellierung
- Nebenfach Statistik und Data Science (60 ECTS): WP6 Einführung in die Bayes-Statistik
- Nebenfach Statistik und Data Science (30 ECTS): WP8 Einführung in die Bayes-Statistik
- Bachelor Informatik mit integriertem Anwendungsfach Statistik: WP40 Einführung in die Bayes-Statistik
- Bachelor Wirtschaftsmathematik: P17 Ausgewählte Gebiete der angewandten Statistik
- Andere Studiengänge auf Anfrage
- Enseignant: Martje Rave
- Enseignant: Volker Schmid
Kick-off date: TBA (mid-May)
Enrollment Key: gradjitvmap
Supervisors:
- Mina Rezaei
- David Rügamer
- Emanuel Sommer
- TBD
- Enseignant: Maarten Jung
- Enseignant: Julius Kobialka
- Enseignant: Mina Rezaei
- Enseignant: David Rügamer
- Enseignant: David Rundel
- Enseignant: Emanuel Sommer
Welcome to this introductory course on causality! This is where we gather all the material and organize the in-person sessions.
Dates [Summer Term 2025]
Lecture: Monday, 12h15 - 13h45
Lecture/Exercise (alternating): Tuesday, 08h30 - 10h00
If self-enrollment in this moodle course does not work automatically, you need to register via the backdoor ;)
--> Enrollment key: backdoor
- Enseignant: Michael Schomaker
- Enseignant: Christoph Wiederkehr
Tuesday, April 29, 10 am
Seminar Room #144, Ludwigstr. 33
Final presentations:
Friday, July 18, 8 am - 6 pm
Old Library #245, Ludwigstr. 33
- Enseignant: Tomasz Olma
- Enseignant: Vincent Starck
- Enseignant: Anna-Carolina Haensch
- Enseignant: Olga Kononykhina
- Enseignant: Leah von der Heyde
- Enseignant: Georg Schollmeyer
password: savage
Times and Dates: Monday, 2.15 to 3.45 pm in A119, and Tuesday, 5.30 to 7.00 pm in F007. The lectures start on 29.04.
Contents: Decision theory deals with rational decisions under uncertainty. It has high interdisciplinary importance, for example, in the analysis and support of decisions in business administration or finance (e.g. investment strategies), economics or sociology (rational choice theory), medicine (e.g. expert systems) or engineering (e.g. autonomous control). Moreover, statistical decision theory can be seen as a formal framework for choosing analysis methods (optimal tests or estimators, best classification algorithms, etc.). This general view, understanding statistics and machine learning as special cases of decision theory, plays a fundamental role in the critical analysis and problem-adequate generalization of any data-based learning procedure.
The course first discusses the general structure of decision problems, including fundamental decision principles. Then it analyzes and characterizes the Bayes and minimax criteria as extreme poles to deal with (state) uncertainty and develops modern alternatives in the context of complex uncertainty (ambiguity).
Curricular Embedding: 6 ECTS, Master's programme in Statistics and Data Science: "Narrow electice (2 out of 3)" in the Methodology and Modelling track, "wider elective" in the Social Statistics and Data Science and the Econometrics tracks, "general elective" for all tracks; elective in the Master's programme Versicherungs- und Finanzmathematik; options for other programmes upon request.
- Enseignant: Ivan Melev
Schedule:
Time | Lecturer | Begin | |
---|---|---|---|
Lecture |
Tuesday, 12:15 - 13:45 |
Prof. Dr. Heumann |
29.04.2025 |
Exercise course (Group 1) |
Wednesday, 08:15 - 09:45 | Sapargali, Garces Arias |
30.04.2025 |
Exercise course (Group 2) |
Wednesday, 14:15 - 15:45 |
Sapargali, Garces Arias |
30.04.2025 |
Tutorial | Thursday, 08:15 - 09:45 |
Jai |
24.04.2025 |
Lecture |
Friday, 10:15 - 11:45 |
Prof. Dr. Heumann |
25.04.2025 start of lecture |
Enrollment Key
- The enrollment key is "stat_inf_s25"
- Enseignant: Esteban Garces Arias
- Enseignant: Christian Heumann
- Enseignant: Jai Lunkad
- Enseignant: Nurzhan Sapargali
- Enseignant: Göran Kauermann
- Enseignant: Ivan Melev
- Enseignant: Michael Windmann
Schedule
Type | Date | Location | Start |
---|---|---|---|
Lecture | Mondays 12:15-14:00 | Geschw.-Scholl-Pl. 1 (A) / A 022 | 23.04.2025 |
Lecture/Tutorial | Fridays 12:15-14:00 | 25.07.2025 |
Enrolment Key: ectheory25
- Enseignant: Mauricio Olivares Gonzalez
In the seminar "Foundation Models in e-Health" we will delve into the rapidly evolving landscape of AI, focusing on the practical use and implementation of foundation models for e-health applications. These models, which serve as the backbone for a wide range of AI applications, offer powerful tools for tackling complex problems across various domains. Throughout the seminar, we'll explore key techniques for fine-tuning and adapting these models to specific e-health tasks, ensuring participants gain a solid understanding of how to leverage their capabilities effectively.
The seminar is designed to bridge the gap between theory and practice, providing master’s students with hands-on experience and actionable insights. We will examine the real-world challenges and do case studies in medical domain.
Recommended prerequisites: Deep learning; Python, PyTorch, TensorFlow, We would also hold the seminar in English and also allow students from other courses (especially DS students)
Organization:
- Enroll key: s25_seminar_ehealth
- First meeting: Friday, 11:00-12:30 am, April. 18th at Ludwig str. 33, Seminar Room (144)
- Weekly meeting: Friday, 11:00-12:30 am
[Zoom](link)
- A first meeting will occur at the beginning of the semester (scheduled in agreement with the participants), during which the seminar topics will be briefly introduced and assigned to the participants.
- Seminar type: Block-type and in-person
- Language: The seminar will be held in English
- Enseignant: Yawei Li
- Enseignant: Mina Rezaei
Einschreibeschlüssel: StoSta25
Termine
Termine
- Enseignant: Jan Anders
- Enseignant: Nurzhan Sapargali
- Enseignant: Fabian Scheipl
- Enseignant: Christoph Wiederkehr
Enrolment key: RegCor25
Format: In-person lectures and lab sessions
Dates & Rooms:
Wed 10-12 @ Main Building A 017
Thu 14-16 @ Schellingstr. 3 (S) - S 001
Grading: Oral exams at the end of the semester.
- Enseignant: Fabian Scheipl
Key for enrolling: adsssds
- Enseignant: Unai Fischer Abaigar
- Enseignant: Anna-Carolina Haensch
- Enseignant: Christoph Kern
Der Schlüssel zum Einschreiben ist Stat2NFSoz25.
- Enseignant: Christoph Kern
- Enseignant: Leonhard Kestel
- Enseignant: Clara Strasser Ceballos
Advanced Statistical Programming using R
The enrolment key / password for the course is: StatProgSS25.
If you have questions please contact statprog@stat.uni-muenchen.de.
The course will be taught in English, more information can be found in the course itself.
- Enseignant: Jacob Beck
- Enseignant: Lisa Bondo Andersen
- Enseignant: Markus Herklotz
- Enseignant: Jan Simson
- Enseignant: Anna Steinberg
Enrollment Key (Einschreibeschlüssel): UTSA_2025
- Enseignant: Tiphaine Wibault
Format
Lecture
- Time: Monday 08:30 - 10:00 am.
- Location: F 007.
Exercise
- Time: Friday 10:15 - 11:45 am.
- Location: F 007.
Enrollment key
- Please only enroll if you want to ACTIVELY participate, you will be automatically unenrolled after 1 month of inactivity. The enrollment key is: IML-2025_advml
Contents
This course is an introduction into concepts and methods of machine learning interpretability and their implementation with R or python. It counts as the module "Advanced Machine Learning". The course focuses on model-agnostic methods for tabular data and in parts is close to the course on our website. Course topics include a general introduction into questions and concepts of interpretable ML, inherently interpretable ML models, global and local feature effect methods, functional decompositions, Shapley values, feature importance methods, regional feature effect methods and local as well as counterfactual explanations.- Enseignant: Coco Bögel
- Enseignant: Giuseppe Casalicchio
Format
Class
- Time: Thursday 12:15 - 13:45 h.
- Location: B 106.
Exercise
- Time: Wednesday 10:15 - 11:45 h.
- Location: D 209.
Enrollment key
- The enrollment key is: "I2ML".
- Enseignant: Ludwig Bothmann
- Enseignant: Philip Boustani
- Enseignant: Simon Rittel
- Grundlagen der Programmierung in Python (Datentypen, Datenstrukturen, Kontrollstrukturen, Fehlerbehandlung, Funktionen, OOP)
- Datenverarbeitung in Python (Numpy, Pandas)
- Visualisierung in Python (Matplotlib, Seaborn, Pandas)
- Machine Learning und Statistik in Python (scikit-learn, statsmodels, scipy)
- Fortgeschrittene Programmiertechniken (PEP8, unit tests, Cython, Joblib)
Einschreibeschlüssel: Python
Vorlesung: Dienstags 9:30 (ab Dienstag, dem 27.04)
- Enseignant: Andreas Bender
- Enseignant: Matthias Feurer
Short Description: You will learn how to tackle practical challenges in ML using R. Topics include an introduction to an ML toolbox in R, (advanced) tuning techniques, custom ML pipelines, classifier calibration, handling class imbalances, ML benchmark experiments with hypothesis testing, ML model ensembling/stacking, and ML interpretability. Sessions consist of brief methodological overviews followed by hands-on exercises, requiring laptops for full engagement.
Prerequisite: To participate in this lecture, you must have completed and passed the courses:
- Introduction to Machine Learning (I2ML)
- Programming with Statistical Software (R), ideally with a good grade as good knowledge of R is required
Kick-off: Wednesday, April, 23rd from 09:00 - 12:00 (s.t.) (check Moodle for up-to-date information)
Enrolment key: appml25
- Enseignant: Giuseppe Casalicchio
- Enseignant: Fiona Ewald
- Enseignant: Matthias Feurer
Teilnehmende dieses BA-Seminars werden basierend auf der offiziellen Seminareinteilung von Dr. Schollmeyer dem Moodle-Kurs hinzugefügt.
Wichtige Informationen zur Orientierung sind in diesem Dokument zusammengefasst.
Bei Fragen wenden Sie sich bitte an ludwig.bothmann@lmu.de
Kick-off: 10.04.25, 12.15-13.45 Uhr, Alte Bibliothek, Ludwigstr. 33, Raum 245
- Enseignant: Ludwig Bothmann
- Enseignant: Giuseppe Casalicchio
Enrolment key: Infstat2_S25
- Enseignant: Jan Anders
- Enseignant: Sergio Buttazzo
- Enseignant: Eugen Gorich
- Enseignant: Göran Kauermann
Tuesday 16-18 Geschw.-Scholl-Platz 1 (A) - M 110
Thursday 14-16 Geschw.-Scholl-Platz 1 (A) - M 105
Enrolment key:
CIN25
- Enseignant: Vincent Starck
- Enseignant: Jörg Drechsler
- Enseignant: Jörg Drechsler
- Enseignant: Anna-Carolina Haensch
The course is an introduction to widely used algorithms and data structures, focusing on those most useful in data science. Students are introduced to how data is represented in memory, from individual characters and numbers to complex structures representing hierarchies and graphs. The standard analytical tools used to determine an algorithm's efficiency and the accuracy of its results are also covered.
This course can be taken as an elective module "WP1" and is recommended for the 6th semester (4th semester if you are motivated) in the Bachelor's program. Note that this course can not be credited towards the Master's degree. It targets students with an interest in programming, computer science, and algorithmic thinking. If you liked ProgR, we think you will also like this course.
Schedule:
Day / Time | Room | Lecturer | Dates | ||
---|---|---|---|---|---|
Lecture | Tuesday 14:00--16:00 c.t. |
| Bischl | 2025-04-29 -- 2025-07-22 | |
Lct. / Ex. | Wednesday 12:00--14:00 c.t. | Schellingstr. 3 (S) - S 005 | Binder | 2025-04-23 -- 2025-07-23 |
Credits: 6 ECTS
- The enrolment key is: AL_GODS_25
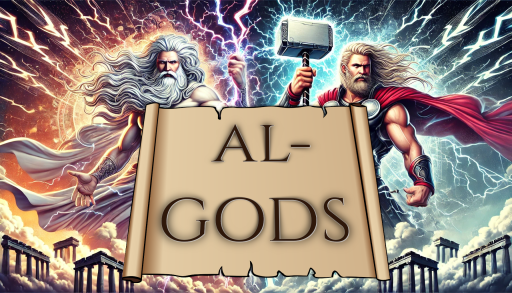
- Enseignant: Martin Binder
- Enseignant: Bernd Bischl
- Enseignant: Tobias Pielok
The course Analysis of high-dimensional biological data (formerly Statistische Methoden in Genomik und Proteomik / Statistical methods for biological high-throughput data) will cover important statistical methods and concepts for the analysis of high-dimensional biological high-throughput data. We will focus on bulk RNA-Seq, single-cell RNA-Seq, proteomic, metabolic, and especially microbiome data such as 16S rRNA and other amplicon data.
Schedule:
Date and Time | Place | Person | Beginn | |
---|---|---|---|---|
Lecture | Wed, 14:00 - 16:00 | Prof.-Huber-Pl. 2 (V) - LEHRTURM-VU107 |
C.L Müller | 23.04.2025 |
Exercises | Mo, 10:00 - 12:00 (bi-weekly) |
Geschw.-Scholl-Pl. 1 (M) - M 203 | Olayo-Alarcon | 05.05.2025 |
Enrollment key:
HighDimBio25
- Enseignant: Christian Müller
- Enseignant: Roberto Olayo Alarcon
Description:
In the rapidly evolving field of biomedical research, generating large-scale high-dimensional datasets has become commonplace. This, in turn, has motivated the development and application of new statistical and machine-learning methods to gain valuable insights from such data. In this seminar, students will learn about the most recent advances in data science and statistics aimed at analyzing large-scale biomedical data. The topics covered include (but are not limited to): Compositional data analysis, Variable selection, Network inference and analysis, Self-supervised deep learning, Selective inference, High-dimensional clustering, etc.
Organization:
- A first meeting will occur at the beginning of the semester (scheduled in agreement with the participants), where the seminar topics are briefly introduced and assigned to the participants.
- Seminar type: Block-type and in-person
- Language: The seminar will be held in English
- Enrollment to the seminar will be done by the lecturer after seminar assignment
- Enseignant: Roberto Olayo Alarcon
Date & Time | Place | Person | |
---|---|---|---|
Lecture | Mo., 10:15 - 11:45 | S 001 | Rügamer |
Lecture | Di., 10:15 - 11:45 | S 006 | Rügamer |
Exercise | Th., 12:15 - 13:45 | E 004 | Kobialka/Schulte |
Tutorial | Fr., 08:15 - 09:45 | B 106 | Runnwerth |
Lecture: David Rügamer
Exercise: Julius Kobialka, Rickmer Schulte
Tutorial: Kilian Runnwerth
Enrollment Key: StatMod101
- Note: Due to labor day (public holiday),
- the first lab session will be on May 8
- the introductory lectures will be available only as videos (see table of content)
- Enseignant: Julius Kobialka
- Enseignant: David Rügamer
- Enseignant: Kilian Runnwerth
- Enseignant: Rickmer Schulte
Instructors:
- David Rügamer
- Mina Rezaei
- Emanuel Sommer
Time:
- Lecture: Every Monday 16:00 - 18:00 (c.t.) -> Room: Geschw.-Scholl-Pl. 1 (M) / M 105
- Labs: Every Thursday 10:00 - 12:00 (c.t.) -> Room: Geschw.-Scholl-Pl. 1 (M) - M 014
Start:
- Note: Due to labor day (public holiday),
- the first lab session will be on May 8
- the first lecture session will be on May 5
Enrollment Key:
- Singularity
- Enseignant: Mina Rezaei
- Enseignant: David Rügamer
- Enseignant: Emanuel Sommer
Enrolment Key: sl_summer25
- Enseignant: Ludwig Bothmann
- Enseignant: Xiao-Yin To
- Enseignant: Anna-Maria Brandtner
- Enseignant: Anna-Carolina Haensch
- Enseignant: Sonja Kellner
- Enseignant: Iris Trautmann
- Enseignant: Johannes Piller
- Enseignant: Simon Wiegrebe
The field of Automated Machine Learning is thriving, and researchers make tremendous progress in democratizing machine learning. An important new model class is the class of foundation models, and we will discuss how AutoML techniques can be applied to such models, and what these models offer for AutoML. Of course, the seminar will also cover other recent advances, such as human-in-the-loop AutoML.
More concretely, we will discuss topics along the lines of:
- How can we use AutoML to tune the hyperparameters of foundation models?
- Can we use foundation models in AutoML?
- How can we incorporate human experts in the AutoML process?
The following papers are samples of the seminar content:
- https://www.nature.com/articles/s41586-024-08328-6
- https://proceedings.neurips.cc/paper_files/paper/2022/hash/cf6501108fced72ee5c47e2151c4e153-Abstract-Conference.html
- https://openreview.net/forum?id=VTTL6x0z6V
- https://arxiv.org/abs/2412.07820
Time: Tuesday, 4PM
Location: Seminar room LU33
Kickoff: April 29th
- Enseignant: Matthias Feurer
Description
This course provides a rigorous and theoretical exploration of dynamical systems in deep learning, building on courses on deep learning, probability theory, and optimization. The focus is on mathematical foundations and advanced concepts such as ordinary and stochastic differential equations, sampling-based inference, transport methods, and diffusion processes. Key topics include Neural ODEs, Langevin dynamics, optimal transport, and score-based generative models. Additionally, the seminar is expected to cover more recent developments like Schrödinger bridges, Hamiltonian Monte Carlo, and physics-informed neural networks.
Participation
Only via invite after applying in the seminar registration phase
Recommended prerequisite
- Deep Learning Course
- (Optimization)
- Solid Background in Probability Theory
- Enseignant: Julius Kobialka
- Enseignant: Yawei Li
- Enseignant: Tobias Pielok
- Enseignant: David Rügamer
- Enseignant: Rickmer Schulte
- Enseignant: Emanuel Sommer
Statistik II für Statistiker: Wahrscheinlichkeitstheoretische Grundlagen der Statistik
Termin | Hörsaal | Dozent | |
---|---|---|---|
Vorlesung | Mo 10-12 | Volker Schmid | |
Do 9–12 | |||
Übung Gruppe 1 | Mo 14–16 | N.N. | |
Übung Gruppe 2 | Mi 8–10 | ||
Tutorium | Di 18-20 | Michael Kobl |
Einschreibeschlüssel: Bernoulli
- Enseignant: Michael Kobl
- Enseignant: Nicolai Palm
- Enseignant: Patrick Schenk
- Enseignant: Daniel Schlichting
- Enseignant: Volker Schmid
Person: Dr. Cornelia Oberhauser
SAS course as a 5-day block course during the semester break
Dates:
Day |
Time |
Room |
|
---|---|---|---|
Mon 17.03.2025 |
Lecture | 9:15 - approx. 12:15 |
online via Zoom |
Exercise | 13:15 - 17:00 |
online via Zoom |
|
Tue 18.03.2025 |
Lecture |
9:15 - approx. 12:15 | online via Zoom |
Exercise | 13:15 - 17:00 | online via Zoom | |
Thu 20.03.2025 |
Lecture |
9:15 - approx. 12:15 | online via Zoom |
Exercise | 13:15 - 17:00 | online via Zoom | |
Mon 24.03.2025 |
Lecture |
9:15 - approx. 12:15 | online via Zoom |
Exercise |
13:15 - 17:00 | online via Zoom | |
Tue 25.03.2025 |
Lecture |
9:15 - approx. 12:15 | online via Zoom |
Exercise |
13:15 - 17:00 | online via Zoom |
Enrolment key
- The enrolment key is: ‘saskurs2025’
Guest key
- The guest key is: ‘saskurs2025’
- Enseignant: Cornelia Oberhauser
Termine
- Wiederholungsübung: tbd
Einschreibeschlüssel
- Der Einschreibeschlüssel lautet "stats25wdh".
- Enseignant: Helen Alber
- Enseignant: Matthias Aßenmacher
- Enseignant: Michael Sawitzki
Termine
- Vorlesung: Dienstag, 16 - 18 c.t.
- Übungen (Statistik II):
Mittwoch, 12 - 14 c.t. & 14 - 16 c.t.
Donnerstag, 18 - 20 s.t.
Freitag, 10 - 12 c.t. - Übung (Statistik I):
Montag, 10 - 12 c.t.
Einschreibeschlüssel
- Der Einschreibeschlüssel lautet "2025wiwistat".
- Enseignant: Matthias Aßenmacher
- Enseignant: Benjamin Dornow
- Enseignant: Polina Gordienko
- Enseignant: Vanessa Kleisch
- Enseignant: Antonio Melieni
- Enseignant: Teresa Rupprecht