Der Einschreibeschlüssel ist: problemlabs
- Trainer/in: Kajetan Söhnen
- Trainer/in: Dirk Deckert
- Trainer/in: Franz Merkl
- Trainer/in: Alejandro Caicedo Serrano
- Trainer/in: Robert Helling
- Trainer/in: Sabine Jansen
Große Persönlichkeiten der Mathematik
Doch was zeichnet einen großen Mathematiker (oder eine große Mathematikerin) aus? Ist es
RAFFINESSE:
Elegante Beweise, die man erst beim vierten Lesen versteht, sind schließlich die Schönsten.
ÄSTHETIK:
Mathematik ist allerdings zum Glück nicht ganz so (mandel)brotlos wie die bildenden Künste.
TIEFGANG:
Eigentlich zieht einen die Mathematik nur so nach unten, damit man sie in aller ihrer Schöne wahrnehmen kann.
SELBSTÄNDIGKEIT:
Als großer Mathematiker muss man selbstständig sein und am besten schreibt man seine Vermutungen nur an den Rand seines Notizheftes und redet mit niemanden darüber.
ENTDECKERGEIST:
Ständig auf der Suche nach der nächsten Überdeckung und Metrik zur Vermessung der Welt. Mathematiker sind echte Pioniere.
LOGISCHES DENKEN:
Große Mathematiker sind Meister des logischen Denkens und verstehen spätestens jetzt, dass diese Liste nicht ganz Cauchy ist und nur als Schlüssel dient.
Und wie wird man zum großen Mathematiker? Nun ALLER ANFANG ist schwer, wird komplett großgeschrieben und ist der Einschreibeschlüssel dieses Moodlekurses.
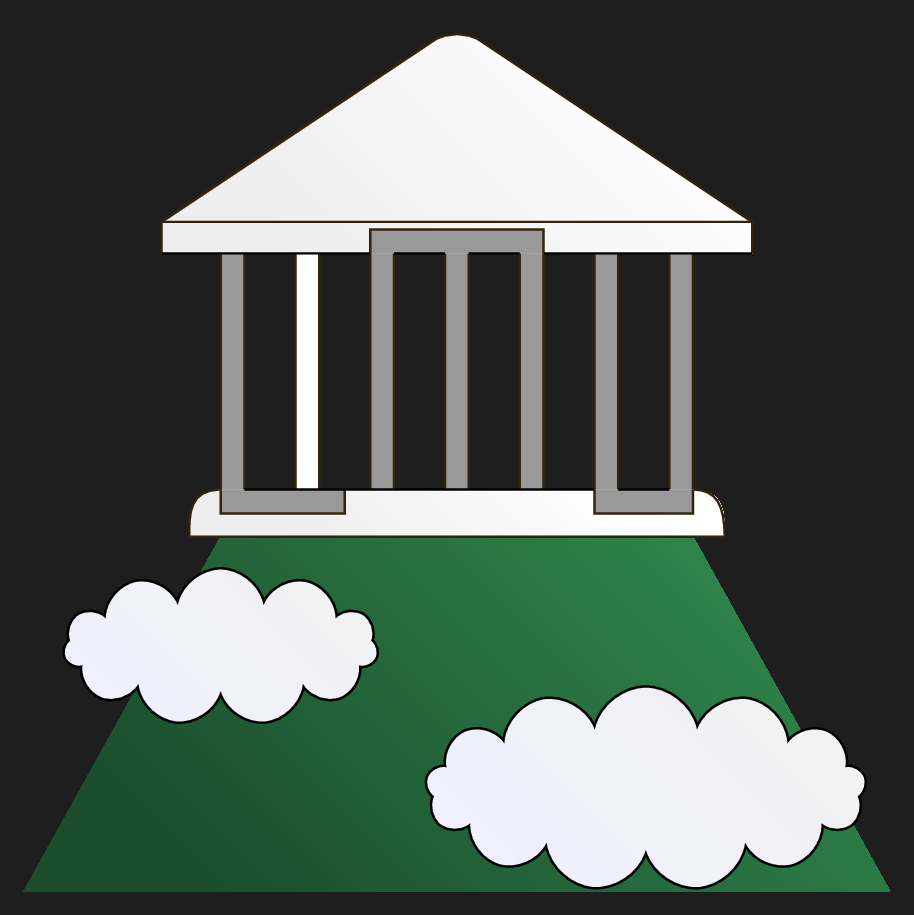
- Gesandter des oLyMpUs: Sebastian Hensel
- Gesandter des oLyMpUs: Kajetan Söhnen
- Trainer/in: Jinyeop Lee
- Trainer/in: Thành Nam Phan
- Trainer/in: Arnaud Triay-Alcouffe
- Trainer/in: Simon Gritschacher
- Trainer/in: Sebastian Hensel
- Trainer/in: Simon Gritschacher
- Trainer/in: Dieter Kotschick
- Trainer/in: Arnaud Triay-Alcouffe
- Trainer/in: Sebastian Hensel
- Trainer/in: Kajetan Söhnen
- Trainer/in: Alessandro Sgarabottolo
- Trainer/in: Peter Müller
- Trainer/in: Sara Terveer
- Trainer/in: Ralf Gerkmann
- Trainer/in: Ralf Gerkmann
- Trainer/in: Fabian Porsche
- Trainer/in: Julian Becker
- Trainer/in: Ralf Gerkmann
- Trainer/in: Andreas Rosenschon
- Trainer/in: Simon Seidenschwarz
- Trainer/in: Long Meng
- Trainer/in: Larry Read
- Trainer/in: Arnaud Triay-Alcouffe
- Trainer/in: Werner Bley
- Trainer/in: Eva Brenner
Course description:
In many applications, we are interested in studying an object of interest x. However, its properties are often not directly measurable. Instead, we only observe y = F(x), where the function F is known. To infer about x from the observation y, we seek an (approximate) inverse mapping x ≈ F-1(y). This process is known as solving the inverse problem.
In this course, we will cover three topics. The first is classical regularization theory, which highlights the difficulties of solving inverse problems related to noisy data. In the second part, we move to the variational formulation of inverse problems and discuss optimization strategies. Finally, the last part focuses on explainable data-driven and network-based approaches for inverse problems.
Planning:
Credits: 9 ECTS
Modules:
MSc Math: WP35
MSc FiMa: WP12 or WP22
MSc Stat&DS: WP 31 or WP 52
MSc DS: WP 3
Enrolment Key: N*OLC,1s+EGI96rqDea-
Target Audience: MSc Math students
Lectures: Tuesdays 16:00-18:00 in room B006 and Fridays 10:00-12:00 in room A027 in Theresienstr. 39
Tutorials: Mondays 12:00-14:00 or 16:00-18:00 in room 504 in Akademiestr. 7 (5th floor).
- Trainer/in: Stefan Kolek Martinez de Azagra
- Trainer/in: Melnyk Oleh
Wir werden typische Aufgabenstellungen beim Staatsexamen in Analysis behandeln,
Lösungsmethoden besprechen und evtl. noch etwas zugrunde liegende Theorie
wiederholen. Wir beginnen mit dem Themenbereich Funktionentheorie und
arbeiten uns dann zu den Gewöhnlichen Differentialgleichungen durch.
Wir starten am Mittwoch 23.4.2025 und Donnerstag 24.4.2025 mit
ganz "normalem" Aufgabenrechnen.
Einschreibeschlüssel: stexana
- Trainer/in: Heribert Zenk
Dienstag 10-12 Uhr in B041 und Mittwoch 8.30-10 Uhr in B041;
Übung Mittwoch 14-16 Uhr in B046
Wir starten am ersten Vorlesungstag, Mittwoch 23.4.2025 um 8.30 Uhr..
Einschreibeschlüssel: fock
- Trainer/in: Heribert Zenk
Dienstag 8-10 Uhr und Donnerstag 12-14 Uhr in C123;
(Zentral)übung Mittwoch 16-18 Uhr in C123
Beginn: 23.4.2025 -- mit einer Vorlesung statt Übung
Die Vorlesung ist die zweite eines dreisemestrigen Kurses in Mathematik
für das Physikstudium.
Einschreiben mit Schlüssel m2p
- Trainer/in: Matthias Bauernfeind
- Trainer/in: Cassian Hallinger
- Trainer/in: Kajetan Söhnen
- Trainer/in: Alois Wohlschlager
- Trainer/in: Heribert Zenk
- Trainer/in: Christian Fries
- Trainer/in: Alessandro Sgarabottolo
- Trainer/in: Niklas Weber
- Trainer/in: Ulrich Terstiege
- Trainer/in: Ulrich Terstiege
- Trainer/in: Tizian Wenzel
- Trainer/in: Julius Hallmann
- Trainer/in: Konstantinos Panagiotou
- Trainer/in: Tamas Makai
- Trainer/in: Konstantinos Panagiotou
Einschreibeschlüssel: Querschnitt25S
- Trainer/in: Leon Bollmann
- Trainer/in: Erwin Schörner
- Trainer/in: Jago Silberbauer
- Trainer/in: Leonard Wetzel
Einschreibeschlüssel: Grundlagen2S25
- Trainer/in: Erwin Schörner
- Trainer/in: Francois Visconti
Einschreibeschlüssel: Diff2SS25
- Trainer/in: Daniel Rost
- Trainer/in: Tizian Wenzel
Einschreibeschlüssel: LA2SS25
- Trainer/in: Laura Paul
- Trainer/in: Daniel Rost
Einschreibeschlüssel: ExamUF25S
- Trainer/in: Daniel Rost
- Trainer/in: Erwin Schörner
- Trainer/in: Thilo Meyer-Brandis
- Trainer/in: Annika Steibel
- Trainer/in: Thomas Sørensen
- Trainer/in: Thomas Vogel
- Trainer/in: Wiebke Bartolomaeus
- Trainer/in: Paula Reichert-Schürmer
- Trainer/in: Christian Lange
- Trainer/in: Bernhard Leeb
Enrolment Key: Num2S25
- Trainer/in: Giao Duong
- Trainer/in: Peter Philip
Einschreibeschlüssel: An2S25
- Trainer/in: Daniel Misselbeck-Wessel
- Trainer/in: Peter Philip
- Trainer/in: Paula Reichert-Schürmer
Einschreibeschlüssel: AxM25
- Trainer/in: Peter Philip
- Trainer/in: Lukas Böke
- Trainer/in: Thomas Vogel
Kursbeschreibung
In dieser Vorlesung werden bedingte Erwartungswerte mithilfe des Satzes von Radon-Nikodým eingeführt und ihre wesentlichen Eigenschaften hergeleitet. Zudem werden die Grundlagen der Theorie stochastischer Prozesse in diskreter Zeit, mit einem starken Fokus auf Martingale, behandelt. Dazu werden wir uns mit relevanten Methoden aus der Maß- und Integrationstheorie befassen und insbesondere das starke Gesetz der großen Zahlen, das Null-Eins-Gesetz von Kolmogorov und die Lemmata von Borel-Cantelli beweisen.
Vorgesehen für Studierende folgender Studiengänge
- Mathematik im Bachelor und Master, Wirtschaftsmathematik im Bachelor, Theoretische und Mathematische Physik im Master
Voraussetzungen
- Kenntnisse der Stochastik und der Maß- und Integrationstheorie
Einschreibeschlüssel
- W-Theorie
- Trainer/in: Alexander Kalinin
- Trainer/in: Fabian Nolte
Course Description
In this reading course, we will study stochastic processes in continuous time such as stochastic integrals driven by Brownian motion. By using the approach of Kunita and Watanabe that is based on Riesz’s representation theorem, we will introduce stochastic integrals with respect to square-integrable martingales and analyse their probabilistic properties. In this context, the approximation and simulation of continuous processes will be discussed.
Target Participants
- Master students of Mathematics
Pre-requisites
- Probability theory and measure and integration theory
Registration key
- Integral
- Trainer/in: Alexander Kalinin
Course Description
In this seminar, we will study one-dimensional stochastic Volterra integral equations with a particular focus on fractional kernels. For this purpose, fundamental concepts in stochastic analysis, such as semimartingales, stochastic integrals and fractional Brownian motion, will be discussed. The aim of this course is to derive unique strong solutions under Lipschitz conditions on the drift and diffusion coefficients of the stochastic Volterra equation.
Target Participants
- Master students of Mathematics and Financial and Insurance Mathematics
Pre-requisites
- Probability theory and measure and integration theory
Registration key
- Volterra
- Trainer/in: Alexander Kalinin
Die Vorlesung Funktionentheorie (engl. complex analysis) behandelt Funktionen einer komplexen Variable. Zentrale Themen sind holomorphe und meromorphe Funktionen, Laurent-Reihen, der Residuensatz und der cauchysche Integralsatz.
Benutzen Sie zum Einschreiben den passenden Schlüssel je nach Studiengang und Prüfungsordnung:Mathematik PO 2021: Ma2021
Wirtschaftsmathematik PO2021: Wima2021
Lehramt PO 2021: Lehr2021
Lehramt PO 2011: Lehr2011
Alle anderen Studiengänge und Prüfungsordnungen: Laurent
- Trainer/in: Margherita Ferrero
- Trainer/in: Florian Haberberger
- Trainer/in: Christian Hainzl
Blockkurs in der vorlesungsfreien Zeit, in dem die Grundlagen des
Textsatzsystems LaTeX und speziell die Erstellung mathematischer Texte
behandelt werden. Nähere Informationen zu Ablauf und Anmeldung auf der Webseite des Kurses.
- Trainer/in: Mechtild Callies
- Trainer/in: Adalbert Fono
- Trainer/in: Gitta Kutyniok
- Trainer/in: Adalbert Fono
- Trainer/in: Johannes Maly
- Trainer/in: Julia Karczewski
- Trainer/in: Katharina Oberpriller
Enrolment key: aml25
Credits: 6 ECTS (2SWS lecture + 2SWS exercise)
Modules:
- MSc FiMa: WP23 „Advanced Topics in Computer and Data Science B”
- MSc Math: WP42 „Überblick über ein aktuelles Forschungsgebiet B”
Description:
Real-world applications of machine learning require not only a strong theoretical foundation but also a solid knowledge of the methodologies, tools, and heuristics essential for implementing machine learning algorithms. However, the practical aspects of machine learning are often overlooked in mathematics programs. This course bridges that gap by providing students with hands-on experience in implementation and empirical analysis of machine learning algorithms — critical skills for those pursuing careers in data analysis or machine learning.
Content:
The course covers fundamental topics such as linear regression, gradient descent, regularization techniques, logistic regression, support vector machines (SVMs), and basic neural networks. Additionally, the course will explore advanced optimization methods, multi-class classification strategies, and ensemble learning techniques such as boosting and bagging.A key component of the course is extensive programming in Python, using libraries such as NumPy, Matplotlib, Pandas, and scikit-learn. We will work with real datasets, including MNIST handwritten digits, the Boston Housing dataset, Wine dataset, etc.
- Trainer/in: Mariia Seleznova
Enrolment key: omsose2025
Credits: 9 ECTS
Format: 4 hours lecture, 2 hours exercise
Target audience: MSc FiMa & Math
Time and Location:
Lectures: Tuesday 14-16 (room B133), Thursday 14-16 (room B045)
Exercise Sessions: Monday 12-14 (room B134)
Modules:
MSc FiMa:
- PStO 2021: WP12 Advanced Topics in Mathematics A (9 ECTS)
- PStO 2019: WP13 Advanced Topics in Mathematics A (9 ECTS)
- WP26 Fortgeschrittene Themen aus der Numerischen Mathematik (9 ECTS)
- WP35 Fortgeschrittene Themen aus der KI und Data Science (9 ECTS)
Description:
Optimization is the doctrine for finding the "best" alternative between a set of possible options in terms of a given objective function. The course is devoted to the study of the most widely used optimization methods and their convergence analysis. Throughout the lecture, the students will learn how to select the most suited optimization method for a given problem and to evaluate the expected rate of convergence of the algorithm in that specific scenario. The focus will be continuous optimization, meaning that we will consider problems with continuous variables living in a continuous vector space.
Content:
- basics of optimization;
- first order methods (gradient descent, conjugate gradient, Barzilai-Borwein and Polyak step);
- line search methods (Armijo, nonmonotone);
- second order methods (Newton, Quasi-Newton, Trust-Region);
- constrained optimization (projected gradient method, KKT conditions).
- Trainer/in: Arinze Folarin
- Trainer/in: Leonardo Galli
- Trainer/in: Garam Kim
Password for signing up on the
Webpage!
Signing up closed 07. May 2025.
- Trainer/in: Maximilian Duell
- Trainer/in: Thomas Sørensen